Continuous Model Evaluation and Adaptation to Distribution Shifts: A Probabilistic Self-Supervised Approach
2022 25TH INTERNATIONAL CONFERENCE ON INFORMATION FUSION (FUSION 2022)(2022)
摘要
This paper introduces a Bayesian approach to estimating distribution shifts over the modelled variables and continuous model adaptations to mitigate the impact of such shifts. The method exploits probabilistic inference over sets of correlated variables in causal models describing data generating processes. By extending the models with latent auxiliary variables, probabilistic inference over sets of correlated variables enables estimation of the distribution shifts impacting different parts of the models. Moreover, the introduction of latent auxiliary variables makes inference more robust against distribution shifts and supports automated, self-supervised adaptation of the modelling parameters during the operation, often significantly reducing the adverse impact of the distribution shifts. The effectiveness of the method has been validated in systematic experiments using synthetic data.
更多查看译文
关键词
distribution shift, inference, Bayesian Networks, machine learning, trust
AI 理解论文
溯源树
样例
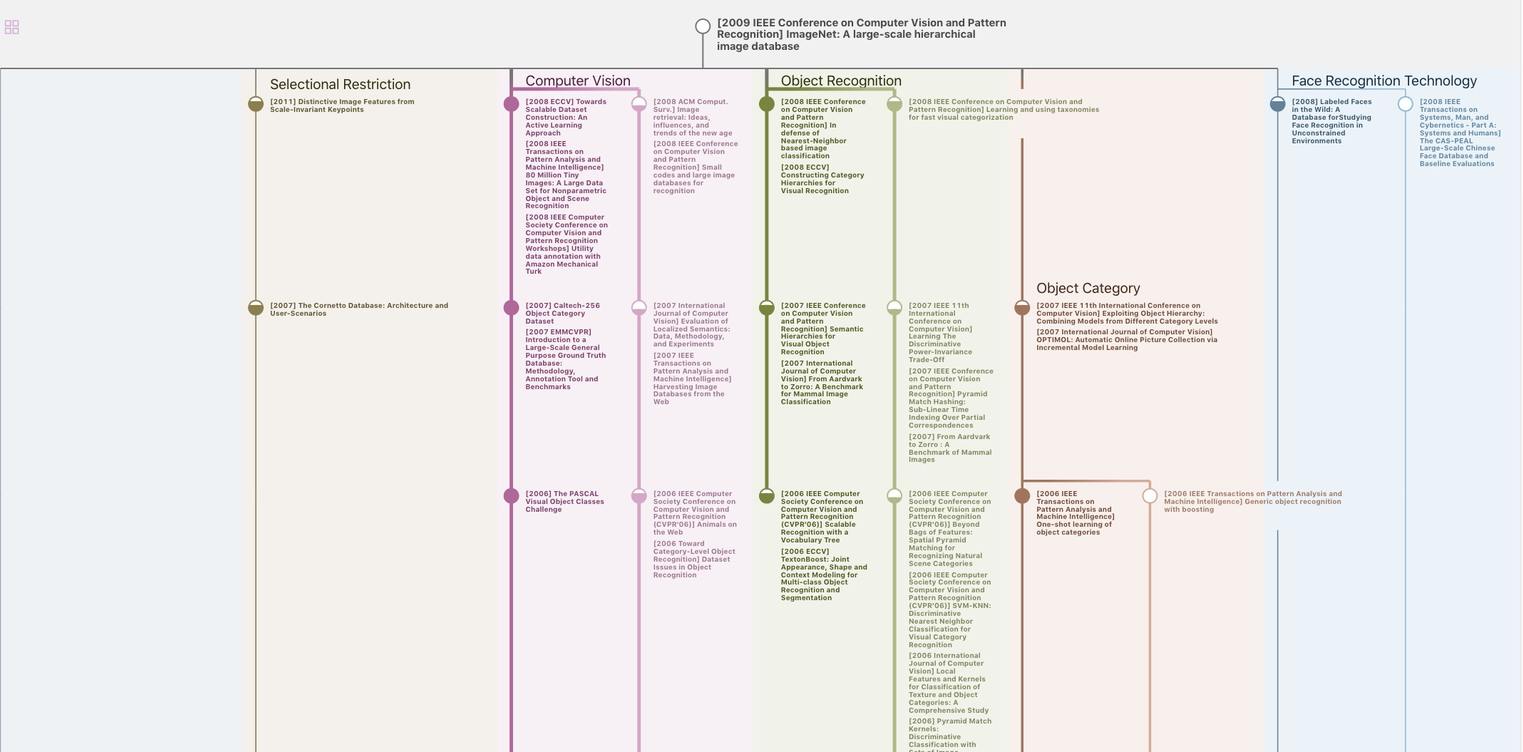
生成溯源树,研究论文发展脉络
Chat Paper
正在生成论文摘要