Predicting clothing attributes with CNN and SURF based classification model
MULTIMEDIA TOOLS AND APPLICATIONS(2022)
摘要
Fashion industry utilizes learning-based strategy for e-commerce applications such as apparel recognition, apparel search, and apparel attribute detection. However, apparel recognition is challenging due to variability in tags for similarly looking apparels. This work utilizes a combination of Convolutional Neural Network and Speed up Robust Feature to propose a framework for efficient apparel recognition. Research highlights that a good quality cloth image provides more information and hence better feature extraction. This work thus proposes Atmospheric Dark Light Adjustment (ADLA) technique to enhance image in the pre-processing phase of the framework. Effectiveness of the proposed integration (ADLA, SURF, and CNN) is validated by experimental results using six apparel attributes on three datasets, namely LSF, DeepFashion2, and Consumer to shop. Results obtained for various performance parameters ensure the desired accuracy. Experimental results are computed for further performance analysis using precision, recall, f-measure, and accuracy parameters. Results show improvements by nearly 4%, 10%, 6%, and 4%, respectively, for the same as compared to CNN alone. Comparison with prior mechanisms considering LSF dataset also highlights the superiority of the proposed framework. Overall, the proposed framework using ADLA with SURF-CNN enhances the performance by 4% to 10% using three fashion datasets.
更多查看译文
关键词
Image matching, Image enhancement, Local feature, Neural network
AI 理解论文
溯源树
样例
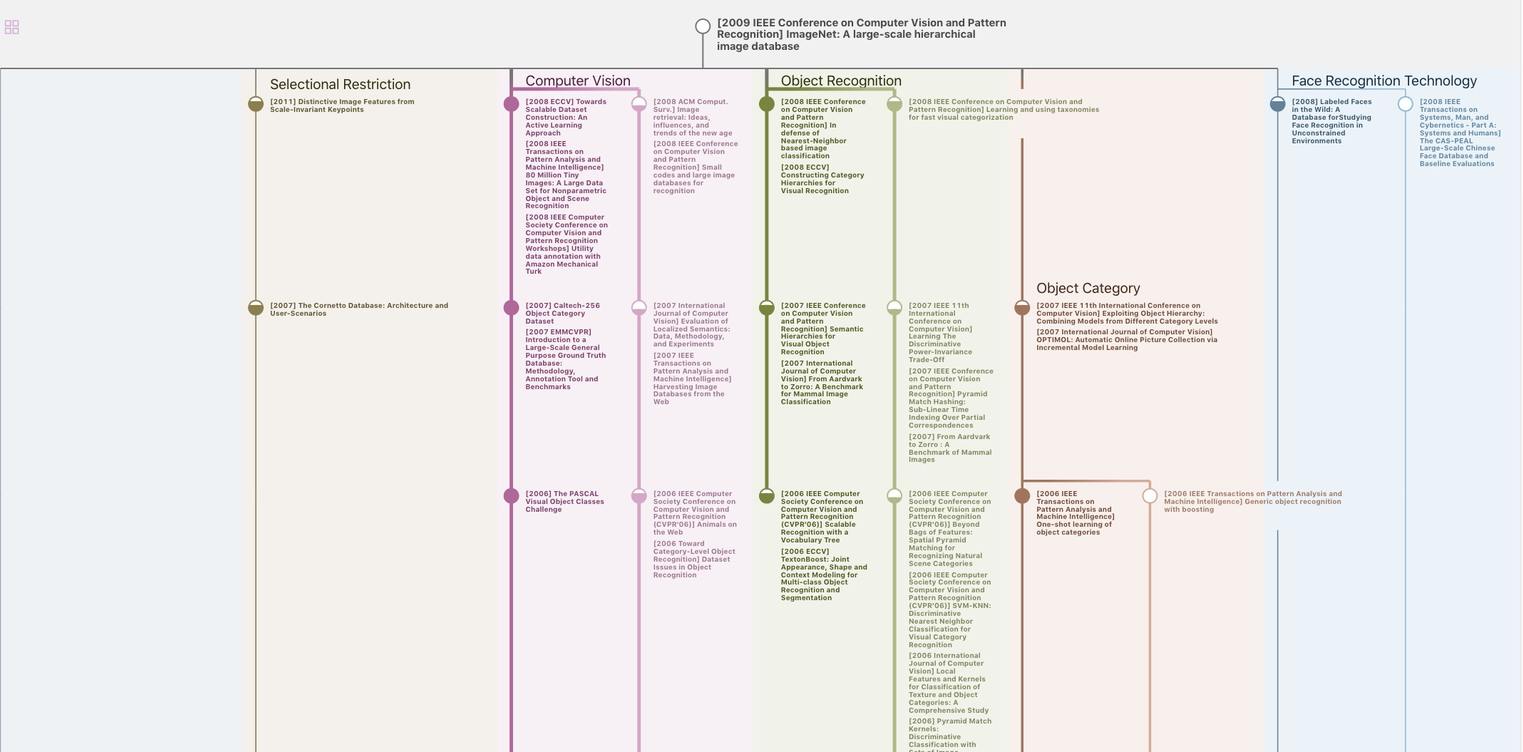
生成溯源树,研究论文发展脉络
Chat Paper
正在生成论文摘要