Malicious Code Classification Method Based on Deep Residual Network and Hybrid Attention Mechanism for Edge Security
WIRELESS COMMUNICATIONS & MOBILE COMPUTING(2022)
摘要
Edge computing is a feasible solution for effectively collecting and processing data in industrial Internet of Things (IIoT) systems, and edge security is an important guarantee for edge computing. Fast and accurate classification of malicious code in the whole lift cycle of edge computing is of great significance, which can effectively prevent malicious code from attacking wireless sensor networks and ensure the stable and secure transmission of data in smart devices. Considering that there is a large amount of code reuse in the same malicious code family, making their visual feature similar, many studies use visualization technology to assist malicious code classification. However, traditional malicious code visual classification schemes have the problems such as single image source, weak ability of deep-level feature extraction, and lack of attention to key image details. Therefore, an innovative malicious code visual classification method based on a deep residual network and hybrid attention mechanism for edge security is proposed in this study. Firstly, the malicious code visualization scheme integrates the bytecode file and assembly file of the malware and converts them into a four-channel RGBA image to fully represent malicious code feature information without increasing the computational complexity. Secondly, a hybrid attention mechanism is introduced into the deep residual network to construct an effective classification model, which extracts image texture features of malicious code from two dimensions of the channel and spatial to improve the classification performance. Finally, the experimental results on the BIG2015 and Malimg datasets show that the proposed scheme is feasible and effective and can be widely applied used in various malicious code classification issues, and the classification accuracy rate is relatively higher than the existing better-performing malicious code classification methods.
更多查看译文
关键词
malicious code classification method,edge security,deep residual network,hybrid attention mechanism
AI 理解论文
溯源树
样例
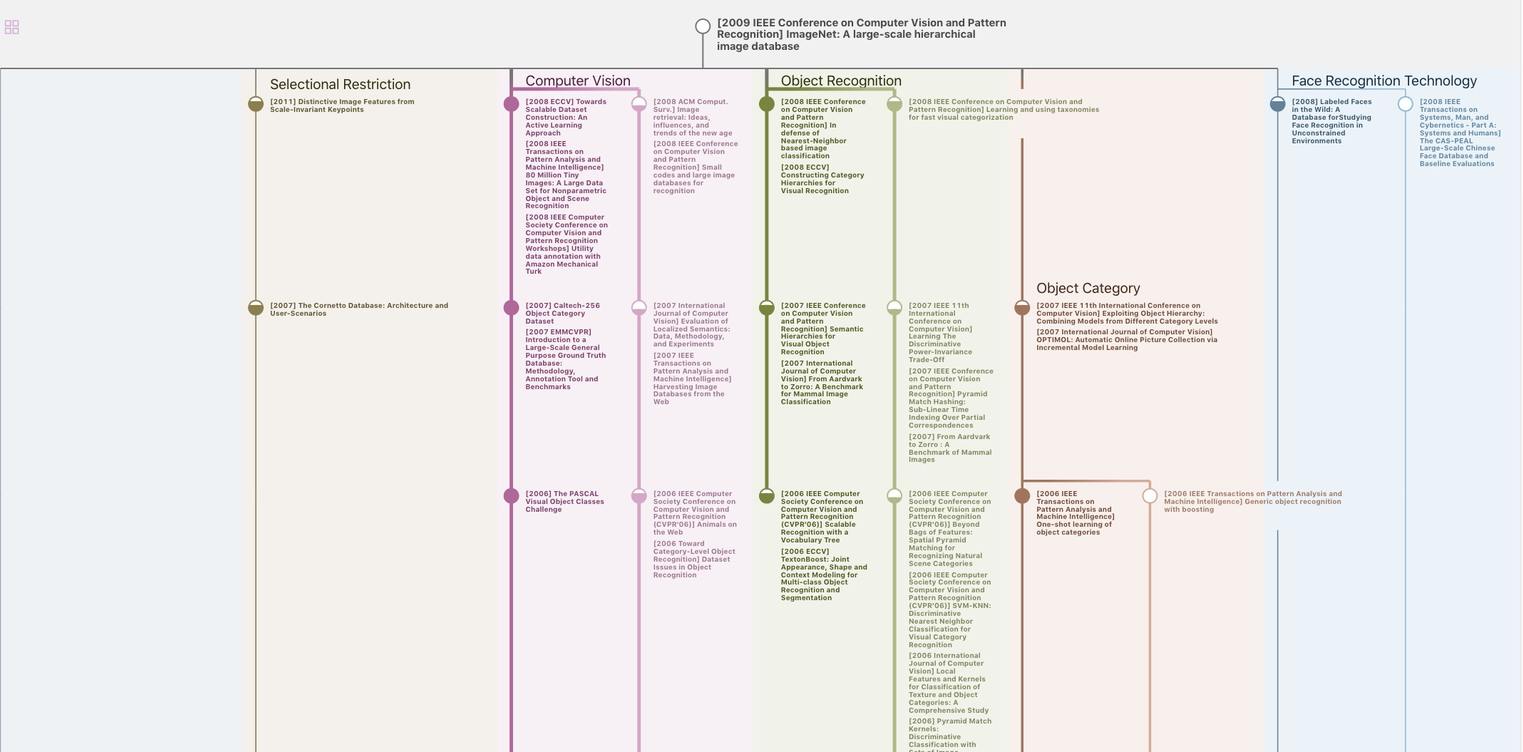
生成溯源树,研究论文发展脉络
Chat Paper
正在生成论文摘要