Archimedes optimizer-based fast and robust fuzzy clustering for noisy image segmentation
The Journal of Supercomputing(2022)
摘要
Fuzzy C-means (FCM) is one of the prominent and effective cluster-based image segmentation techniques exceedingly susceptible to noise and initial cluster centers, thereby effortlessly converging toward local optima. However, FCM consumes exceptionally high computation time due to the repetitive computation of the distance amid cluster centers and pixels. To resolve this apprehension, this paper aims to consider a histogram-based fast fuzzy image clustering (HBFFIC) procedure that primarily tends to carry out morphological reconstruction (MR) operation over the image to assure noise immunity and safeguard details of the imagery. Further, as a replacement for pixels of a summed image, clustering is carried out based on gray-level histogram. This with no qualm radically trims down the computational time as the number of gray levels in an image is normally to a great extent lesser than that of the number of its pixels. Though HBFFIC is a proficient local optimizer however, owing to arbitrary initialization that is carried out in FCM, HBFFIC has the utmost possibility to get effortlessly wedge into local optima. Consequently, this is where the role of nature-inspired optimization algorithms (NIOA) comes into picture. For that reason, this paper successfully makes use of NIOA to prevail over the dilemma using Archimedes optimizer (AO) to discover the most favorable cluster centers. The real-world images particularly synthetic, grayscale, and color pathology images are exercised to perform the experimental study. The experimental study clearly highlights that the proposed hybrid algorithm (HBFFIC-AO) for noisy image segmentation outperforms the other state-of-art algorithms in terms of segmentation accuracy (SA), comparison score (CS), MSE, and PSNR. The visual along with numerical outcomes projected in the experimental study point toward the pre-eminence of the proposed algorithm as compared with the prevailing leading-edge image segmentation algorithms.
更多查看译文
关键词
Fuzzy C-means, Archimedes optimizer, Noisy image segmentation, Nature-inspired optimization algorithms, Swarm intelligence, Histogram-based fast fuzzy image clustering, Fuzzy membership, Membership filtering, Gray-level histogram, Morphological reconstruction, Image segmentation algorithms
AI 理解论文
溯源树
样例
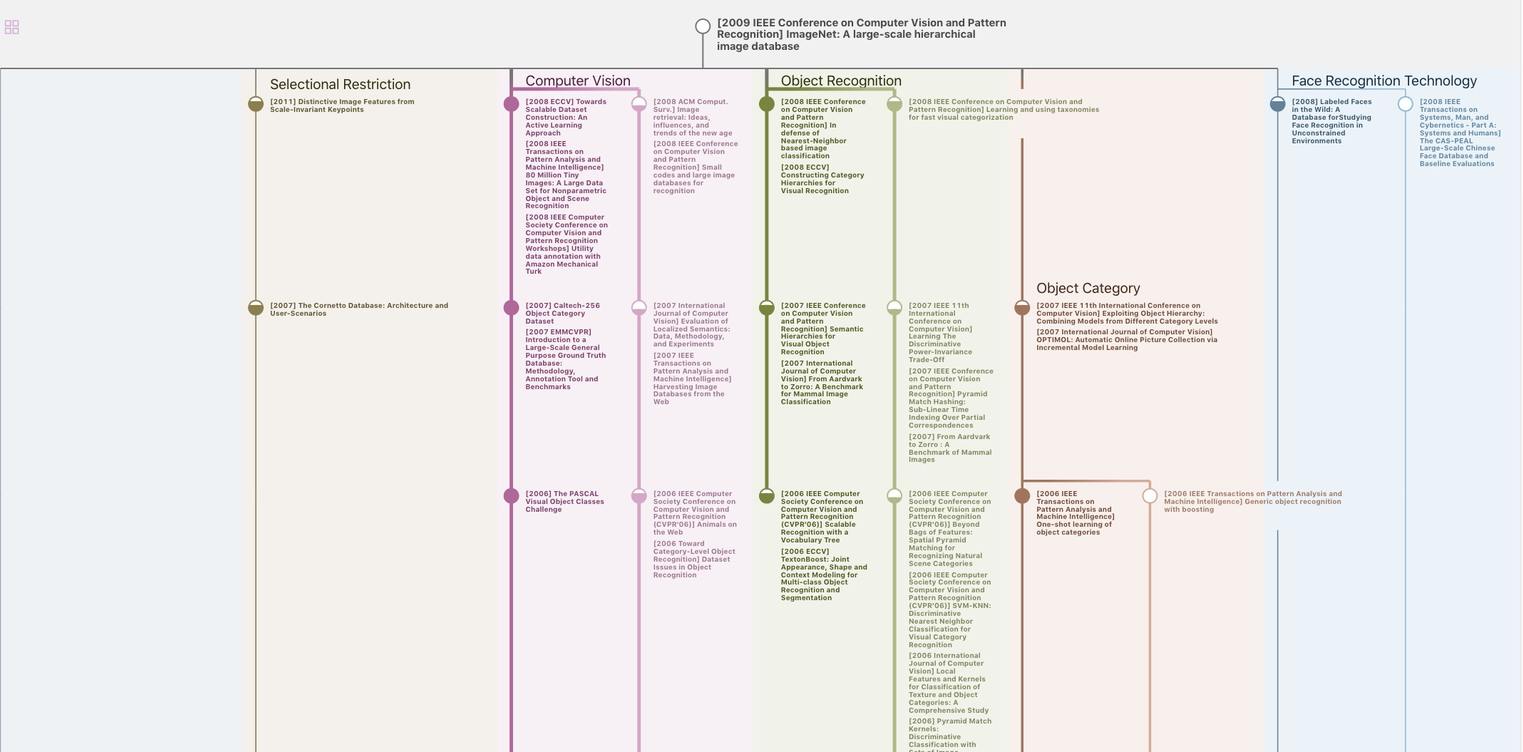
生成溯源树,研究论文发展脉络
Chat Paper
正在生成论文摘要