Regularized linear discriminant analysis based on generalized capped l_2,q -norm
ANNALS OF OPERATIONS RESEARCH(2022)
摘要
iming to improve the robustness and adaptiveness of the recently investigated capped norm linear discriminant analysis (CLDA), this paper proposes a regularized linear discriminant analysis based on the generalized capped l_2,q -norm (GCLDA). Compared to CLDA, there are two improvements in GCLDA. Firstly, GCLDA uses the capped l_2,q -norm rather than the capped l_2,1 -norm to measure the within-class and between-class distances for arbitrary q>0 . By selecting an appropriate q , GCLDA is adaptive to different data, and also removes extreme outliers and suppresses the effect of noise more effectively. Secondly, by taking into account a regularization term, GCLDA not only improves its generalization ability but also avoids singularity. GCLDA is solved through a series of generalized eigenvalue problems. Experiments on an artificial dataset, some real world datasets and a high-dimensional dataset demonstrate the effectiveness of GCLDA.
更多查看译文
关键词
Linear discriminant analysis,Capped norm,Generalized capped norm,Capped norm linear discriminant analysis,Dimensionality reduction
AI 理解论文
溯源树
样例
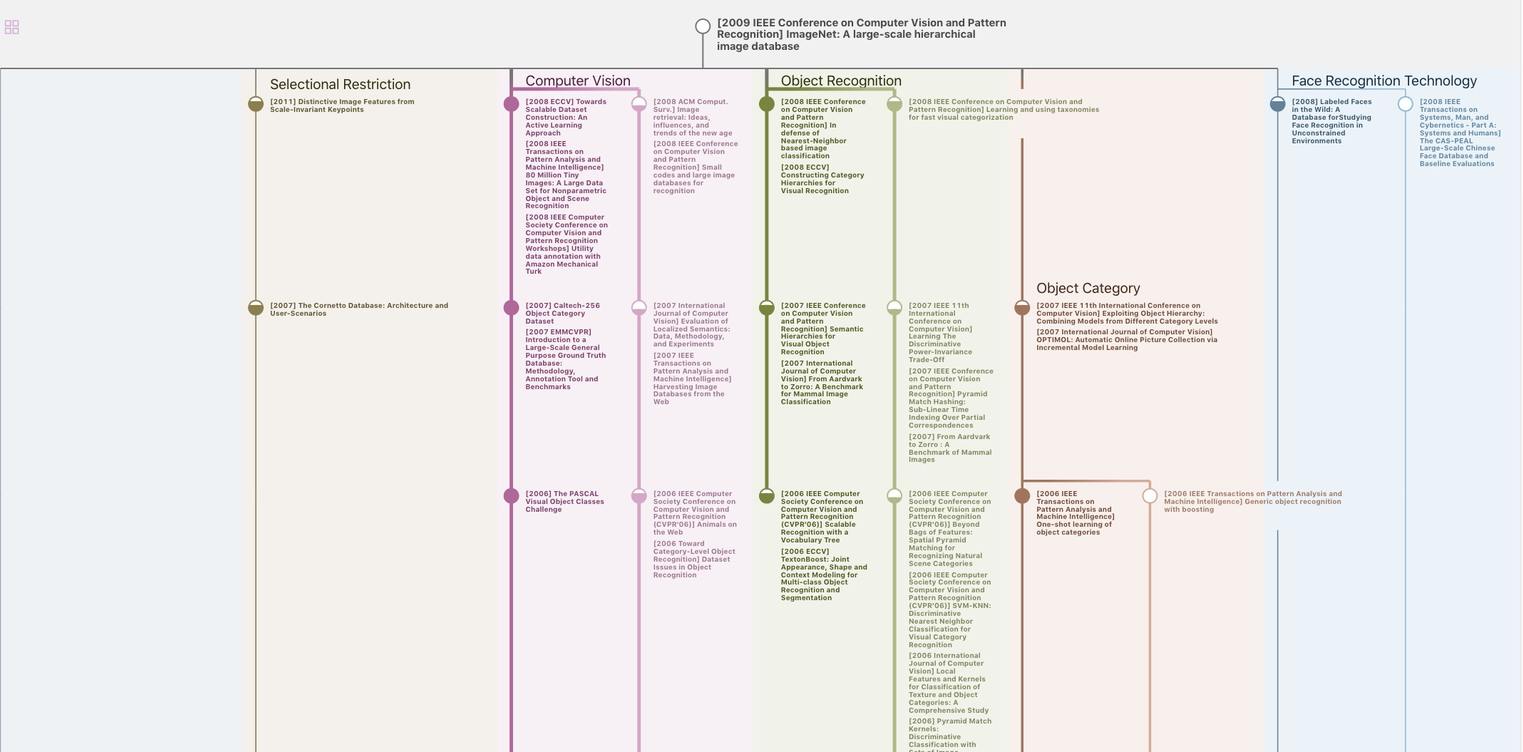
生成溯源树,研究论文发展脉络
Chat Paper
正在生成论文摘要