ERBM-SE: Extended Restricted Boltzmann Machine for Multi-Objective Single-Channel Speech Enhancement
INTERNATIONAL JOURNAL OF INTERACTIVE MULTIMEDIA AND ARTIFICIAL INTELLIGENCE(2022)
摘要
Machine learning-based supervised single-channel speech enhancement has achieved considerable research interest over conventional approaches. In this paper, an extended Restricted Boltzmann Machine (RBM) is proposed for the spectral masking-based noisy speech enhancement. In conventional RBM, the acoustic features for the speech enhancement task are layerwise extracted and the feature compression may result in loss of vital information during the network training. In order to exploit the important information in the raw data, an extended RBM is proposed for the acoustic feature representation and speech enhancement. In the proposed RBM, the acoustic features are progressively extracted by multiple-stacked RBMs during the pre-training phase. The hidden acoustic features from the previous RBM are combined with the raw input data that serve as the new inputs to the present RBM. By adding the raw data to RBMs, the layer-wise features related to the raw data are progressively extracted, that is helpful to mine valuable information in the raw data. The results using the TIMIT database showed that the proposed method successfully attenuated the noise and gained improvements in the speech quality and intelligibility. The STOI, PESQ and SDR are improved by 16.86%, 25.01% and 3.84dB over the unprocessed noisy speech.
更多查看译文
关键词
Restricted Boltzmann Machine, Spectral Masking, Speech Enhancement, Speech Intelligibility, Speech Quality, Supervised Machine Learning
AI 理解论文
溯源树
样例
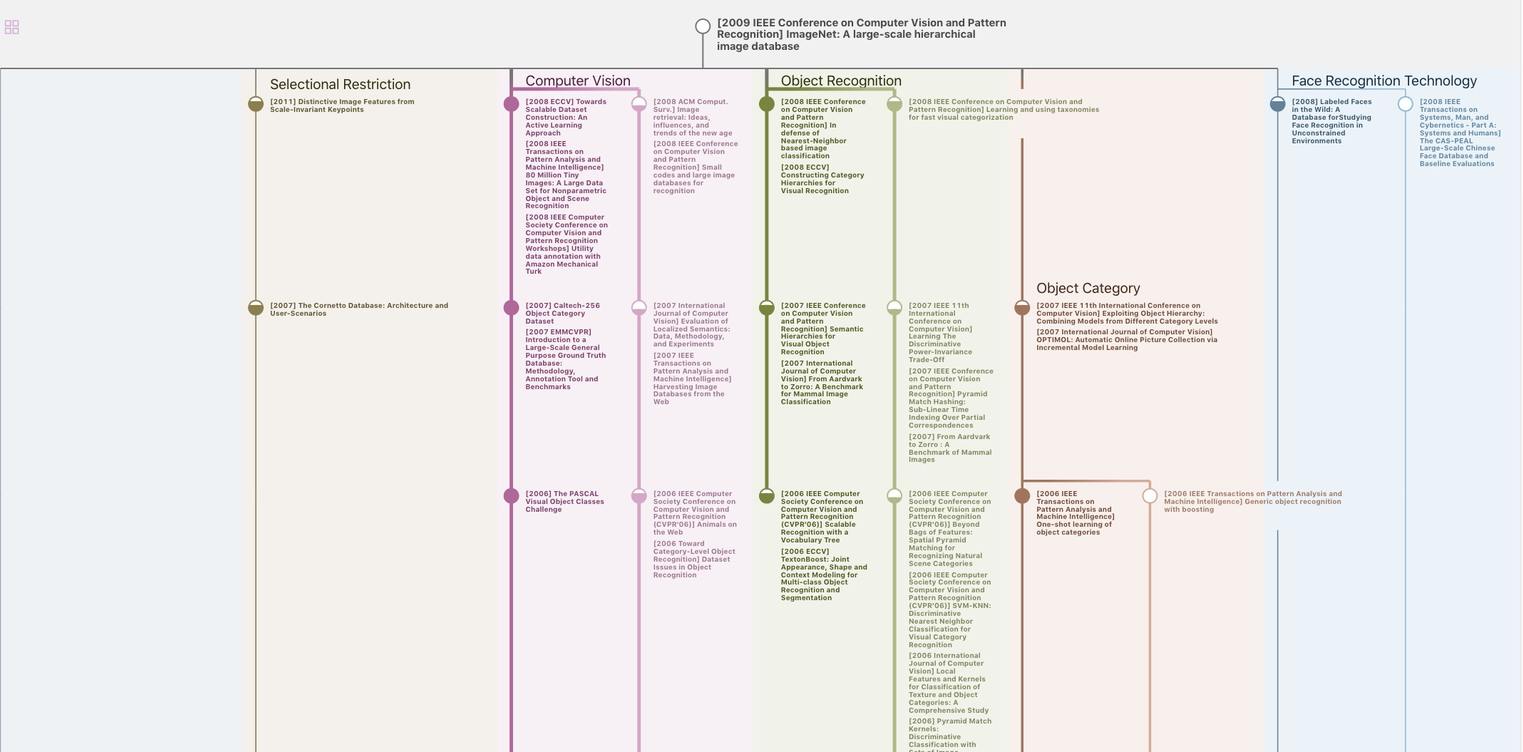
生成溯源树,研究论文发展脉络
Chat Paper
正在生成论文摘要