Fusion-Based Smartphone Positioning Using Unsupervised Calibration of Crowdsourced Wi-Fi FTM
IEEE ACCESS(2022)
摘要
This paper presents a multi-source fusion smartphone localization solution using Wi-Fi Fine Time Measurement (FTM) and Pedestrian Dead Reckoning (PDR), calibrated via multi-source and unsupervised crowdsourcing. In crowdsourcing phase, user movement within the site utilizes PDR to infer their location, and this location is used to calibrate the FTM data. The multi-layer perceptron (MLP) of the ranging model is suitable for non-line-of-sight (NLOS) reception, and the ranging accuracy is improved by more than 24%. In the positioning phase, the 90 percentile error of the ranging model trained using only crowdsourced data is less than 1.37m, which is 32% smaller than the traditional weighted least squares (WLS) localization error.
更多查看译文
关键词
Distance measurement, Wireless fidelity, Position measurement, Location awareness, Crowdsourcing, Unsupervised Learning, Sensors, Dead reckoning, Particle filters, Wi-Fi fine time measurement, pedestrian dead reckoning, crowdsourcing, particle filter, unsupervised learning, fusion-based positioning
AI 理解论文
溯源树
样例
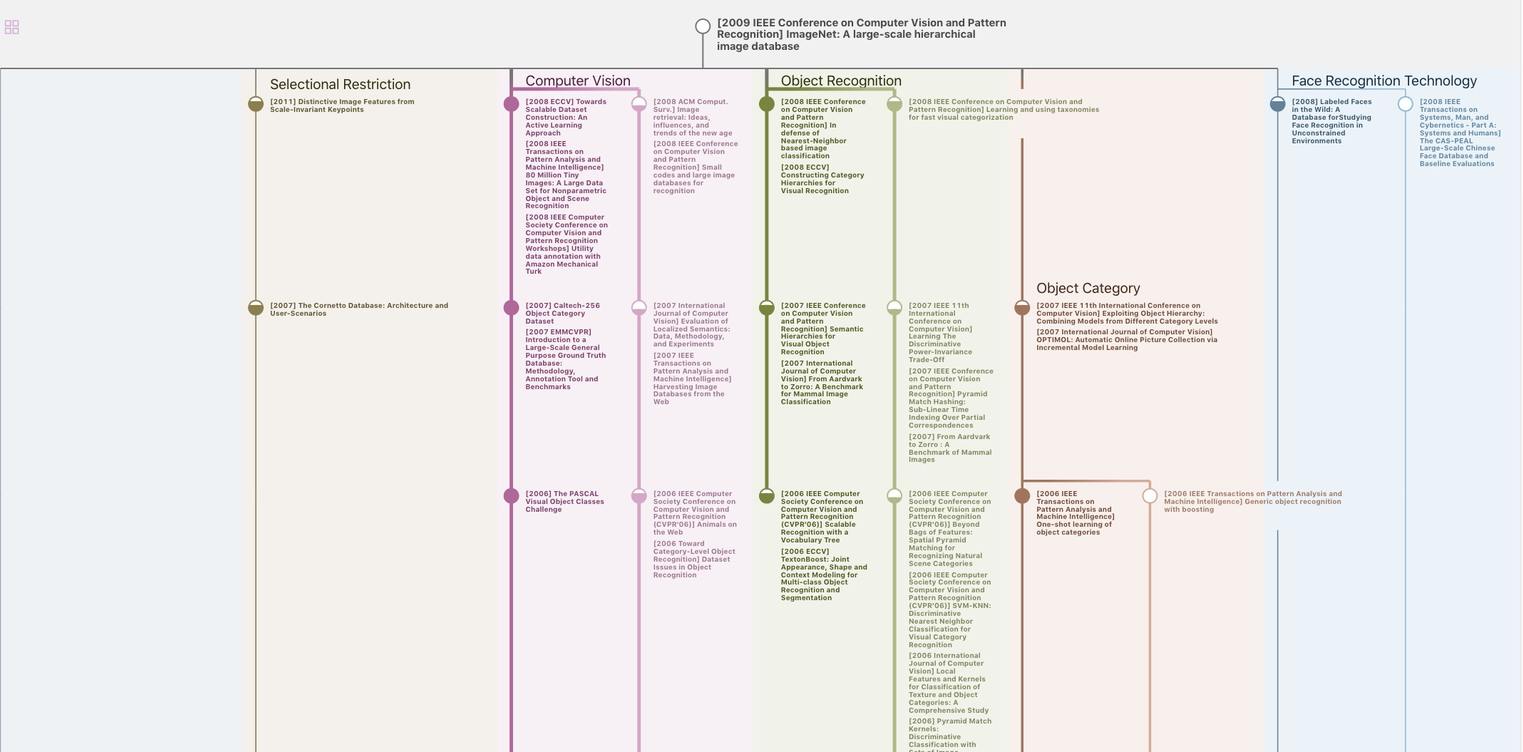
生成溯源树,研究论文发展脉络
Chat Paper
正在生成论文摘要