Identification of Anticancer and Anti-inflammatory Drugs from Drug-target Interaction Descriptors by Machine Learning
LETTERS IN DRUG DESIGN & DISCOVERY(2022)
摘要
Background: Drug repositioning is an important subject in drug-disease research. In the past, most studies simply used drug descriptors as the feature vector to classify drugs or targets or used qualitative data about drug-target or drug-disease to predict drug-target interactions. These data provide limited information for drug repositioning. Objective: Considering both drugs and targets and constructing quantitative drug-target interaction descriptors as a method of drug characteristics are of great significance to the study of drug repositioning. Methods: Taking anticancer and anti-inflammatory drugs as research objects, the interaction sites between drugs and targets were determined by molecular docking. Sixty-seven drug-target interaction descriptors were calculated to describe the drug-target interactions, and 22 important descriptors were screened for drug classification by SVM, LightGBM, and MLP. Results: The accuracy of SVM, LightGBM, and MLP reached 93.29%, 92.68%, and 94.51%, their Matthews correlation coefficients reached 0.852, 0.840, and 0.882, and their areas under the ROC curve reached 0.977, 0.969, and 0.968, respectively. Conclusion: Using drug-target interaction descriptors to build machine learning models can obtain better results for drug classification. Number of atom pairs, force field, hydrophobic interactions, and bSASA are the key features for classifying anticancer and anti-inflammatory drugs.
更多查看译文
关键词
Drug-target interaction, molecular docking, descriptors, SVM, LightGBM, MLP
AI 理解论文
溯源树
样例
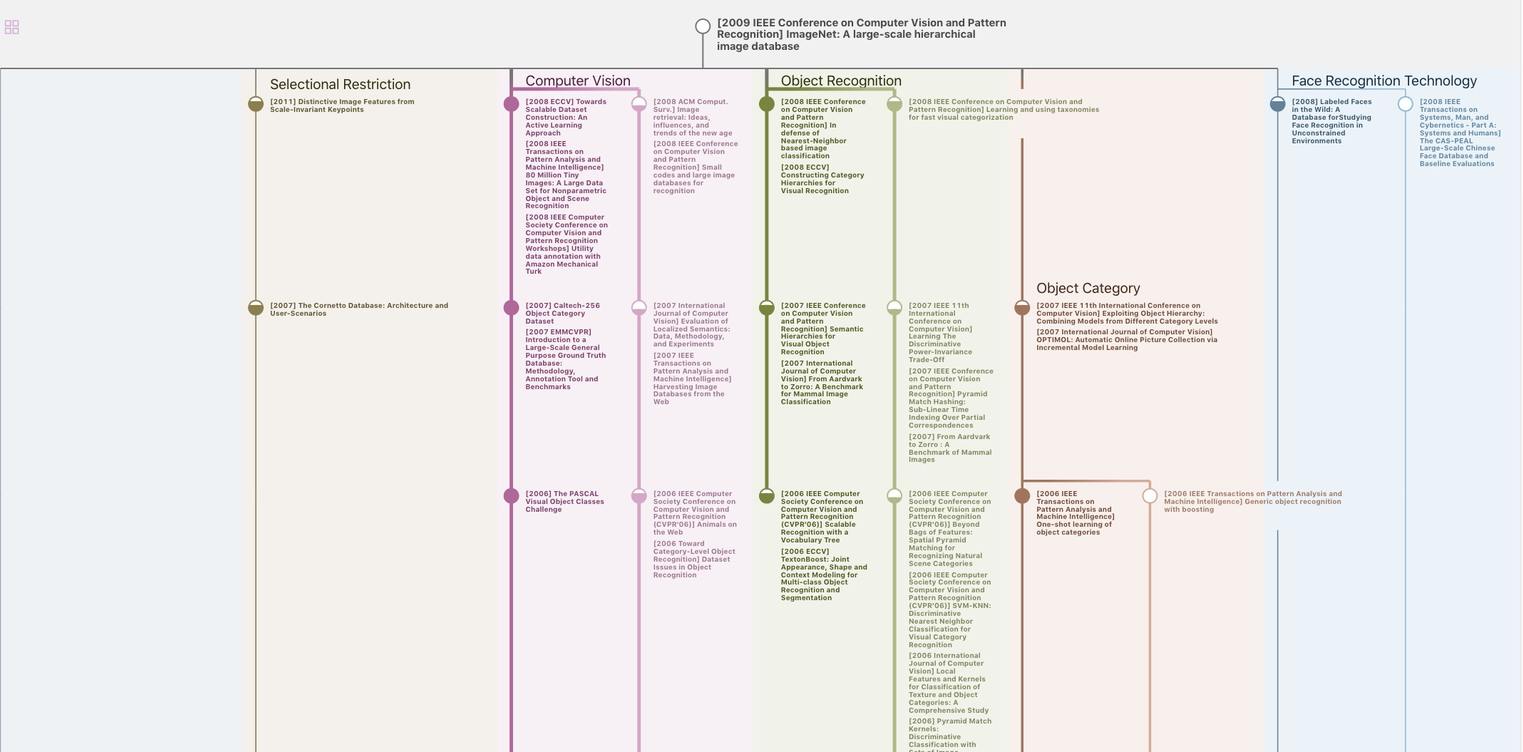
生成溯源树,研究论文发展脉络
Chat Paper
正在生成论文摘要