Foreformer: an enhanced transformer-based framework for multivariate time series forecasting
Applied Intelligence(2022)
摘要
Multivariate time series forecasting (MTSF) has been extensively studied throughout years with ubiquitous applications in finance, traffic, environment, etc. Recent investigations have demonstrated the potential of Transformer to improve the forecasting performance. Transformer, however, has limitations that prohibit it from being directly applied to MTSF, such as insufficient extraction of temporal patterns at different time scales, extraction of irrelevant information in the self-attention, and no targeted processing of static covariates. Motivated by above, an enhanced Transformer-based framework for MTSF is proposed, named Foreformer, with three distinctive characteristics: (i) a multi-temporal resolution module that deeply captures temporal patterns at different scales, (ii) an explicit sparse attention mechanism forces model to prioritize the most contributive components, and (iii) a static covariates processing module for nonlinear processing of static covariates. Extensive experiments on three real-world datasets demonstrate that Foreformer outperforms existing methodologies, making it a reliable approach for MTSF tasks.
更多查看译文
关键词
Multivariate time series forecasting,Attention mechanism,Deep learning,Multi-resolution,Static covariate,Transformer
AI 理解论文
溯源树
样例
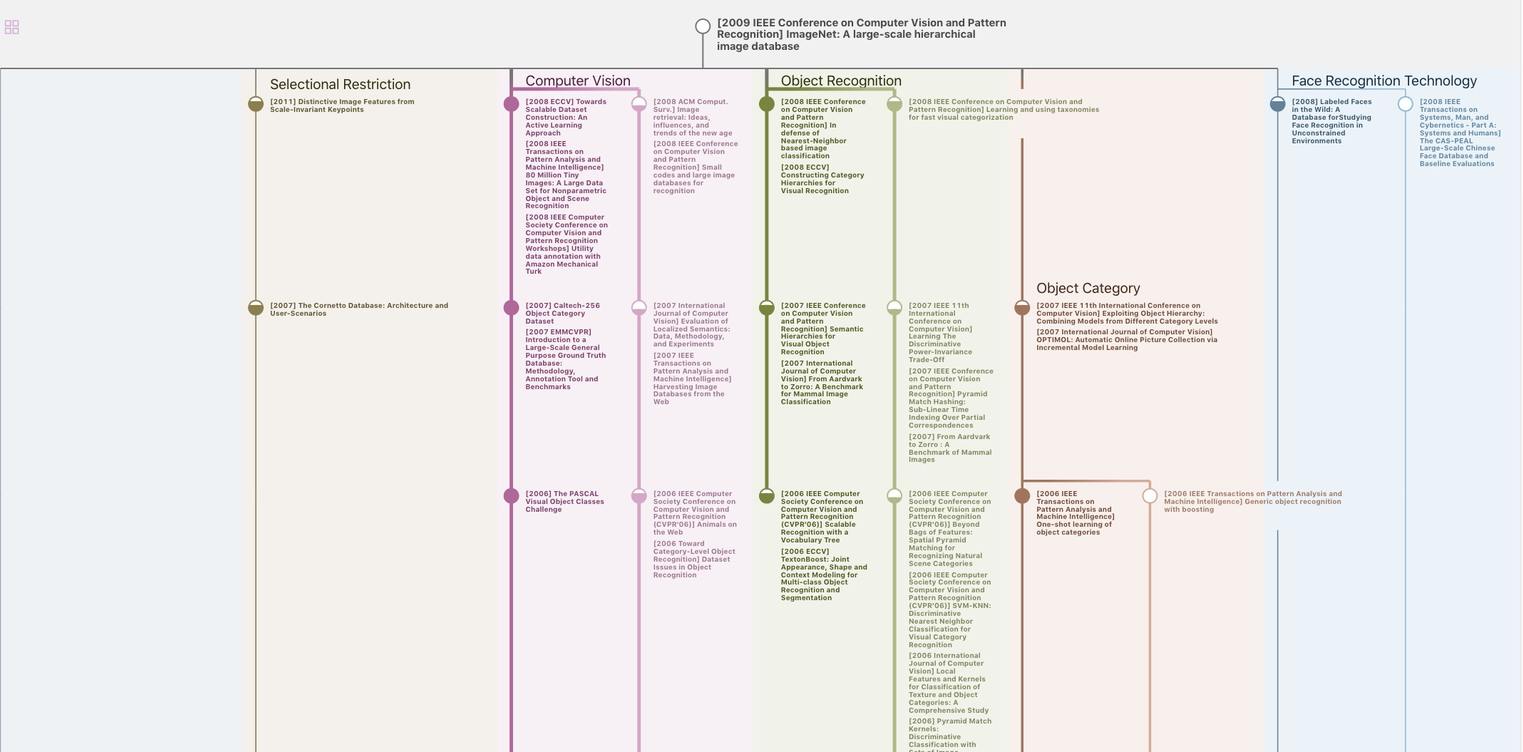
生成溯源树,研究论文发展脉络
Chat Paper
正在生成论文摘要