EQAdap: Equipollent Domain Adaptation Approach to Image Deblurring
IEEE ACCESS(2022)
摘要
In this paper, we present an end-to-end unsupervised domain adaptation approach to image deblurring. This work focuses on learning and generalizing the complex latent space of the source domain and transferring the extracted information to the unlabeled target domain. While fully supervised image deblurring methods have achieved high accuracy on large-scale vision datasets, they are unable to well generalize well on a new test environment or a new domain. Therefore, in this work, we introduce a novel Bijective Maximum Likelihood loss for the unsupervised domain adaptation approach to image deblurring. We evaluate our proposed method on GoPro, RealBlur_J, RealBlur_R, and HIDE datasets. Through intensive experiments, we demonstrate our state-of-the-art performance on the standard benchmarks.
更多查看译文
关键词
Image restoration, Deep learning, Training data, Image reconstruction, Adaptation models, Predictive models, Neural networks, Unsupervised learning, Deep neural networks (DNNs), instance level affinity-domain adaptation (ILA-DA), unsupervised domain adaptation (UDA)
AI 理解论文
溯源树
样例
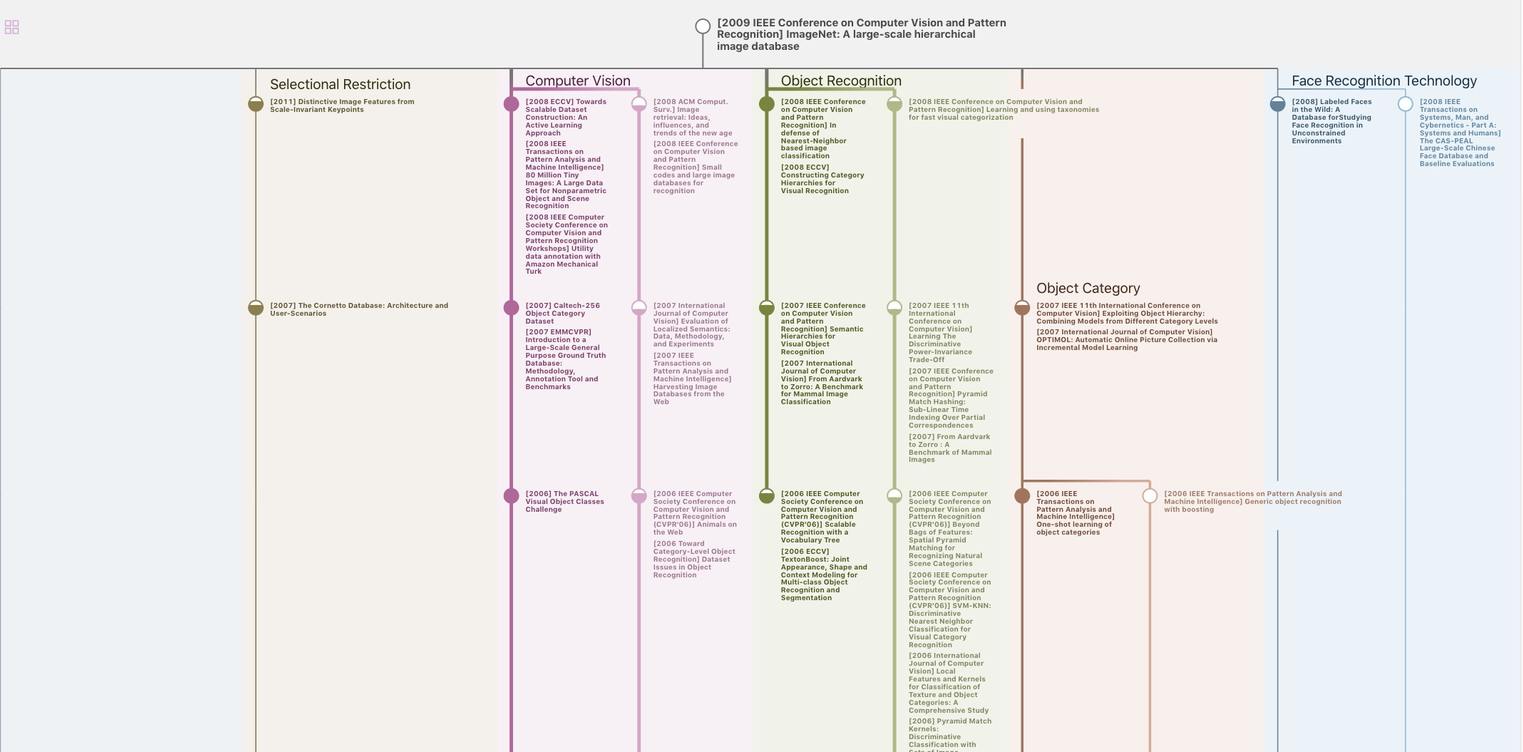
生成溯源树,研究论文发展脉络
Chat Paper
正在生成论文摘要