A Compressed Model-Agnostic Meta-Learning Model Based on Pruning for Disease Diagnosis
JOURNAL OF CIRCUITS SYSTEMS AND COMPUTERS(2023)
摘要
Meta-learning has been widely used in medical image analysis. However, it requires a large amount of storage space and computing resources to train and use neural networks, especially model-agnostic meta-learning (MAML) models, making networks difficult to deploy on embedded systems and low-power devices for smart healthcare. Aiming at this problem, we explore to compress a MAML model with pruning methods for disease diagnosis. First, for each task, we find unimportant and redundant connections in MAML for its classification, respectively. Next, we find common unimportant connections for most tasks with intersections. Finally, we prune the common unimportant connections of the initial network. We conduct some experiments to assess the proposed model by comparison with MAML on Omniglot dataset and MiniImagenet dataset. The results show that our method reduces 40% parameters of the raw models, without incurring accuracy loss, demonstrating the potential of the proposed method for disease diagnosis.
更多查看译文
关键词
Model-agnostic meta-learning, pruning, disease diagnosis
AI 理解论文
溯源树
样例
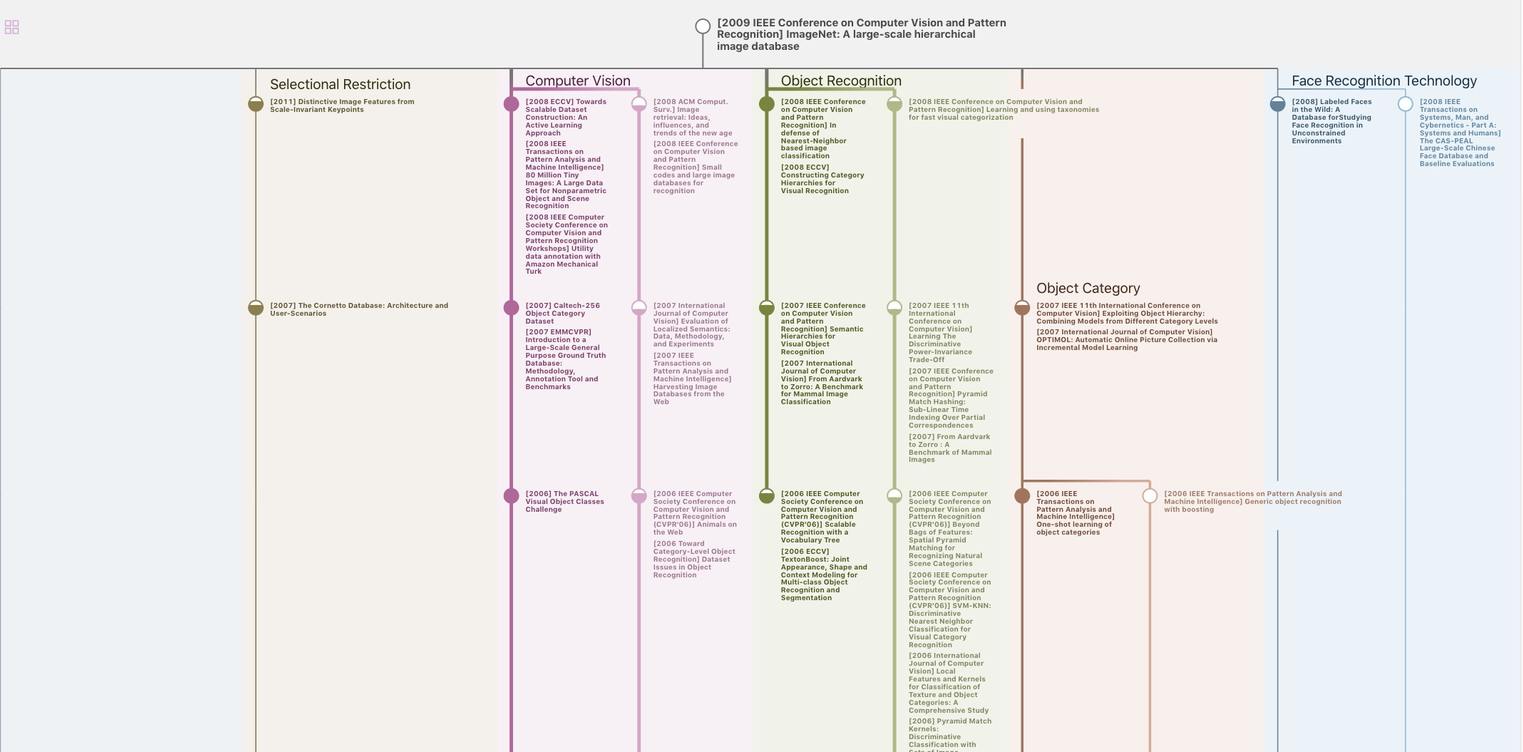
生成溯源树,研究论文发展脉络
Chat Paper
正在生成论文摘要