Machine Learning-Aided Prediction of Post-Fire Shear Resistance Reduction of Q690 HSS Plate Girders
BUILDINGS(2022)
摘要
Fire has significant effects on the residual resistance of steel structures. It is necessary to accurately clarify its effects on Q690 HSS plate girders, which have been widely used. In this paper, the ultimate resistance and effective service resistance of Q690 HSS plate girders after a fire are obtained using material tests and finite element (FE) analysis including parametric studies, where the data of 210 models were collected. The effects of four key parameters (h(w)/t(w) ratio, a/h(w) ratio, exposure temperature and cooling method) on post-fire shear resistance reduction of Q690 HSS plate girders are roughly investigated by individual conditional expectation (ICE), showing exposure temperature is the most important factor. The popular algorithms of machine learning (ML), namely artificial neural network (ANN) and support vector regression (SVR) algorithms, are utilized in model training to predict the reduction factors of both ultimate resistance and effective service resistance. Finally, the results indicate that the prediction using ML shows much better performance than that with traditional ordinary least squares (OLS) regression, and SVR with genetic algorithm (GA) provides the highest prediction accuracy. The results of this paper show the superiority of machine learning for solving prediction problems of steel structures, compared with conventional methods such as linear regression.
更多查看译文
关键词
artificial neural network, high-strength steel, plate girder, post-fire, machine learning, support vector regression
AI 理解论文
溯源树
样例
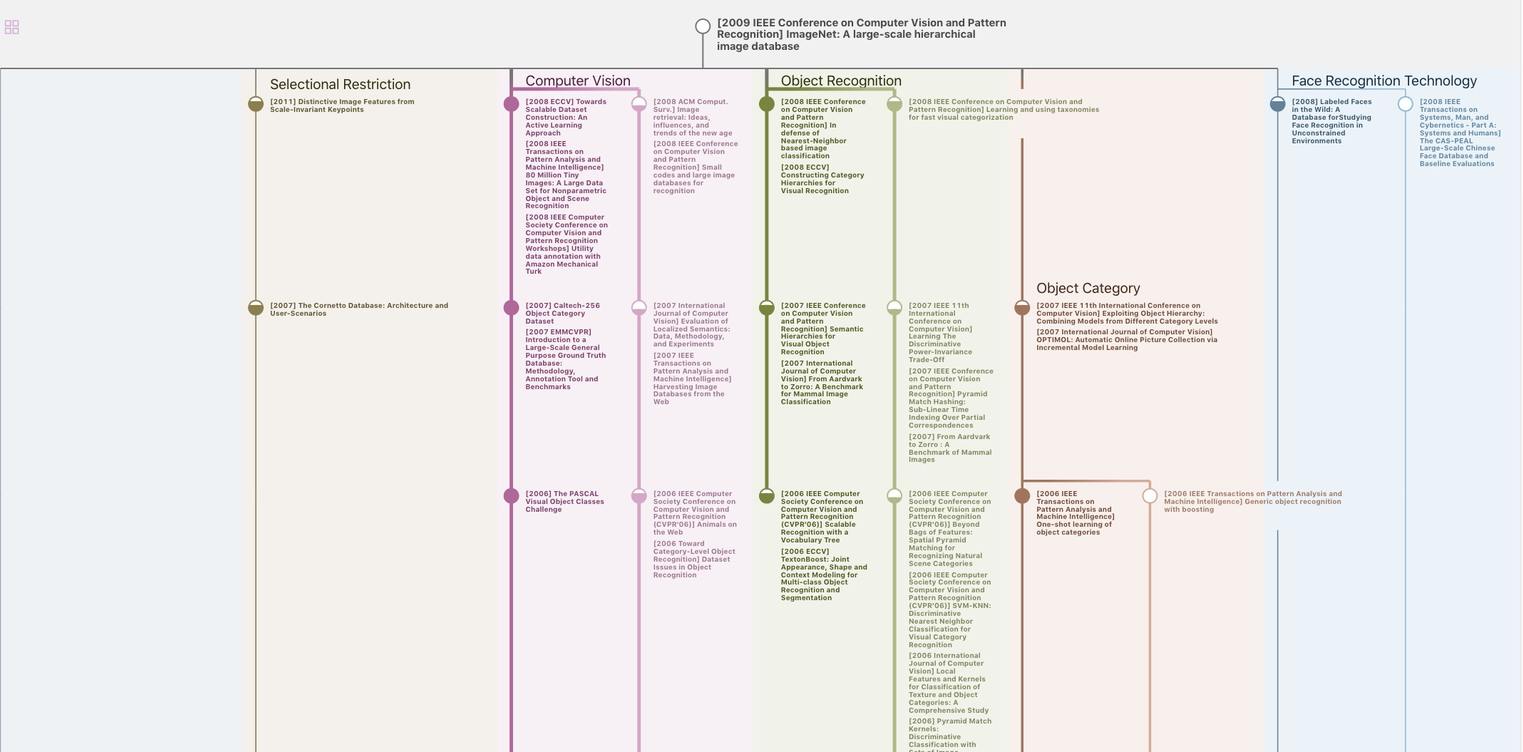
生成溯源树,研究论文发展脉络
Chat Paper
正在生成论文摘要