Exploiting semantic segmentation to boost reinforcement learning in video game environments
MULTIMEDIA TOOLS AND APPLICATIONS(2022)
摘要
In this work we explore enhancing performance of reinforcement learning algorithms in video game environments by feeding it better, more relevant data. For this purpose, we use semantic segmentation to transform the images that would be used as input for the reinforcement learning algorithm from their original domain to a simplified semantic domain with just silhouettes and class labels instead of textures and colors, and then we train the reinforcement learning algorithm with these simplified images. We have conducted different experiments to study multiple aspects: feasibility of our proposal, and potential benefits to model generalization and transfer learning. Experiments have been performed with the Super Mario Bros video game as the testing environment. Our results show multiple advantages for this method. First, it proves that using semantic segmentation enables reaching higher performance than the baseline reinforcement learning algorithm without modifying the actual algorithm, and in fewer episodes; second, it shows noticeable performance improvements when training on multiple levels at the same time; and finally, it allows to apply transfer learning for models trained on visually different environments. We conclude that using semantic segmentation can certainly help reinforcement learning algorithms that work with visual data, by refining it. Our results also suggest that other computer vision techniques may also be beneficial for data prepossessing. Models and code will be available on github upon acceptance.
更多查看译文
关键词
Semantic segmentation,Reinforcement learning,Domain adaptation,Synthetic data
AI 理解论文
溯源树
样例
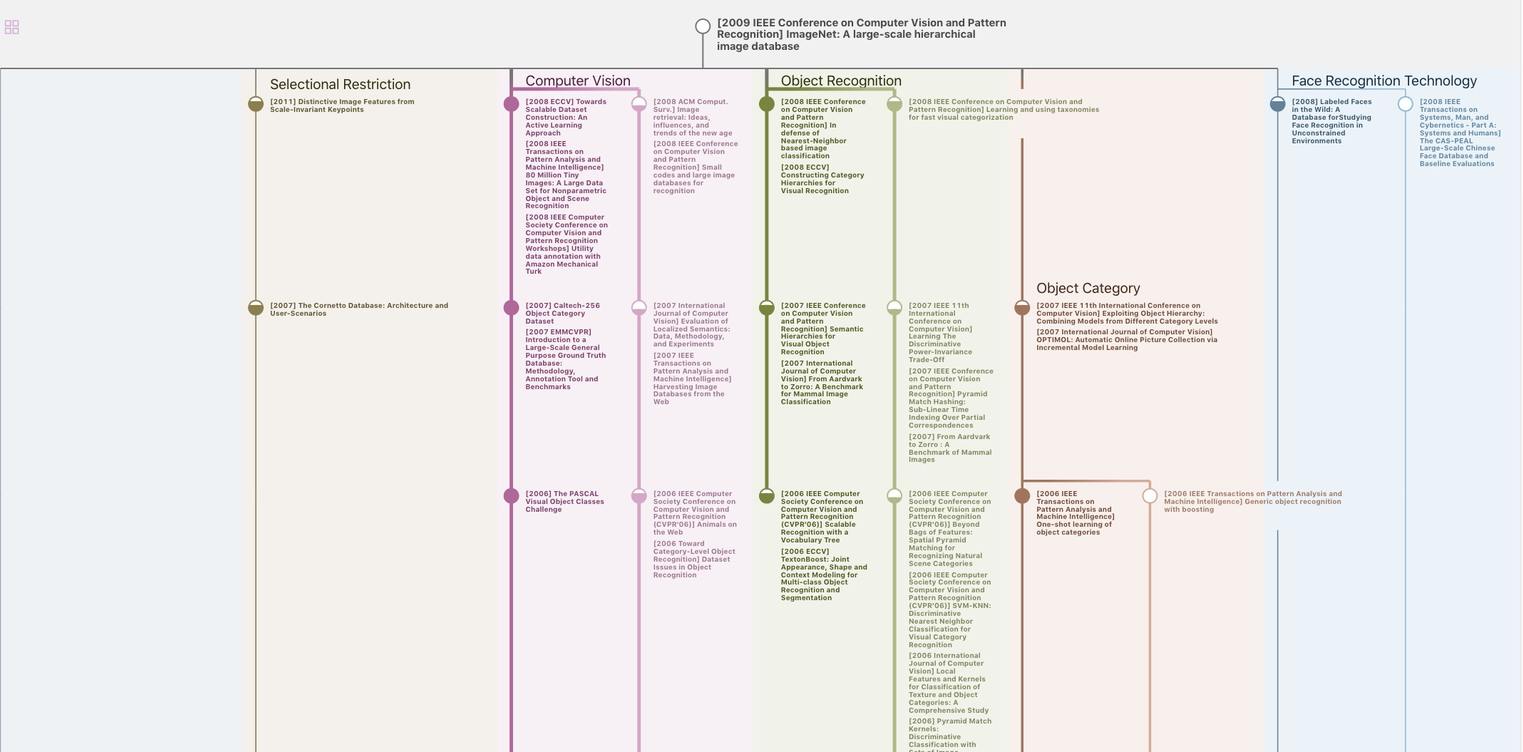
生成溯源树,研究论文发展脉络
Chat Paper
正在生成论文摘要