LiCAM: Long-Tailed Instance Segmentation with Real-Time Classification Accuracy Monitoring
JOURNAL OF CIRCUITS SYSTEMS AND COMPUTERS(2023)
摘要
Recently, deep neural networks have achieved remarkable progress in class balancing instance segmentation. However, most applications in the real world have a long-tailed distribution, i.e., limited training examples in the majority of classes. The long-tailed challenge leads to a catastrophic drop in instance segmentation because the gradient of the head classes suppresses the gradient of the tail classes, leading to a bias towards the major classes. We propose LiCAM, a novel framework for long-tailed segmentation. It features an adaptive loss function named Moac Loss, which is adjustable during the training according to the monitored classification accuracy. LiCAM also cooperates with an oversampling technique named RFS, which alleviates the severe imbalance between head and tail classes. We conducted extensive experiments on the LVIS v1 dataset to evaluate LiCAM. With a coherent end-to-end training pipeline, LiCAM significantly outperforms other baselines.
更多查看译文
关键词
Deep learning, instance segmentation, long-tailed distribution, adaptive, oversample
AI 理解论文
溯源树
样例
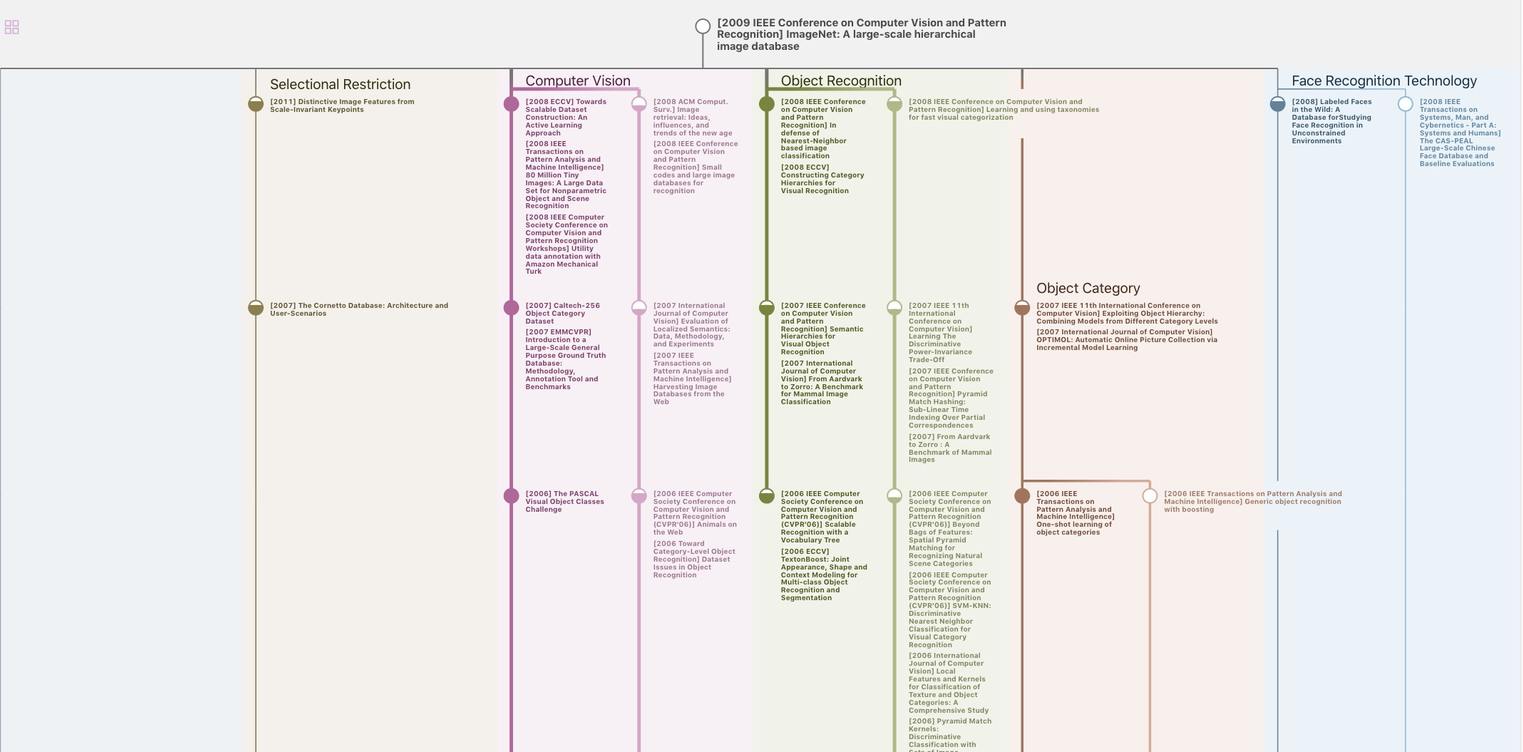
生成溯源树,研究论文发展脉络
Chat Paper
正在生成论文摘要