Abnormal Communication Signals Recognition Based on Image Enhancement and Improved Memory-Augmented Autoencoder
WIRELESS COMMUNICATIONS & MOBILE COMPUTING(2022)
摘要
Detecting increasing anomalous signals is critical to effective spectrum management due to the complexity of the use of electromagnetic spectrum. The anomaly recognition approach based on autoencoder (AE) heavily relies on the assumption that the reconstruction error of normal signals is generally lower than that of abnormal signals. Unfortunately, such an assumption does not necessarily hold due to the excessive generalization ability of the AE. The memory-augmented autoencoder model (MemAE) has been recently proposed to address this issue by introducing memory modules. Still, the standard MemAE model performs poorly on complex image datasets, and its performance for abnormal communication signals recognition is unknown. Therefore, we propose an image enhancement and improved memory-augmented autoencoder model (IIMemAE) to recognize abnormal communication signals. Specifically, we consider two key factors, i.e., the existence of redundant information in the time-frequency spectrogram and the low recognition accuracy of normal signals of the standard MemAE model. We introduce an image enhancement module and an anomaly determination module compared with the standard MemAE model. The proposed IIMemAE model can address the issue that the performance of anomaly recognition may be degraded due to the imbalanced communication signals in the real world. The simulation results show that IIMemAE can effectively recognize synthetic abnormal communication signals even at low signal-to-noise ratio (SNR) and jamming-to-signal ratio (JSR) conditions and outperform the standard MemAE model. Besides, the parametric Pauta criterion proposed can balance the recognition accuracy of normal and abnormal signals to meet the need for diverse recognition tasks.
更多查看译文
AI 理解论文
溯源树
样例
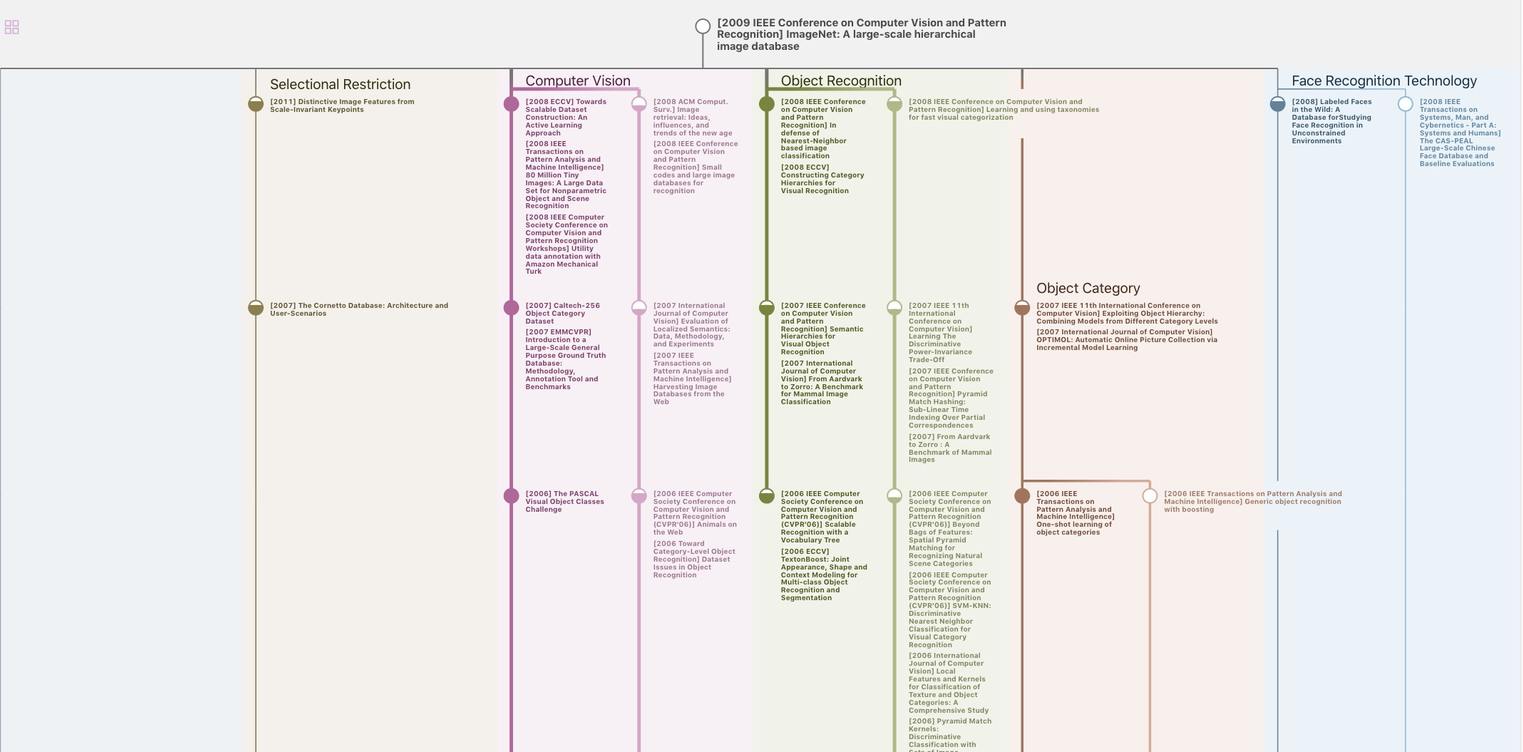
生成溯源树,研究论文发展脉络
Chat Paper
正在生成论文摘要