Probabilistically Robust Radar Waveform Design for Extended Target Detection
IEEE TRANSACTIONS ON SIGNAL PROCESSING(2022)
摘要
We address radar waveform design problem for extended targets under the Peak-to-Average Ratio (PAR) constraint. Based on the stochastic model of the Target Impulse Response (TIR), the distribution of output Signal-to-Interference-pulse-Noise Ratio (SINR) is derived. After that, a novel waveform design metric, named Probabilistically Robust Detection (PRD), is proposed to improve the stability of detection performance against the stochastic TIR. The geometric and limit interpretations for the PRD metric are given to show its rationality. Leveraging on the Semi-Definite Relaxation schemes and the Dinkelbach's algorithm, we devise algorithms to optimize waveform covariance matrix based on the proposed metric in high and low SINR situations, respectively. It is theoretically proved that the global optimal waveform covariance matrix can be achieved in high SINR situations. On the contrary, only the sub-optimal waveform covariance matrix can be obtained in low SINR situations. The transmit waveform is synthesized from the optimized waveform covariance matrix through randomization schemes. Numerical experiments are carried out to evaluate the PRD metric as well as the proposed algorithms. Results verify the effectiveness of the proposed algorithms. Compared with the current design metric, the proposed PRD metric exhibits strong probabilistic robustness on detecting extended targets.
更多查看译文
关键词
Signal to noise ratio, Interference, Measurement, Radar, Radar detection, Probabilistic logic, Optimization, Radar waveform design, extended targets, Signal-to-Interference-pulse-Noise Ratio (SINR), Probabilistically Robust Detection (PRD) metric, Dinkelbach's algorithm
AI 理解论文
溯源树
样例
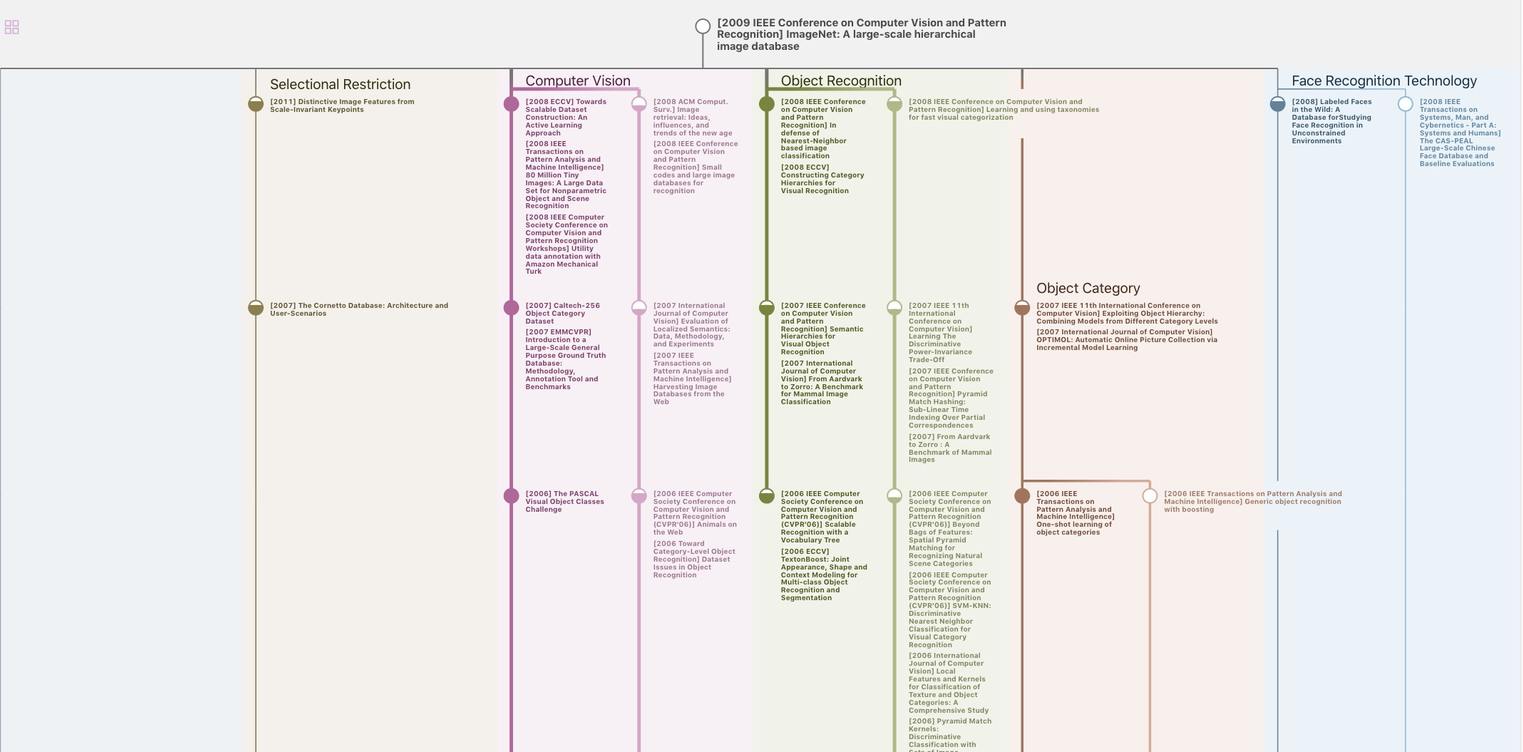
生成溯源树,研究论文发展脉络
Chat Paper
正在生成论文摘要