Direct prediction of genetic aberrations from pathology images in gastric cancer with swarm learning
Gastric cancer : official journal of the International Gastric Cancer Association and the Japanese Gastric Cancer Association(2022)
摘要
Background Computational pathology uses deep learning (DL) to extract biomarkers from routine pathology slides. Large multicentric datasets improve performance, but such datasets are scarce for gastric cancer. This limitation could be overcome by Swarm Learning (SL). Methods Here, we report the results of a multicentric retrospective study of SL for prediction of molecular biomarkers in gastric cancer. We collected tissue samples with known microsatellite instability (MSI) and Epstein–Barr Virus (EBV) status from four patient cohorts from Switzerland, Germany, the UK and the USA, storing each dataset on a physically separate computer. Results On an external validation cohort, the SL-based classifier reached an area under the receiver operating curve (AUROC) of 0.8092 (± 0.0132) for MSI prediction and 0.8372 (± 0.0179) for EBV prediction. The centralized model, which was trained on all datasets on a single computer, reached a similar performance. Conclusions Our findings demonstrate the feasibility of SL-based molecular biomarkers in gastric cancer. In the future, SL could be used for collaborative training and, thus, improve the performance of these biomarkers. This may ultimately result in clinical-grade performance and generalizability.
更多查看译文
关键词
Gastric cancer,Pathology,Biomarker,Artificial intelligence,Blockchain,Swarm learning
AI 理解论文
溯源树
样例
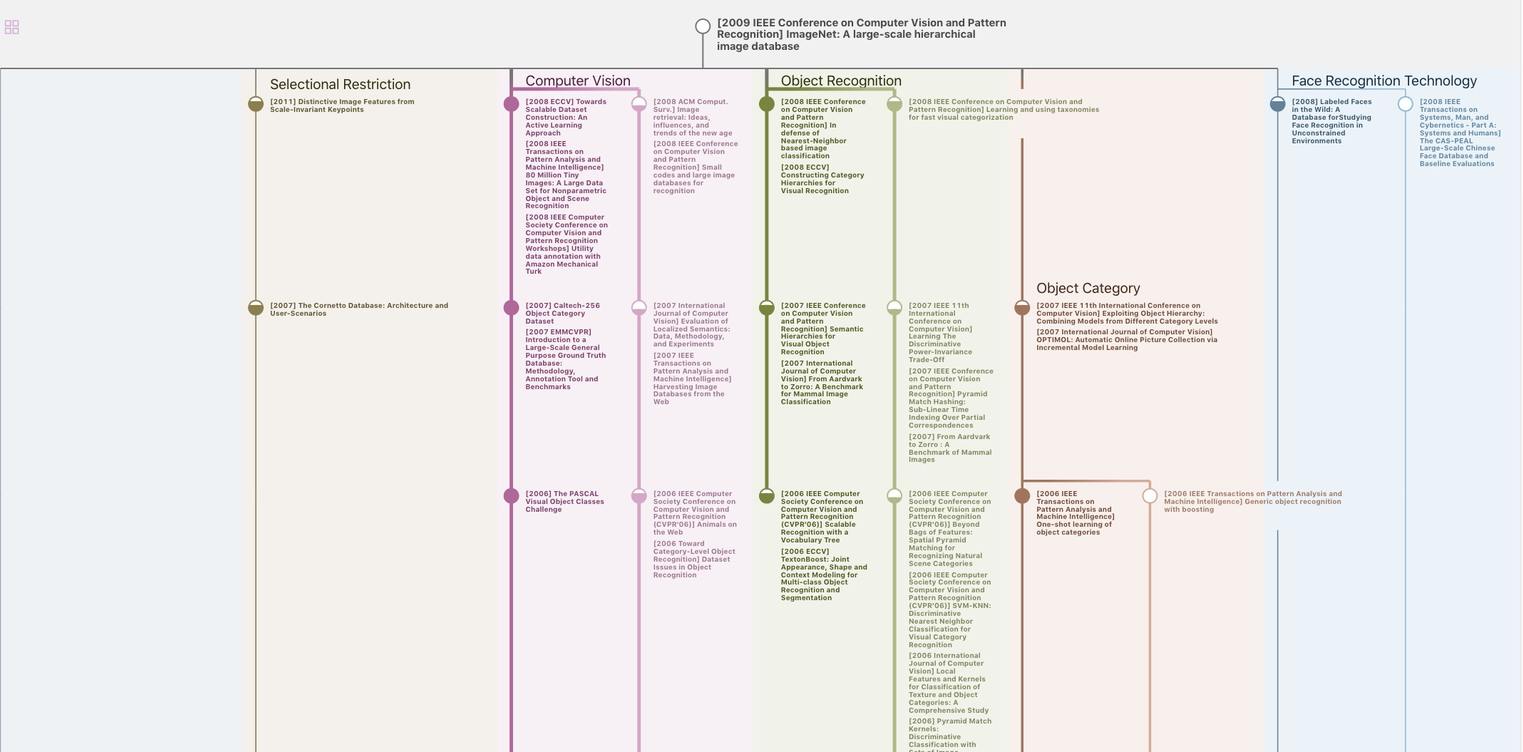
生成溯源树,研究论文发展脉络
Chat Paper
正在生成论文摘要