Energy-aware neural architecture selection and hyperparameter optimization
2022 IEEE 36TH INTERNATIONAL PARALLEL AND DISTRIBUTED PROCESSING SYMPOSIUM WORKSHOPS (IPDPSW 2022)(2022)
摘要
Artificial Intelligence (AI) and Deep Learning in particular have increasing computational requirements, with a corresponding increase in energy consumption. There is a tremendous opportunity to reduce the computational cost and environmental impact of deep learning by accelerating neural network architecture search and hyperparameter optimization, as well as explicitly designing neural architectures that optimize for both energy efficiency and performance. Here, we introduce a framework called training performance estimation (TPE), which builds upon existing techniques for training speed estimation in order to monitor energy consumption and rank model performance-without training models to convergence-saving up to 90% of time and energy of the full training budget. We benchmark TPE in the computationally intensive, well-studied domain of computer vision and in the emerging field of graph neural networks for machine-learned inter-atomic potentials, an important domain for scientific discovery with heavy computational demands. We propose variants of early stopping that generalize this common regularization technique to account for energy costs and study the energy costs of deploying increasingly complex, knowledge-informed architectures for AI-accelerated molecular dynamics and image classification. Our work enables immediate, significant energy savings across the entire pipeline of model development and deployment and suggests new research directions for energy-aware, knowledge-informed model architecture development.
更多查看译文
关键词
deep learning, green AI, sustainable AI, energy efficiency, hyperparameter optimization, early stopping, graph neural networks, computer vision
AI 理解论文
溯源树
样例
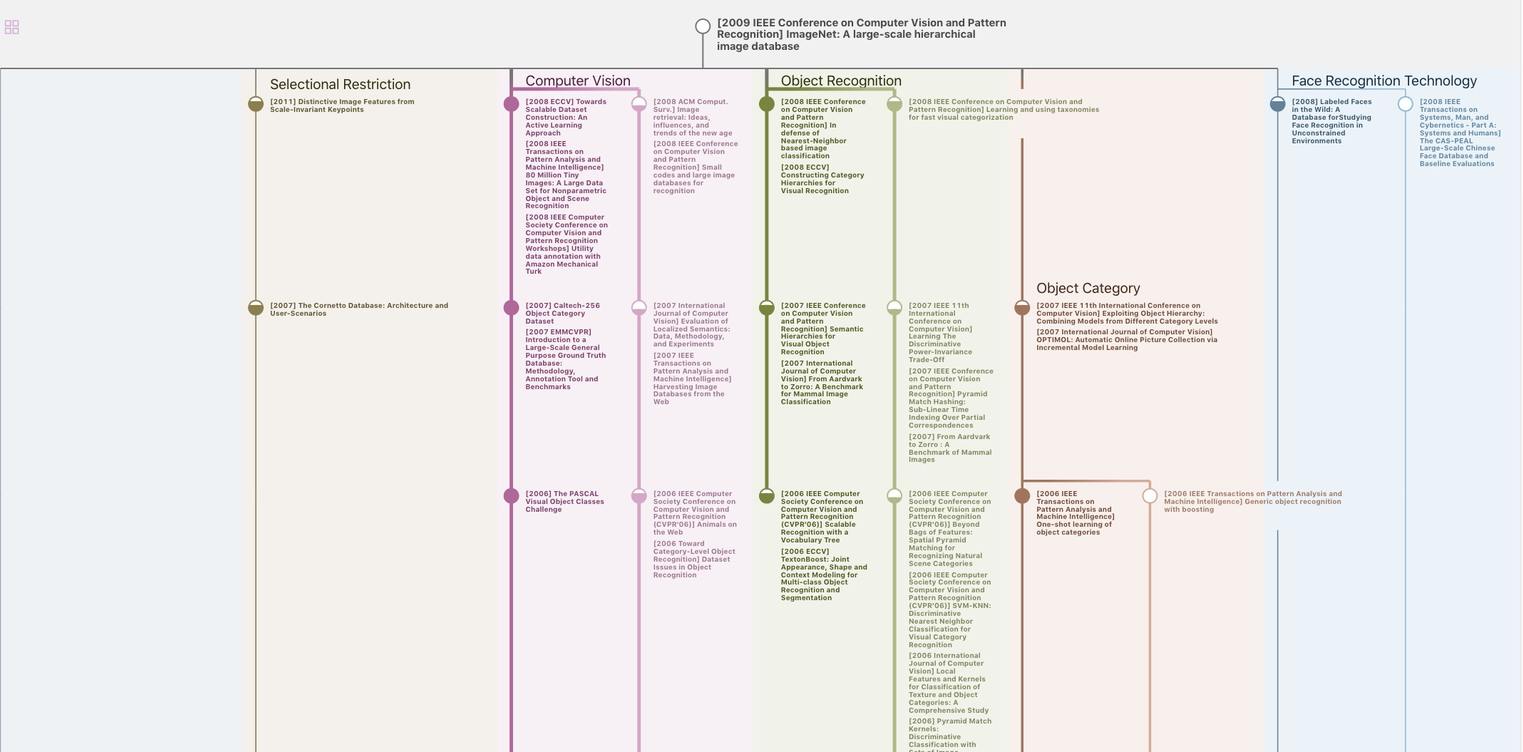
生成溯源树,研究论文发展脉络
Chat Paper
正在生成论文摘要