Automatic Detection of Sand Fouling Levels in Railway Tracks Using Supervised Machine Learning: A Case Study from Saudi Arabian Railway
Arabian Journal for Science and Engineering(2022)
摘要
The presence of sand material in railway tracks, known as sand fouling, can yield unfavorable consequences, resulting in track ballast contamination and causing instability and rapid degradation. Severe sand fouling is a critical problem, especially in desert areas; it can affect the reliability and safety of railway systems and disrupt their services. Therefore, continuous monitoring and periodic maintenance of railway tracks are critical for ensuring smooth and safe operations of railway services. However, one challenge is that the monitoring and inspection of railway tracks for fouled regions is a manual and time-consuming task that railway engineers perform visually. In this study, we addressed this challenge by using machine learning techniques to learn models for the automated detection of sand fouling in railway tracks. We evaluated these models using a sample of railway track geometry data provided by the Saudi Rail Company. The findings of our study revealed that both random forest and artificial neural networks achieved excellent sand fouling detection. Thus, developing an intelligent tool that can identify different sand fouling levels by incorporating the railway geometry data into supervised machine learning algorithms can be highly effective and may assist railway engineers in identifying fouled regions in railway tracks.
更多查看译文
关键词
Ballast fouling,Railway engineering,Artificial intelligence,Machine learning,Railway geometry data
AI 理解论文
溯源树
样例
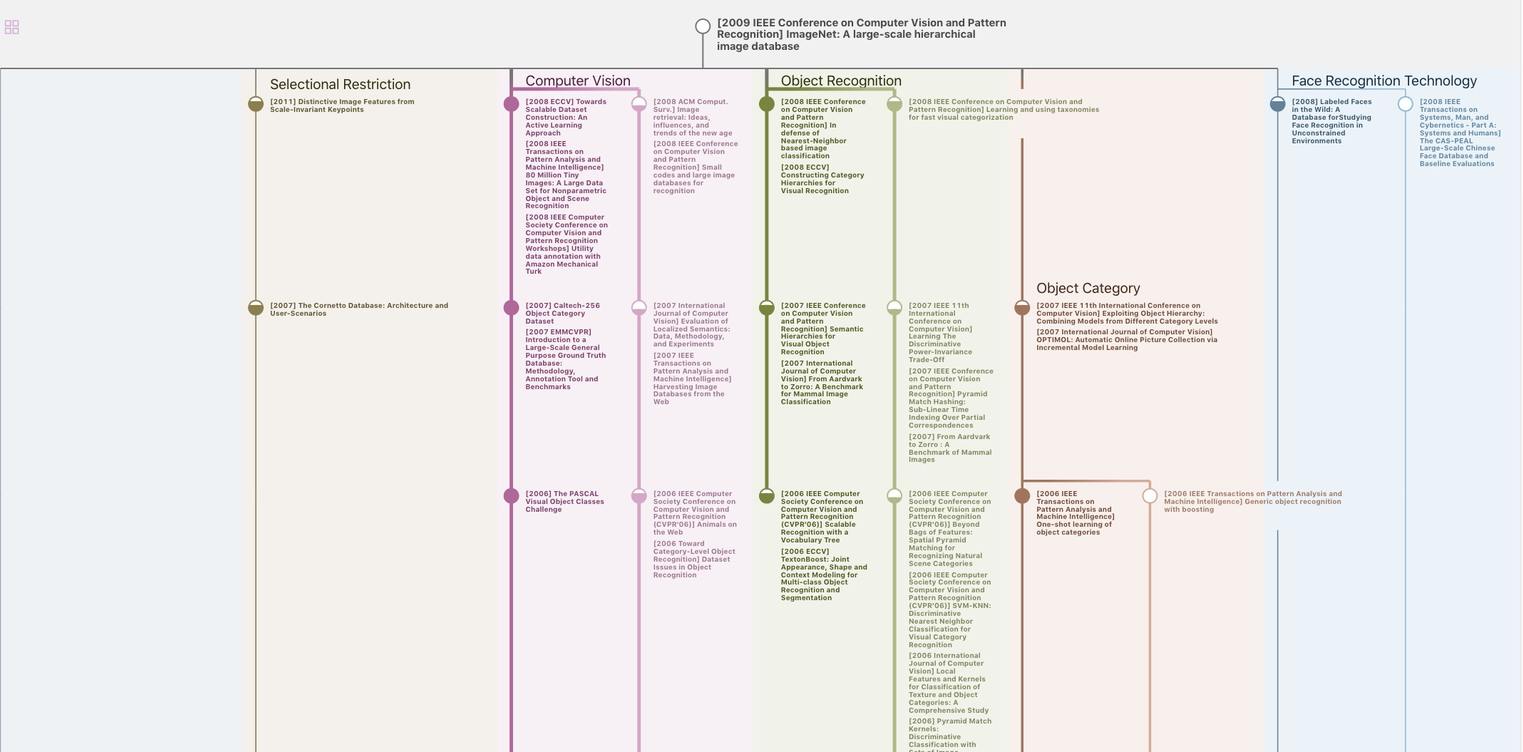
生成溯源树,研究论文发展脉络
Chat Paper
正在生成论文摘要