Performance Analysis and Power Allocation for Spatial Modulation-Aided MIMO-LDM With Finite Alphabet Inputs
IEEE Transactions on Broadcasting(2023)
摘要
In this paper, we study a spatial modulation (SM) aided multiple-input multiple-output (MIMO) layered division multiplexing (LDM) system for broadcast/multicast service delivery in future broadcast/multicast systems. Comprehensive performance analysis and the injection level (IL) optimization are investigated for the SM-aided MIMO-LDM system over correlated Rayleigh fading channels. First, the symbol detection pairwise error probability (PEP) and the average symbol error rate (SER) union bound under joint maximum-likelihood (ML) detection are derived. Second, the spectral efficiency (SE) of the SM-aided MIMO-LDM system with finite alphabet inputs is analyzed. Since the theoretical SE lacks closed-form expression and involves prohibitive computational complexity, we then derive the closed-form lower bounds and tight approximations for the theoretical SE, in which the computational complexity is relieved by several orders of magnitude compared to direct calculation of the theoretical SE. Third, based on the SE analysis, a weighted sum (WS) SE maximization problem with quality of service (QoS) constraints is formulated to optimize IL for the SM-aided MIMO-LDM system. We first demonstrate that WS SE is a unimodal function of IL, and then develop an efficient golden section search (GSS) based algorithm. Simulation results are provided to validate the theoretical analysis. It is shown that our derived SER union bound well matches the simulated SER in the high SNR region. The tightness of the derived closed-form lower bounds and approximations for theoretical SE and the effectiveness of the developed IL optimization algorithm are also verified by simulation results.
更多查看译文
关键词
Layered division multiplexing (LDM),spatial modulation (SM),symbol error rate (SER),spectral efficiency (SE),finite alphabet inputs,injection level (IL)
AI 理解论文
溯源树
样例
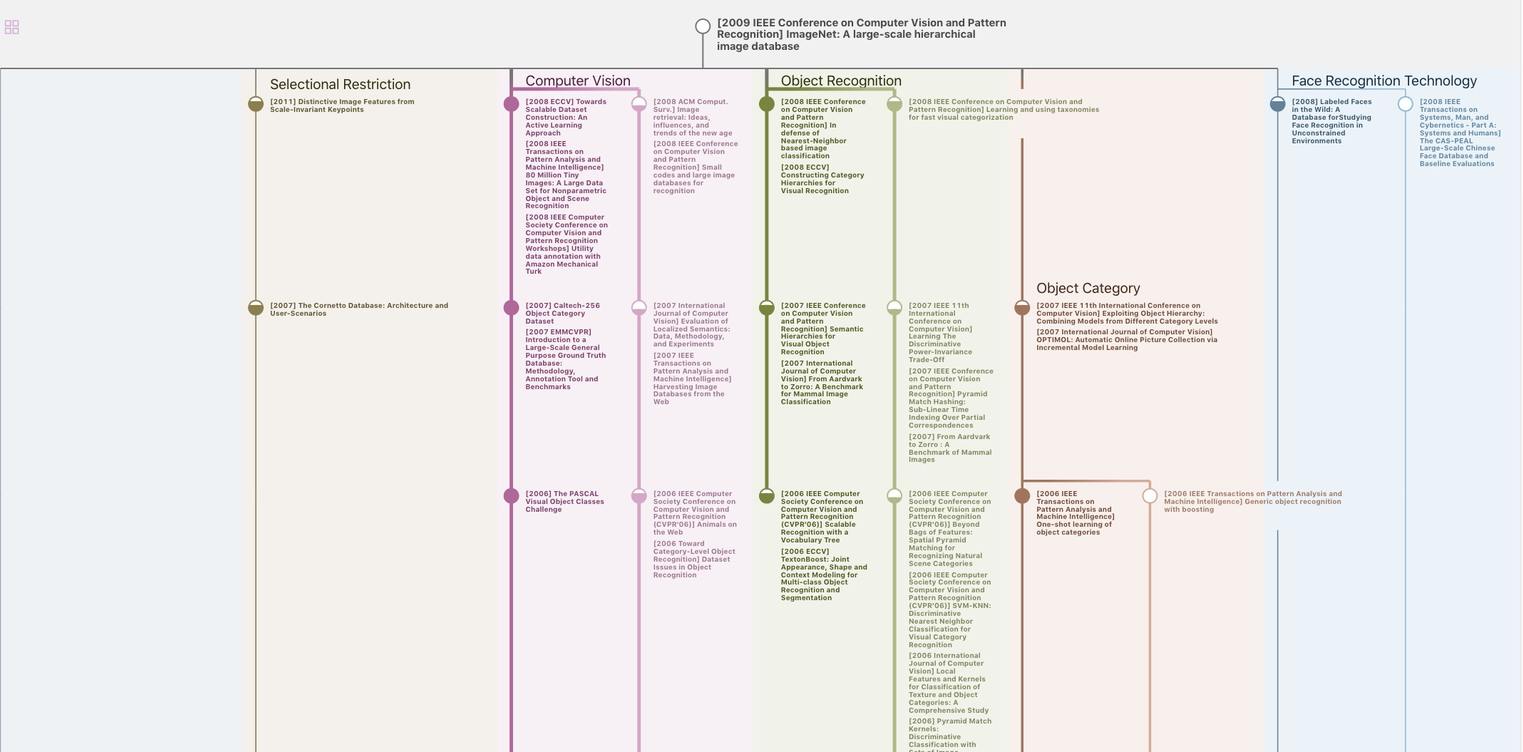
生成溯源树,研究论文发展脉络
Chat Paper
正在生成论文摘要