Application of machine learning algorithms to screen potential biomarkers under cadmium exposure based on human urine metabolic profiles
CHINESE CHEMICAL LETTERS(2022)
摘要
Exposure to environmental cadmium increases the health risk of residents. Early urine metabolic detection using high-resolution mass spectrometry and machine learning algorithms would be advantageous to predict the adverse health effects. Here, we conducted machine learning approaches to screen potential biomarkers under cadmium exposure in 403 urine samples. In positive and negative ionization mode, 4207 and 3558 features were extracted, respectively. We compared seven machine learning algorithms and found that the extreme gradient boosting (XGBoost) and random forest (RF) classifiers showed better accuracy and predictive performance than others. Following 5-fold cross-validation, the value of area under curve (AUC) was both 0.93 for positive and negative ionization modes in XGBoost classifier. In the RF classifier, AUC were 0.80 and 0.84 for positive and negative ionization modes, respectively. We then identified a biomarker panel based on XGBoost and RF classifiers. The incorporation of machine learning models into urine analysis using high-resolution mass spectrometry could allow a convenient assessment of cadmium exposure. (c) 2022 Published by Elsevier B.V. on behalf of Chinese Chemical Society and Institute of Materia Medica, Chinese Academy of Medical Sciences.
更多查看译文
关键词
Human urine, High-resolution mass spectrometry, Cadmium exposure, Metabolic profiles, Machine learning
AI 理解论文
溯源树
样例
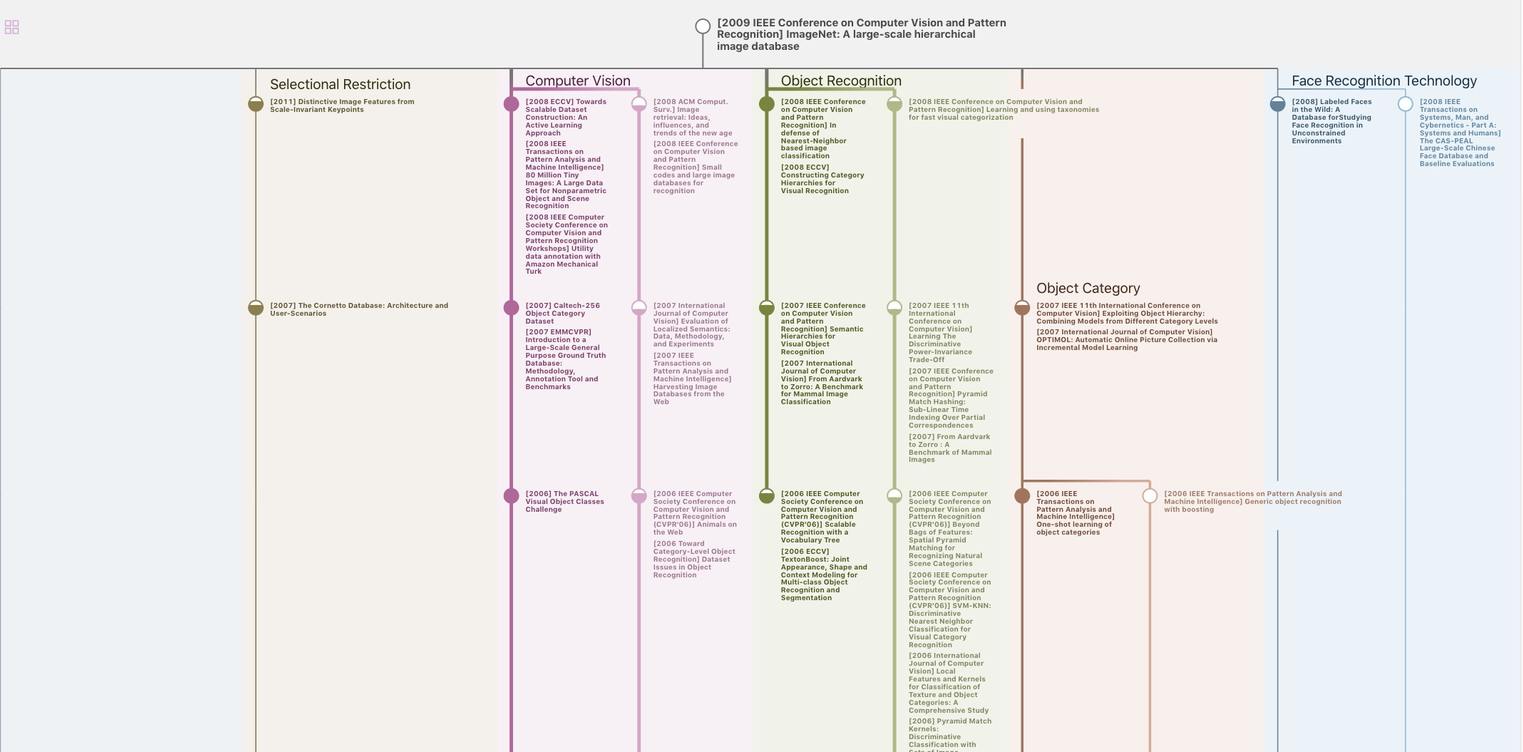
生成溯源树,研究论文发展脉络
Chat Paper
正在生成论文摘要