Enhanced Recurrent Neural Network for Fault Diagnosis of Uncertain Wind Energy Conversion Systems*
2022 8TH INTERNATIONAL CONFERENCE ON CONTROL, DECISION AND INFORMATION TECHNOLOGIES (CODIT'22)(2022)
摘要
In this paper, new fault detection and diagnosis (FDD) techniques dealing with uncertainties in wind energy conversion (WEC) systems are proposed. The uncertainty is addressed by using the intervalvalued data representation. The main contributions are twofold: first, to simplify the Recurrent Neural Network (RNN) model in terms of training and computation time and storage cost as well, a reduced version of RNN is proposed. Reduced RNN is established on the H-K-means algorithms to treat the correlations between samples and extract a reduced number of observations from the training data matrix. The main idea behind using H-K-means algorithms for dataset size reduction is to simplify the RNN model in terms of training and computation time. Second, two reduced RNN-based interval-valued data techniques are proposed to distinguish between the different WEC system operating modes. Therefore, two reduced RNN-based interval centers and ranges and interval upper and lower bounds techniques are proposed to deal with the WEC system uncertainties. The presented results confirm the high feasibility and effectiveness of the proposed FDD techniques.
更多查看译文
关键词
recurrent neural network,wind energy conversion systems,fault detection and diagnosis techniques,computation time,storage cost,training data matrix,dataset size reduction,lower bounds techniques,FDD techniques,WEC system operating modes,RNN-based interval-valued data techniques,H-K-means algorithms,interval upper bounds techniques
AI 理解论文
溯源树
样例
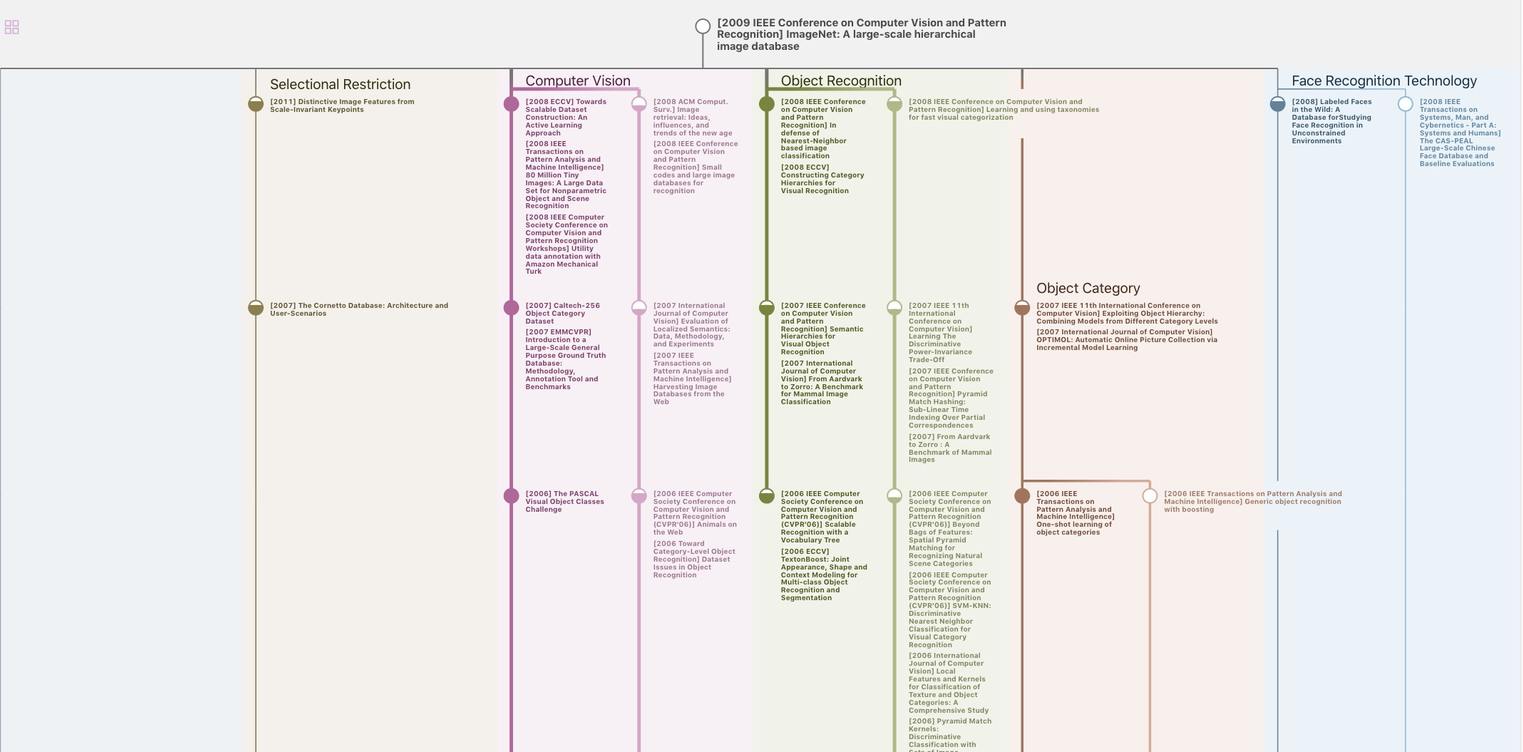
生成溯源树,研究论文发展脉络
Chat Paper
正在生成论文摘要