Improved Ensemble Approach for Fault Diagnosis of Wind Energy Conversion Systems*
2022 8TH INTERNATIONAL CONFERENCE ON CONTROL, DECISION AND INFORMATION TECHNOLOGIES (CODIT'22)(2022)
摘要
Safe production is of great significance in the process industry like the wind energy conversion (WEC) systems. An unexpected fault in part of the WEC system can damage the entire mechanical system, resulting in huge economic losses and even catastrophic failures. Therefore, this paper proposes an effective neural networks-based ensemble approach for fault detection and diagnosis (FDD) of WEC systems. The main contributions are twofold: first, an ensemble learning technique based on the combination of different neural network (ANN, CFNN, and GFNN) into one optimal model are developed in order to distinguish between the different WEC systems operating modes. Then, in order to enhance the results in terms of computation time and storage cost, a reduced version of the proposed neural network-based ensemble technique is presented. The main idea behind this proposal is to use the Hierarchical K-means (H-K-means) clustering to extract only the most significant samples from raw data. Then, the reduced data are introduced as inputs to the proposed neural network-based ensemble technique method to deal with the problem of fault classification. The experimental results demonstrated the feasibility and effectiveness of the proposed FDD techniques.
更多查看译文
关键词
process industry,safe production,wind energy conversion systems,fault diagnosis,FDD techniques,fault classification,neural network-based ensemble technique method,storage cost,computation time,different WEC systems operating modes,different neural network,ensemble learning technique,fault de-tection,effective neural networks-based ensemble approach,catastrophic failures,huge economic losses,entire mechanical system,WEC system,unexpected fault
AI 理解论文
溯源树
样例
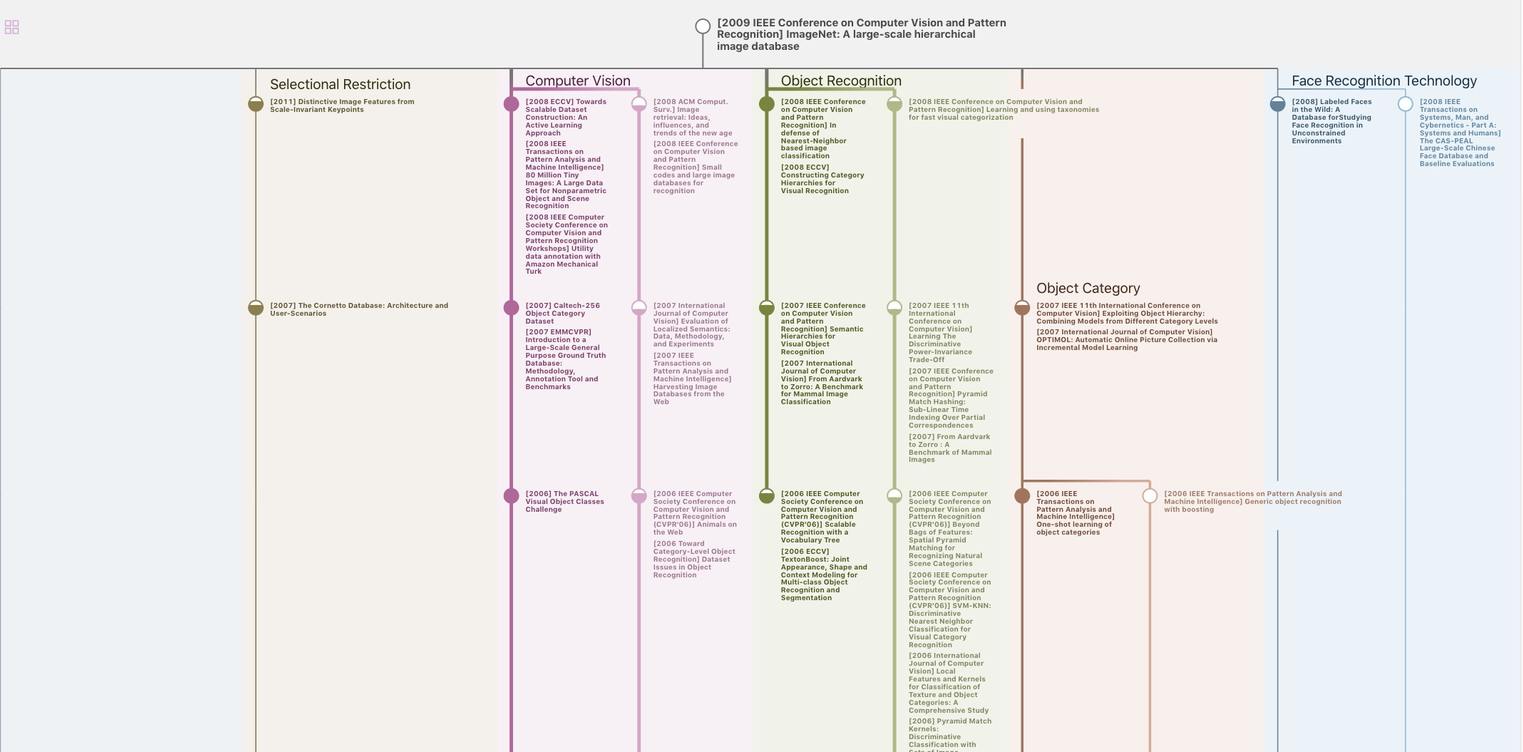
生成溯源树,研究论文发展脉络
Chat Paper
正在生成论文摘要