Arbitrary surface data patching method based on geometric convolutional neural network
NEURAL COMPUTING & APPLICATIONS(2022)
摘要
In free-form surface reconstruction technology, the ultimate goal is to obtain a computer model of a free-form surface. This study discusses the inpainting method of arbitrary surface data based on geometric convolutional neural networks. Reverse engineering is a process of product design technology reproduction, that is, reverse analysis and research of a target product, to deduce and obtain design elements such as the processing flow, organizational structure, functional characteristics and technical specifications of the product to produce functional similar but not identical products. This paper realizes the noise point elimination of point cloud data by studying reconstruction technology of free-form surfaces using a geometric convolutional neural network model. Point cloud data without gross errors are provided for later reconstruction. High-precision arbitrary surface data are realized for surface reconstruction. The repair of arbitrary surface data is completed based on BP and RBF neural networks, and a certain degree of data supplementing for the incomplete point cloud data measured is realized. Finally, free-form surface reconstruction is realized. In reverse engineering, a flexible surface is converted into a rigid surface, and the data points are collected using contact measuring equipment. The influencing factors of reverse engineering are work efficiency, technical ability and the influencing factors of innovative design. The data points obtained in this study are processed by denoising, streamlining and filtering. The sorted data points are used to obtain an optimized mathematical model after curve and surface fitting and smoothing. The research in this study proves that the data points obtained using the reverse engineering method can be very well applied in subsequent work. Therefore, this data collection method can be applied to other instances. In the experiment, the maximum error between the output of the RBF network and the test data is 0.0086 when surface 2 is repaired. When the hybrid learning algorithm is trained with different sample sets, the average width is 0.24, and the number of iterations is 1000. The experimental results of repairing various surface defect data show that the data repairing method has good versatility, fast data repairing speed and high precision, so it has high practical value.
更多查看译文
关键词
Geometric convolutional,Neural network,Arbitrary surface,Data inpainting
AI 理解论文
溯源树
样例
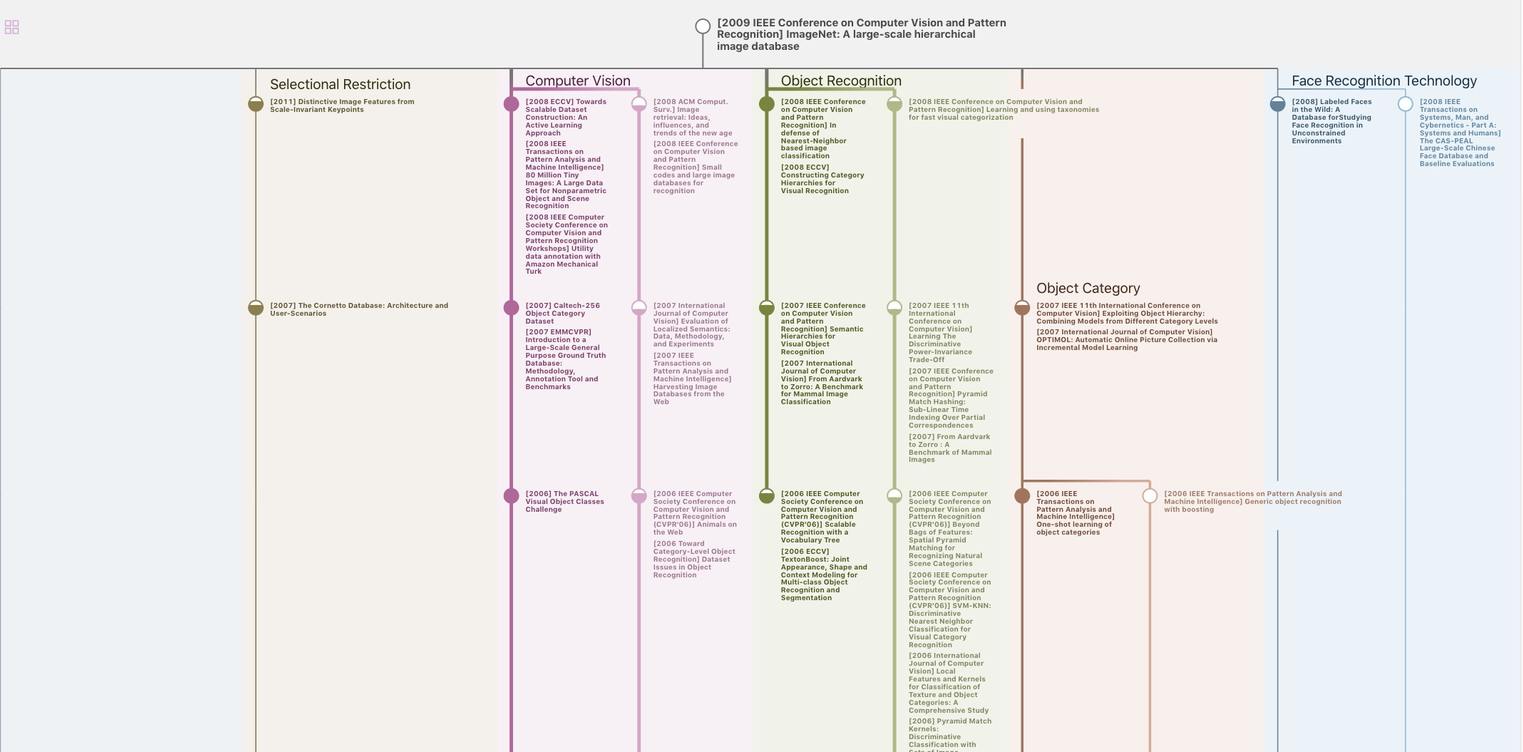
生成溯源树,研究论文发展脉络
Chat Paper
正在生成论文摘要