Neuro-inspired Image Compression Architectures
2022 IEEE International Conference on Imaging Systems and Techniques (IST)(2022)
摘要
The goal of this work is to propose an end-to-end image compression architecture that consists of a well-known transform, like the Discrete Cosine Transform (DCT) or the Discrete Wavelet Transform (DWT), and the recently released neuro-inspired quantization that is based on the Leaky Integrate-and-Fire neuron. This novel quantization mimics the visual system that can be seen as an intelligent biological “machine” that is able to capture and transform the visual stimulus into a sequence of discrete events, called spikes. This spike train is informative enough for the human brain to perceive and take decisions according to the content of the visual stimulus. As a result, it is expected that mimicking the way the neurons generate this code of spikes will be highly beneficial for the image processing community as it describes a completely different, dynamic and energy-efficient manner the visual stimulus can be compressed. In this work, we show that the performance of the neuro-inspired quantizer is highly improved when the input signal is first transformed by DCT or DWT. To be able to measure the performance of the proposed architectures we used the Shannon Entropy to compute the number of bits required to store the compressed images and the Peak Signal to Noise Ratio (PSNR) to calculate the image distortion. Using these metrics, we show that the best rate-distortion trade-of fis given by the architecture that consists of the DCT and the neuro-inspired quantizer.
更多查看译文
关键词
neuro- inspired quantization,DCT,DWT,spikes,Leaky Integrate-and-Fire
AI 理解论文
溯源树
样例
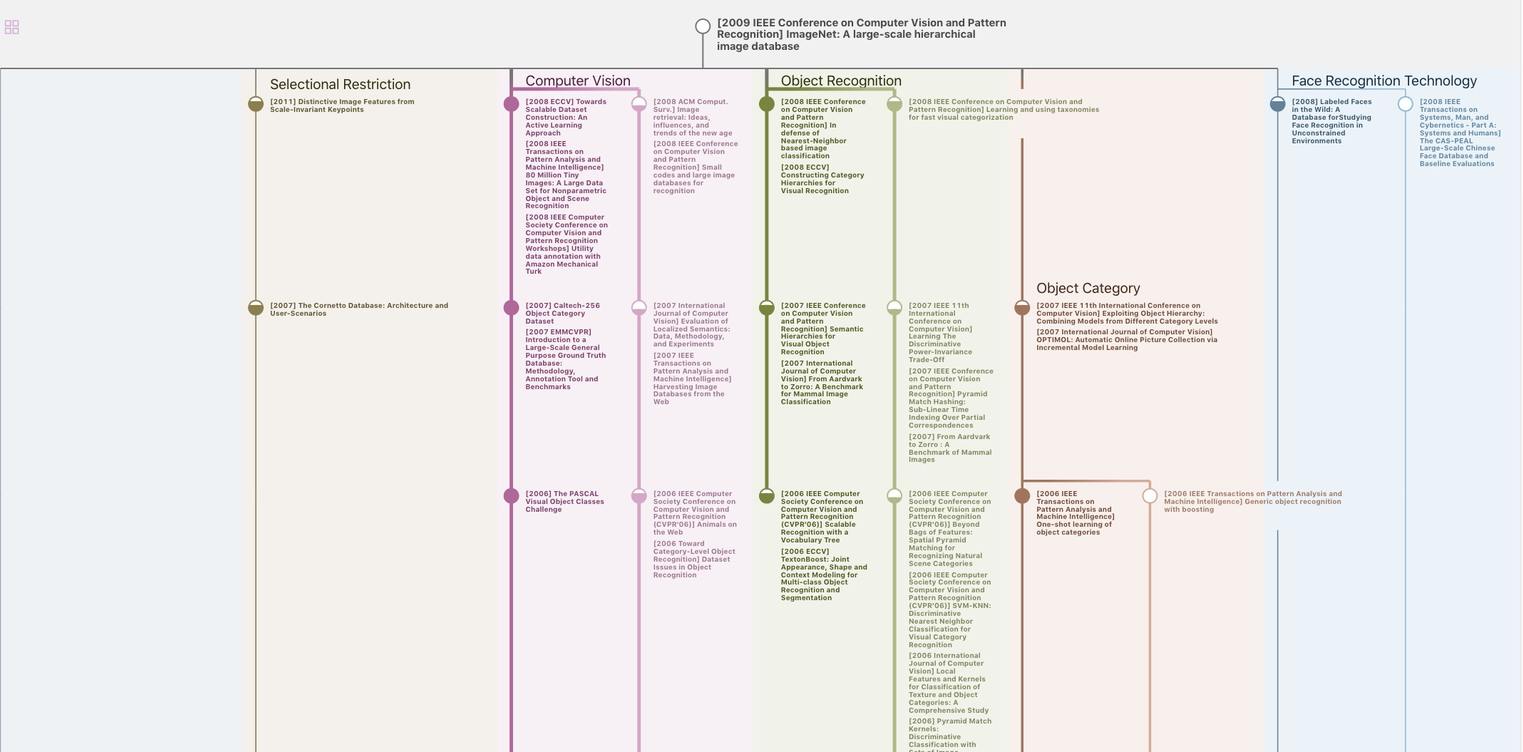
生成溯源树,研究论文发展脉络
Chat Paper
正在生成论文摘要