FCT: fusing CNN and transformer for scene classification
INTERNATIONAL JOURNAL OF MULTIMEDIA INFORMATION RETRIEVAL(2022)
摘要
Scene classification based on convolutional neural networks (CNNs) has achieved great success in recent years. In CNNs, the convolution operation performs well in extracting local features, but its ability to capture global feature representations is limited. In vision transformer (ViT), the self-attention mechanism can capture long-term feature dependencies, but it breaks down the details of local features. In this work, we make full use of the advantages of the CNN and ViT and propose a Transformer-based framework that combines CNN to improve the discriminative ability of features for scene classification. Specifically, we take the deep convolutional feature as the input and establish the scene Transformer module to extract the global feature in the scene image. An end-to-end scene classification framework called the FCT is built by fusing the CNN and scene Transformer module. Experimental results show that our FCT achieves a new state-of-the-art performance on two standard benchmarks MIT Indoor 67 and SUN 397, with the accuracy of 90.75% and 77.50%, respectively.
更多查看译文
关键词
Scene classification, Convolutional neural networks, Vision transformer, Deep learning
AI 理解论文
溯源树
样例
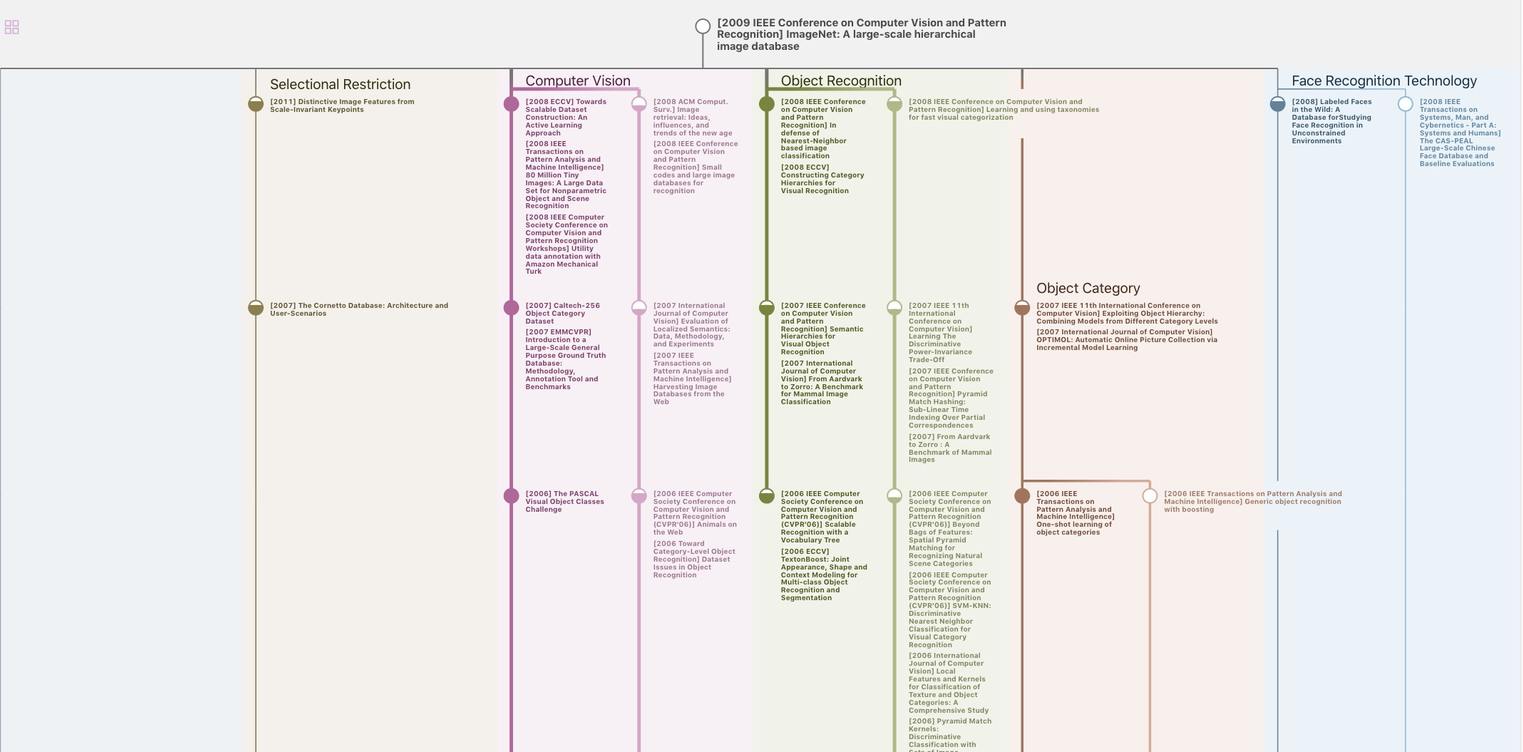
生成溯源树,研究论文发展脉络
Chat Paper
正在生成论文摘要