Modern hybrids - optimization using local search, population-based search, and machine learning
Genetic and Evolutionary Computation Conference(2022)
摘要
BSTRACTThis talk summarizes our 15+ years of work on the use of Machine Learning for Search & Optimization. I review the four main approaches that we invented during this time. Since learning during search takes effort, it should not surprise that we designed three of these approaches for a particular target range of total function evaluations: from few tens of dozens, to thousands, to many hundreds of thousands of function evaluations. The last hybrid I review regards a surrogate-based approach for optimization under stochastic uncertainty. The wonder of this research area is that each of these four methods defines the state of the art in its respective area, giving significant empirical evidence that learning to optimize can be highly effective.
更多查看译文
关键词
surrogate-assisted optimization, optimization under uncertainty, small budget optimization, large budget optimization
AI 理解论文
溯源树
样例
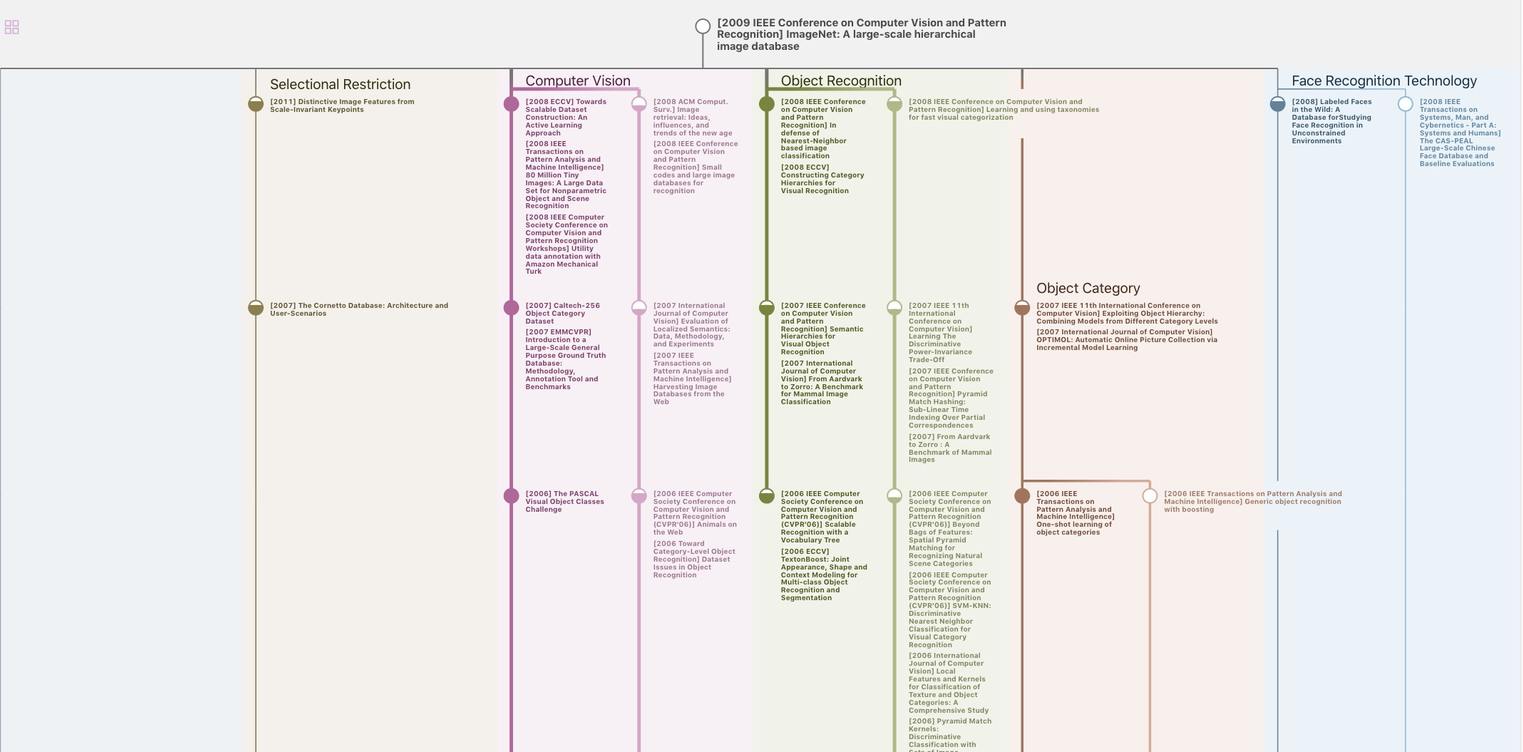
生成溯源树,研究论文发展脉络
Chat Paper
正在生成论文摘要