Effectiveness of Human-in-the-Loop Sentiment Polarization with Few Corrected Labels
INTERNATIONAL JOURNAL OF ADVANCED COMPUTER SCIENCE AND APPLICATIONS(2022)
摘要
In this work, we investigated the effectiveness of adopting Human-in-the-Loop (HITL) aimed to correct automatically generated labels from existing scoring models, e.g. SentiWordNet and Vader to enhance prediction accuracy. Recently, many proposals showed a trend in utilizing these models to label data by assuming that the labels produced are near to ground truth. However, none investigated the correctness of this notion. Therefore, this paper fills this gap. Bad labels result in bad predictions, hence hypothetically, by positioning a human in the computing loop to correct inaccurate labels accuracy performance can be improved. As it is infeasible to expect a human to correct a multitude of labels, we set out to answer the questions of "What is the smallest percentage of corrected labels needed to improve prediction quality against a baseline? " and "Would randomly selecting automatic labels for correction produce better prediction than specifically choosing labels with distinct data points? ". Naive Bayes (NB) and Decision Tree (DT) were employed on AirBnB and Vaccines public datasets. We could conclude from our results that not all ML algorithms are suited to be used in a HITL environment. NB fared better than DT at producing improved accuracy with small percentages of corrected labels, as low as 1%, exceeding the baseline. When selected for human correction, labels with distinct data points assisted in enhancing the accuracy better than random selection for NB across both datasets, yet partially for DT.
更多查看译文
关键词
Human-in-the-loop, few labels, sentiment polarization
AI 理解论文
溯源树
样例
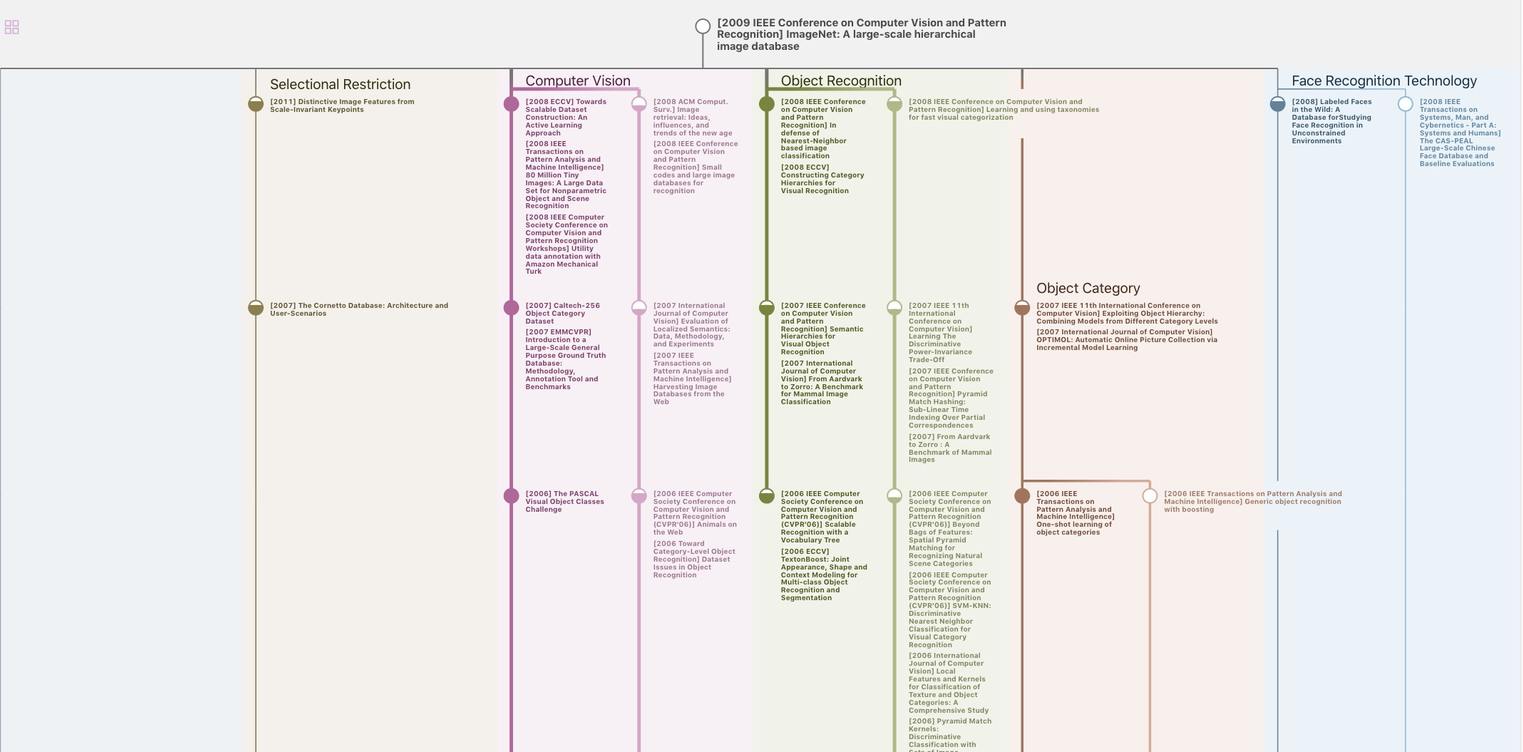
生成溯源树,研究论文发展脉络
Chat Paper
正在生成论文摘要