Non-contact surface roughness evaluation of milling surface using CNN-deep learning models
INTERNATIONAL JOURNAL OF COMPUTER INTEGRATED MANUFACTURING(2024)
摘要
Machining quality control is a bottleneck operation as human inspectors and expensive equipment is needed in most operations. Automated quality assurance in the manufacturing industry has the potential to replace humans and lower the cost of the machined product. This paper presents the analysis of end-milled machined surfaces backed with experimental and deep learning model investigations. The effects of machining parameters like spindle speed, feed rate (table feed), depth of cut, cutting speed, and machining duration were investigated to find machined surface roughness using Taguchi orthogonal array. Following standard DOE, surface roughness and machined surface image were recorded for each machining experiment and categorized into four classes, viz. fine, smooth, rough, and coarse, based on the roughness value (Ra). The machined surface images were used to develop CNN models for surface roughness class prediction. Further, comparative studies among the five popular optimizers were performed. The results showed that the CNN model with the 'Rectified Adam' optimizer performed better amongst the optimizer pool, with the training and test accuracy of 96.30% and 92.91%, respectively. The proposed CNN model features a highly accurate and slim structure, potentially substituting human quality control procedures that employ expensive surface roughness measuring devices.
更多查看译文
关键词
Deep learning,convolution neural network,Taguchi method,surface roughness,non-contact classification,end milling
AI 理解论文
溯源树
样例
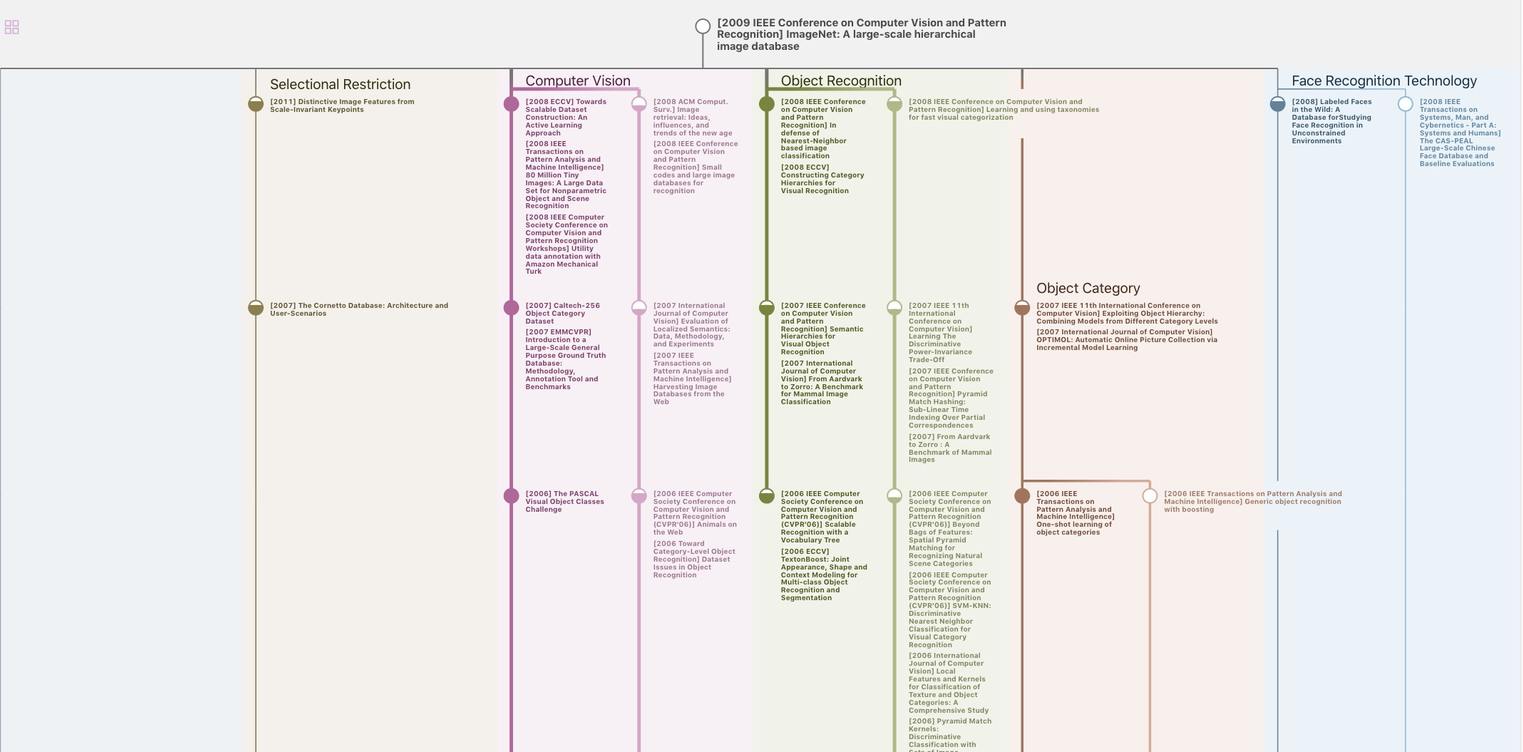
生成溯源树,研究论文发展脉络
Chat Paper
正在生成论文摘要