Uncertainty Management in Lebesgue-Sampling-Based Li-Ion Battery SFP Model for SOC Estimation and RDT Prediction
IEEE-ASME TRANSACTIONS ON MECHATRONICS(2023)
摘要
The state-of-charge (SOC) estimation and remaining-dischargeable-time (RDT) prediction based on the simplified first principle (SFP) model under Lebesgue sampling (LS) has advantages of less computation and higher performance. The accuracy, precision, and convergence speed of SOC estimation and RDT prediction are significantly influenced by the model. This article introduces an online dichotomy method for the parameter adaptation of the LS-based SFP model to improve the accuracy and convergence speed. Besides, the uncertainty of RDT prediction is managed by adjusting model noises through a short-term correction loop to improve the precision of RDT prediction. The developed SFP is then integrated with an extended Kalman filter (EKF) in the LS framework for lithium-ion battery SOC estimation and RDT prediction. Experimental results show that the proposed approach can greatly improve the performance in terms of convergence speed, accuracy, and computing efficiency. Compared with the LS-EKF, the convergence speed and RDT prediction accuracy of the proposed method are improved by 98.6% and 47.4% under dynamic loading profiles, respectively. The absolute average error of SOC estimation keeps in the range of 2%.
更多查看译文
关键词
Extended Kalman filter (EKF),Lebesgue sampling (LS),remaining dischargeable time (RDT),simplified first principle (SFP) model,state of charge (SOC),uncertainty management (UM)
AI 理解论文
溯源树
样例
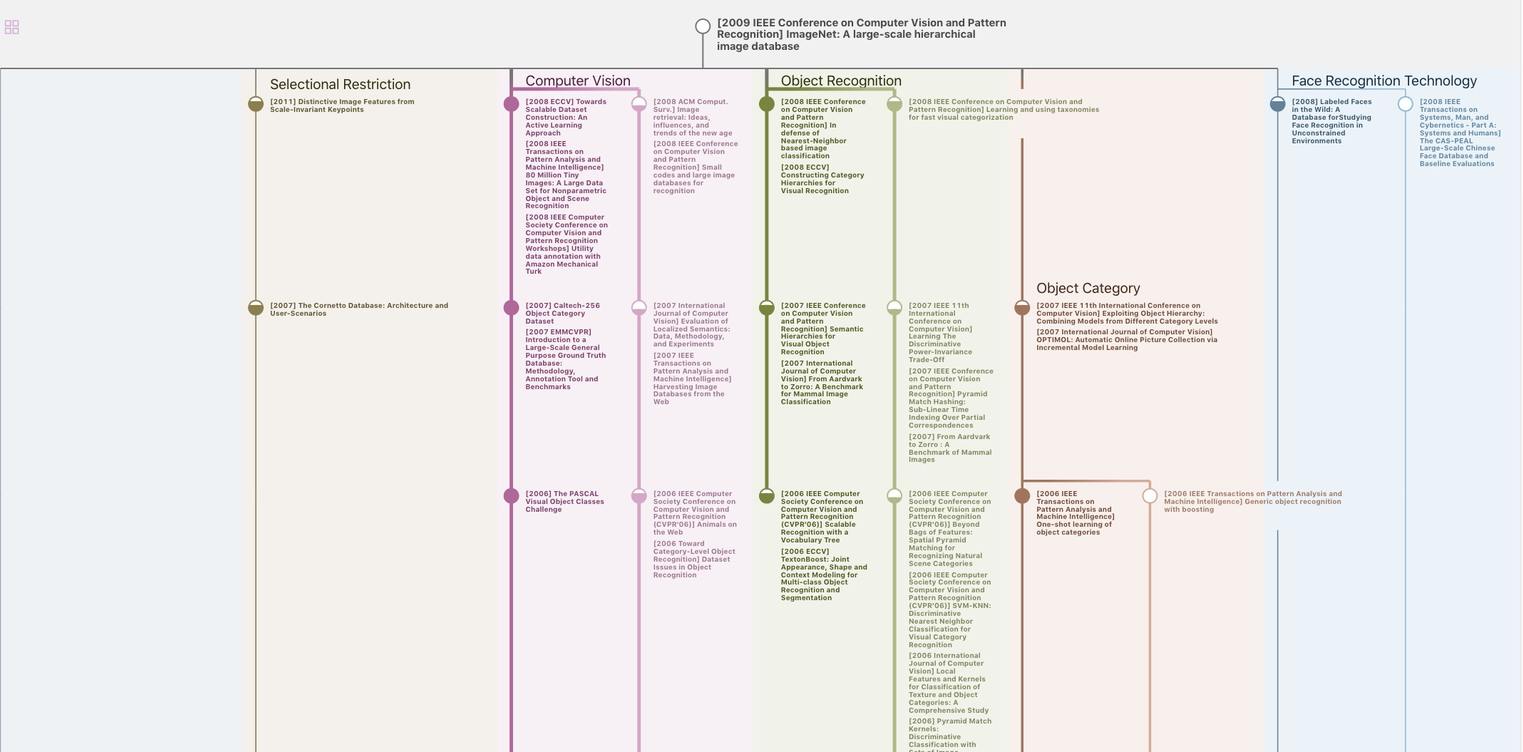
生成溯源树,研究论文发展脉络
Chat Paper
正在生成论文摘要