UIFGAN: An unsupervised continual-learning generative adversarial network for unified image fusion
Information Fusion(2022)
摘要
In this paper, we propose a novel unsupervised continual-learning generative adversarial network for unified image fusion, termed as UIFGAN. In our model, for multiple image fusion tasks, a generative adversarial network for training a single model with memory in a continual-learning manner is proposed, rather than training an individual model for each fusion task or jointly training multiple tasks. We use elastic weight consolidation to avoid forgetting what has been learned from previous tasks when training multiple tasks sequentially. In each task, the generation of the fused image comes from the adversarial learning between a generator and a discriminator. Meanwhile, a max-gradient loss function is adopted for forcing the fused image to obtain richer texture details of the corresponding regions in two source images, which applies to most typical image fusion tasks. Extensive experiments on multi-exposure, multi-modal and multi-focus image fusion tasks demonstrate the advantages of our method over the state-of-the-art approaches.
更多查看译文
关键词
Image fusion,Continual-learning,Generative adversarial network,Max-gradient loss,Unified model
AI 理解论文
溯源树
样例
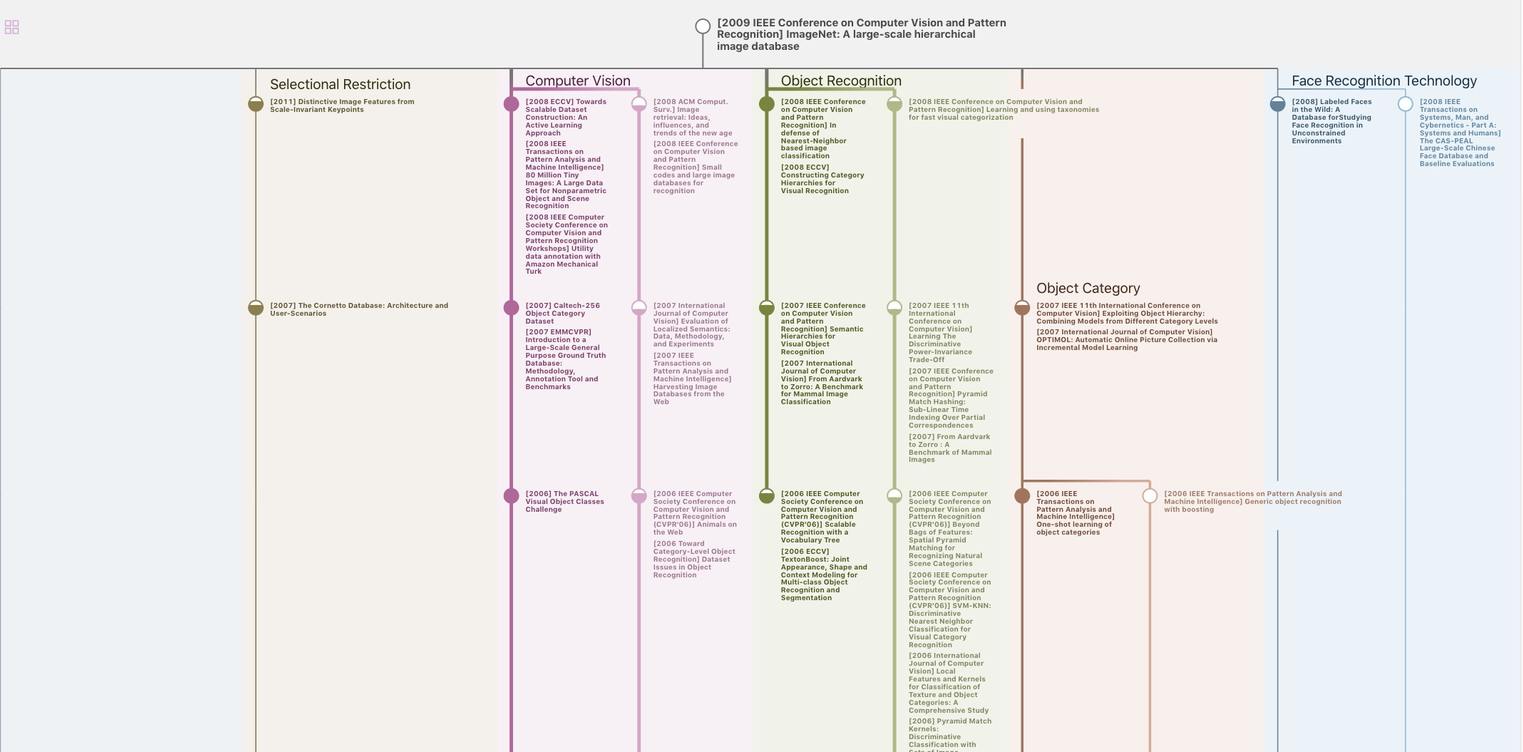
生成溯源树,研究论文发展脉络
Chat Paper
正在生成论文摘要