Exploring System and Machine Learning Performance Interactions when Tuning Distributed Data Stream Applications
2022 IEEE 38th International Conference on Data Engineering Workshops (ICDEW)(2022)
摘要
Deploying machine learning (ML) applications over distributed stream processing engines (DSPEs) such as Apache Spark Streaming is a complex procedure that requires extensive tuning along two dimensions. First, DSPEs have a vast array of system configuration parameters (such as degree of parallelism, memory buffer sizes, etc.) that need to be optimized to achieve the desired levels of latency and/or throughput. Second, each ML model has its own set of hyper-parameters that need to be tuned as they significantly impact the overall prediction accuracy of the trained model. These two forms of tuning have been studied extensively in the literature but only in isolation from each other. This position paper identifies the necessity for a combined system and ML model tuning approach based on a thorough experimental study. In particular, experimental results have revealed unexpected and complex interactions between the choices of system configuration and hyper-parameters, and their impact on both application and model performance. These findings open up new research directions in the field of self-managing stream processing systems.
更多查看译文
关键词
stream processing,machine learning,system parameter tuning,hyper-parameter tuning
AI 理解论文
溯源树
样例
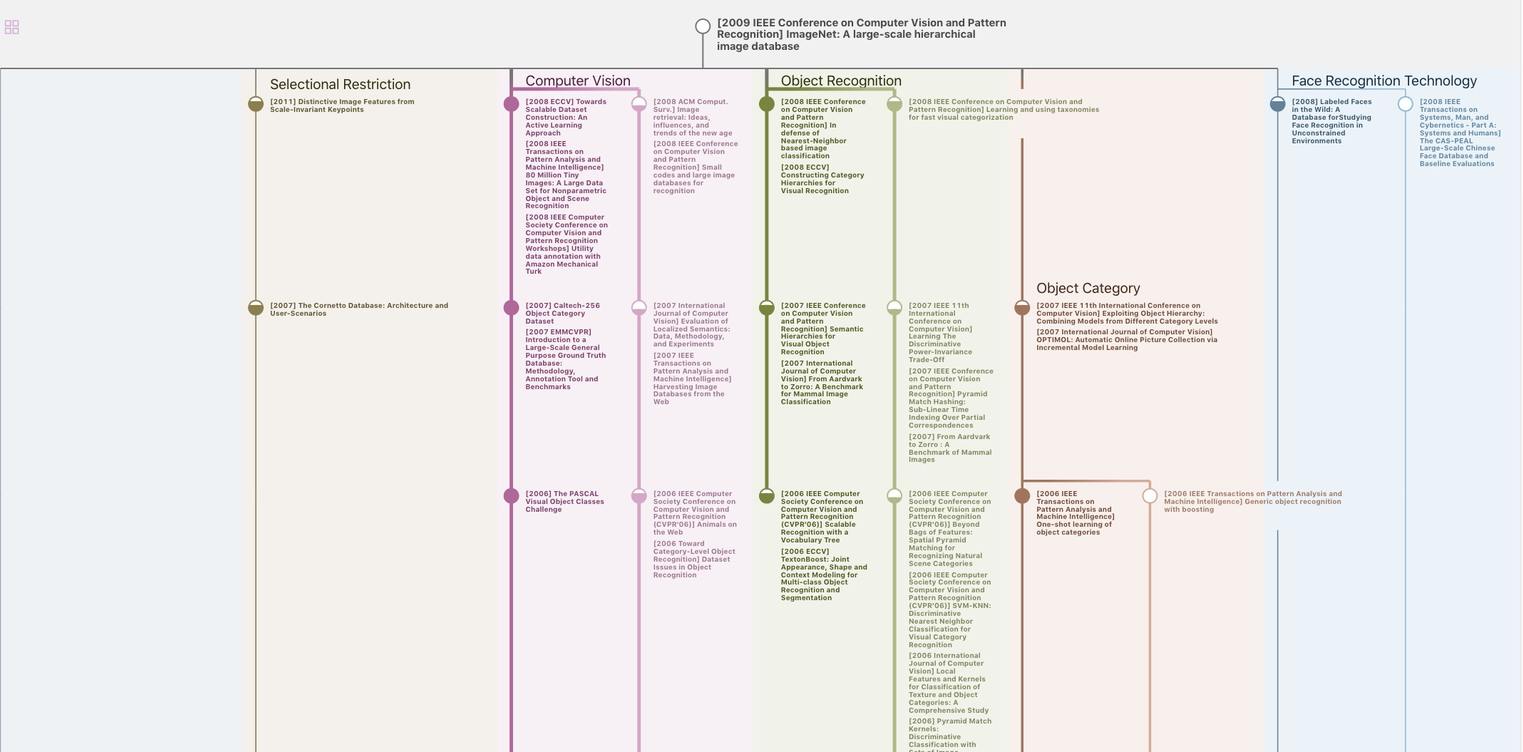
生成溯源树,研究论文发展脉络
Chat Paper
正在生成论文摘要