Predicting Tunneling-Induced Ground Collapse Based on TBM Operational Data and Geological Data
INTERNATIONAL JOURNAL OF COMPUTATIONAL METHODS(2022)
摘要
Collapses are the most sensational types of events and frequently the ones that cause the most serious consequences in tunneling operations. It often occurs because of insufficient geological studies and the limitations of experience-based decision-making. To cope with those problems, we proposed a data-driven model based on the tunnel boring machine operational data and the in situ geological information to forecast tunneling-induced ground collapse. In the proposed model, we offered a general data process flow diagram to process engineering data. Three machine learning classifiers, k-nearest neighbors, support vector classifier, and random forests were adopted for collapse prediction. The performance of the three classifiers was verified based on the data from the Yinsong water conveyance tunnel. The results illustrated that the proposed data-driven model was sufficient for the studying task with 90% of the collapsed zones being identified on average. The contributions of this paper are to provide a reliable data process flow diagram to process engineering data and offer an accurate and robust model for identifying collapses.
更多查看译文
关键词
Tunnel boring machine, ground collapse, TBM operational data, machine learning classifier
AI 理解论文
溯源树
样例
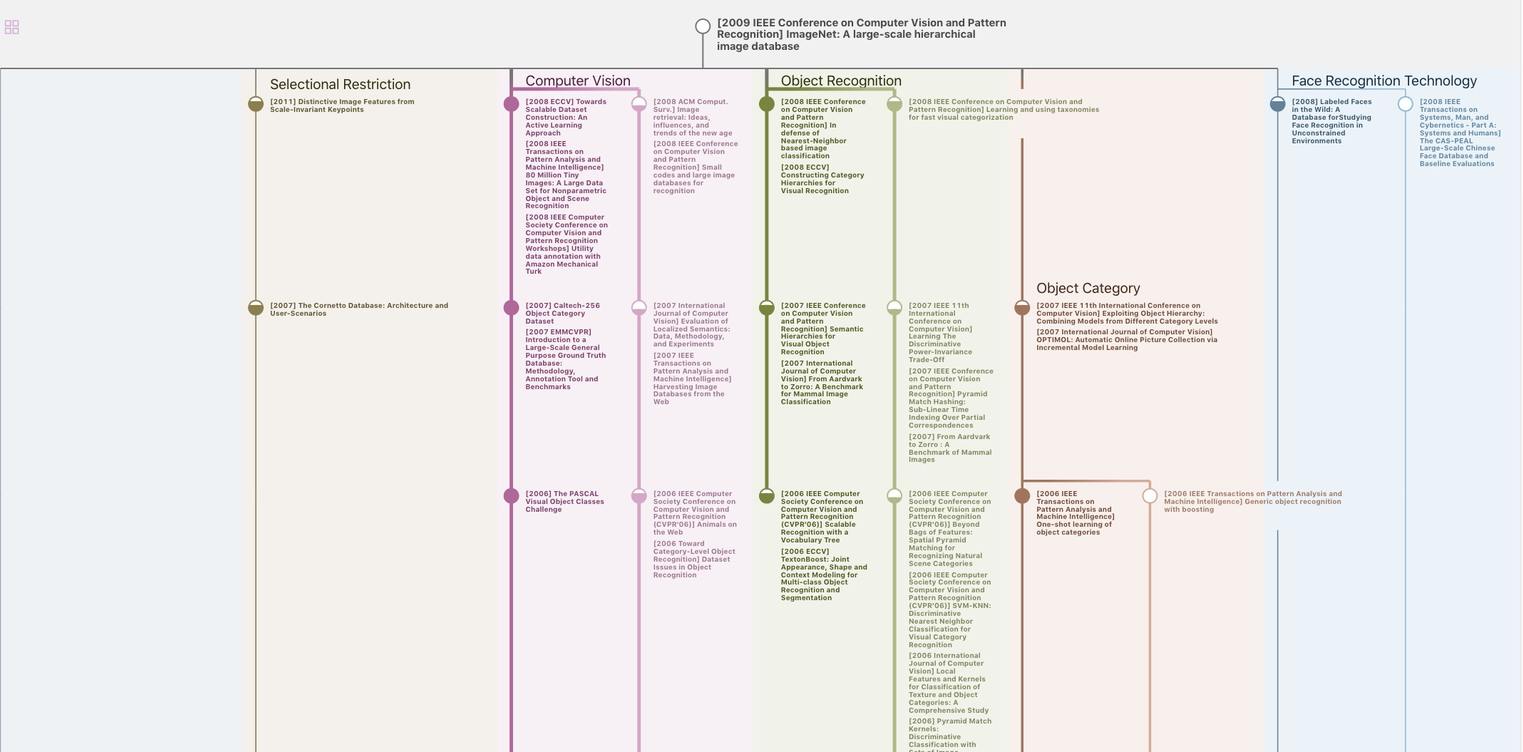
生成溯源树,研究论文发展脉络
Chat Paper
正在生成论文摘要