Machine Learning for Model Predictive Control of Cascaded H-Bridge Inverters
2022 IEEE 21ST MEDITERRANEAN ELECTROTECHNICAL CONFERENCE (IEEE MELECON 2022)(2022)
摘要
Finite Control Set Model Predictive Control (MPC) is an effective control technique for Cascaded H-Bridge converters. However, its computational complexity becomes impractical when the number of levels of the converter increases. Machine Learning techniques can be successfully used to reduce the computational burden of the optimal control computation and this paper provides a comparison among conventional MPC and well-known Machine Learning techniques: Support Vector Machines, Regression Trees, Neural Networks and Linear Regression. A simulation study is presented for a Cascaded HBridge Static Synchronous Compensator varying the number of levels and using different Machine Learning control strategies. The results underline that some ML techniques can substantially reduce computational complexity while keeping the performance comparable with the optimal control.
更多查看译文
关键词
cascaded H-bridge inverters,finite control set model predictive control,cascaded H-bridge converters,computational complexity,machine learning techniques,optimal control computation,conventional MPC,support vector machines,cascaded H-bridge static synchronous compensator,ML techniques,regression trees,neural networks,linear regression
AI 理解论文
溯源树
样例
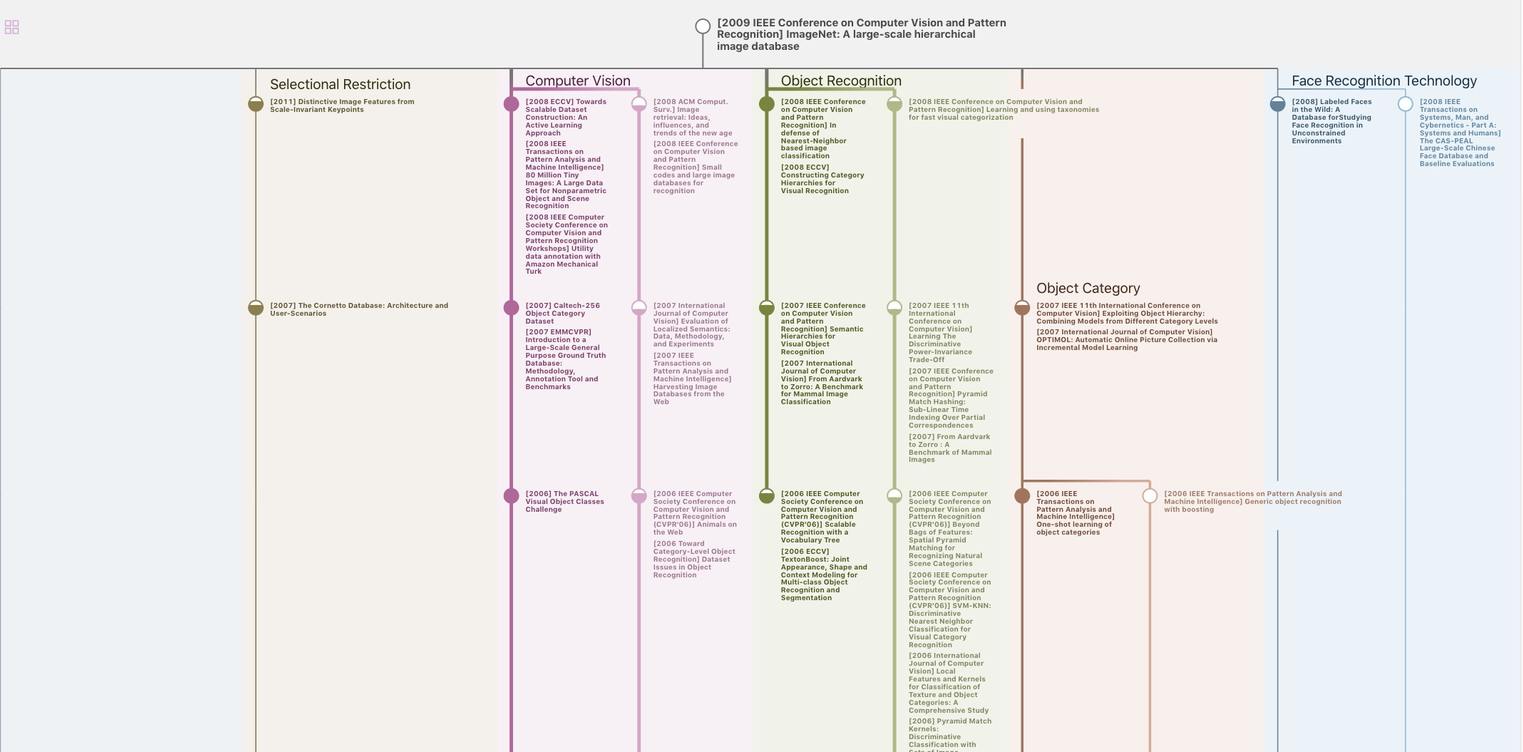
生成溯源树,研究论文发展脉络
Chat Paper
正在生成论文摘要