An Insulator Defect Detection Model in Aerial Images Based on Multiscale Feature Pyramid Network
IEEE TRANSACTIONS ON INSTRUMENTATION AND MEASUREMENT(2022)
摘要
The faults caused by insulator defects will seriously threaten the operational safety of the power grid. Therefore, insulator defect detection plays a crucial role in inspecting transmission lines. Compared with traditional methods, the network such as You Only Look Once (YOLO) family based on deep learning has high accuracy and strong robustness in insulator recognition and fault detection. However, the performance of these network is usually affected by the shooting conditions as well as aerial images with diverse types of insulators and complex backgrounds, resulting in poor detection result. In addition, the relatively small insulator fault (bunch-drop) area in aerial images will make detection difficult. To solve these problems, this article proposes an improved insulator defect detection model based on YOLOv4 (ID-YOLO). To create our model, we design a new backbone network structure, cross-stage partial and residual split attention network (CSP-ResNeSt), that can solve the interference problem of complex backgrounds in aerial images to enhance the network's feature extraction capability. In addition, we adopt a new multiscale bidirectional feature pyramid network with simple attention module (Bi-SimAM-FPN), which can address the difficulty of identifying a small scale of insulator defects in an image for more efficient feature fusion. We experimentally demonstrate that the mean average precision (mAP) of the proposed model is 95.63%, which is 3.5% higher than that of the YOLOv4. Most importantly, the detection speed of this model can reach 63 frames/s, which meets the requirements of real-time detection of insulator bunch-drop faults.
更多查看译文
关键词
Insulators, Feature extraction, Detectors, Neurons, Neck, Power transmission lines, Head, Aerial images, fault detection, insulator, multiscale feature pyramid network, You Only Look Once (YOLO)
AI 理解论文
溯源树
样例
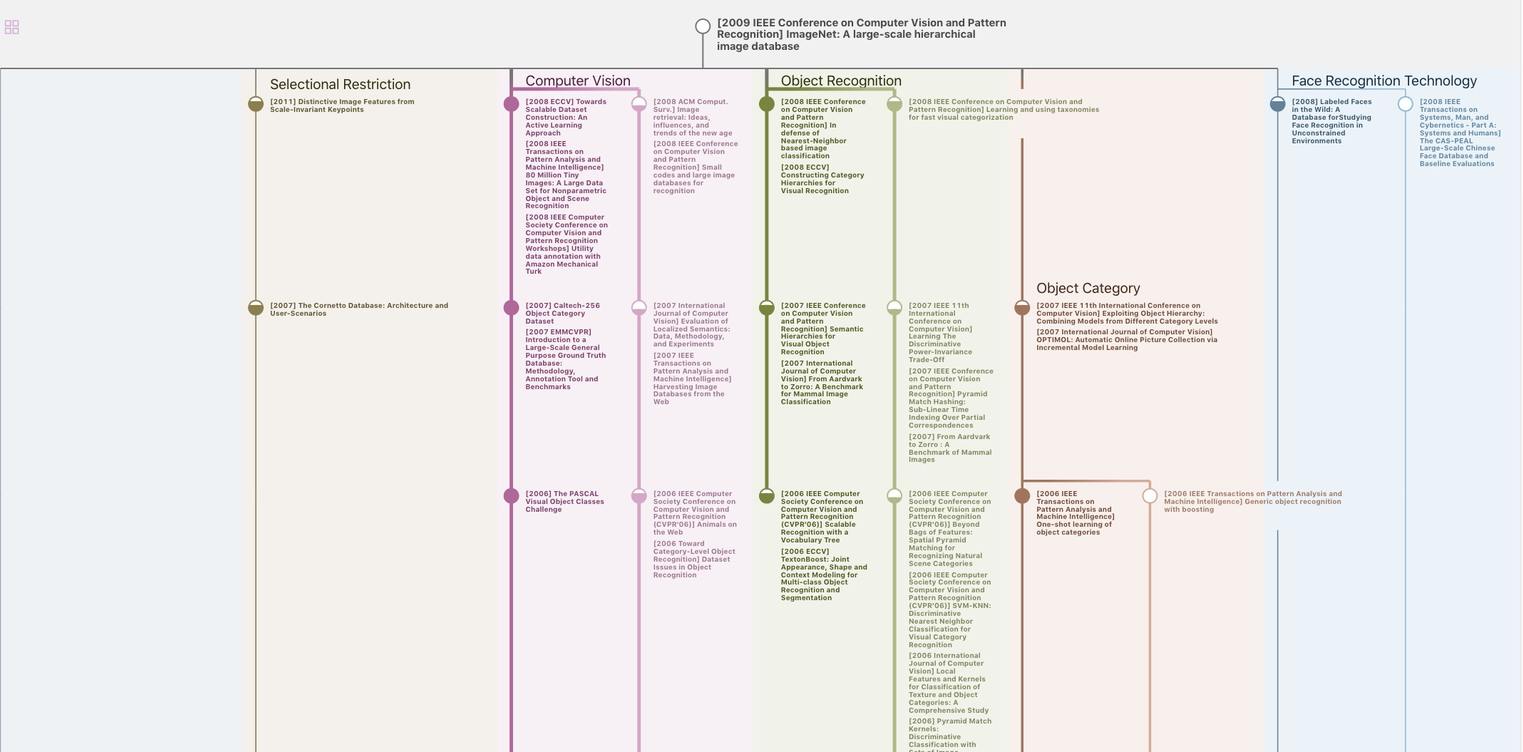
生成溯源树,研究论文发展脉络
Chat Paper
正在生成论文摘要