SPECTRAL-SPATIAL MULTISCALE RESIDUAL NETWORK FOR HYPERSPECTRAL IMAGE CLASSIFICATION
XXIV ISPRS CONGRESS: IMAGING TODAY, FORESEEING TOMORROW, COMMISSION III(2022)
摘要
In recent years, deep neural networks (DNN) are commonly adopted for hyperspectral image (HSI) classification. As the most representative supervised DNN model, convolutional neural networks (CNNs) have outperformed most algorithms. But the main problem of CNN-based methods lies in the over-smoothing phenomenon. Meanwhile, mainstream methods usually require a large number of samples and a large amount of computation. A multi-task learning spectral-spatial multiscale residual network (SSMRN) is proposed to learn features of objects effectively. In the implementation of the SSMRN, a multiscale residual convolutional neural network (MRCNN) is proposed as spatial feature extractors and a band grouping-based bi-directional gated recurrent unit (Bi-GRU) is utilized as spectral feature extractors. To evaluate the effectiveness of the SSMRN, extensive experiments are conducted on public benchmark data sets. The proposed method can retain the detailed boundary of different objects better and yield a competitive performance compared with two state-of-the-art methods especially when the training samples are inadequate.
更多查看译文
关键词
Hyperspectral Image (HSI), Classification, Convolutional Neural Network (CNN), Recurrent Neural Network (RNN), Multi-Task Learning, Residual Networks
AI 理解论文
溯源树
样例
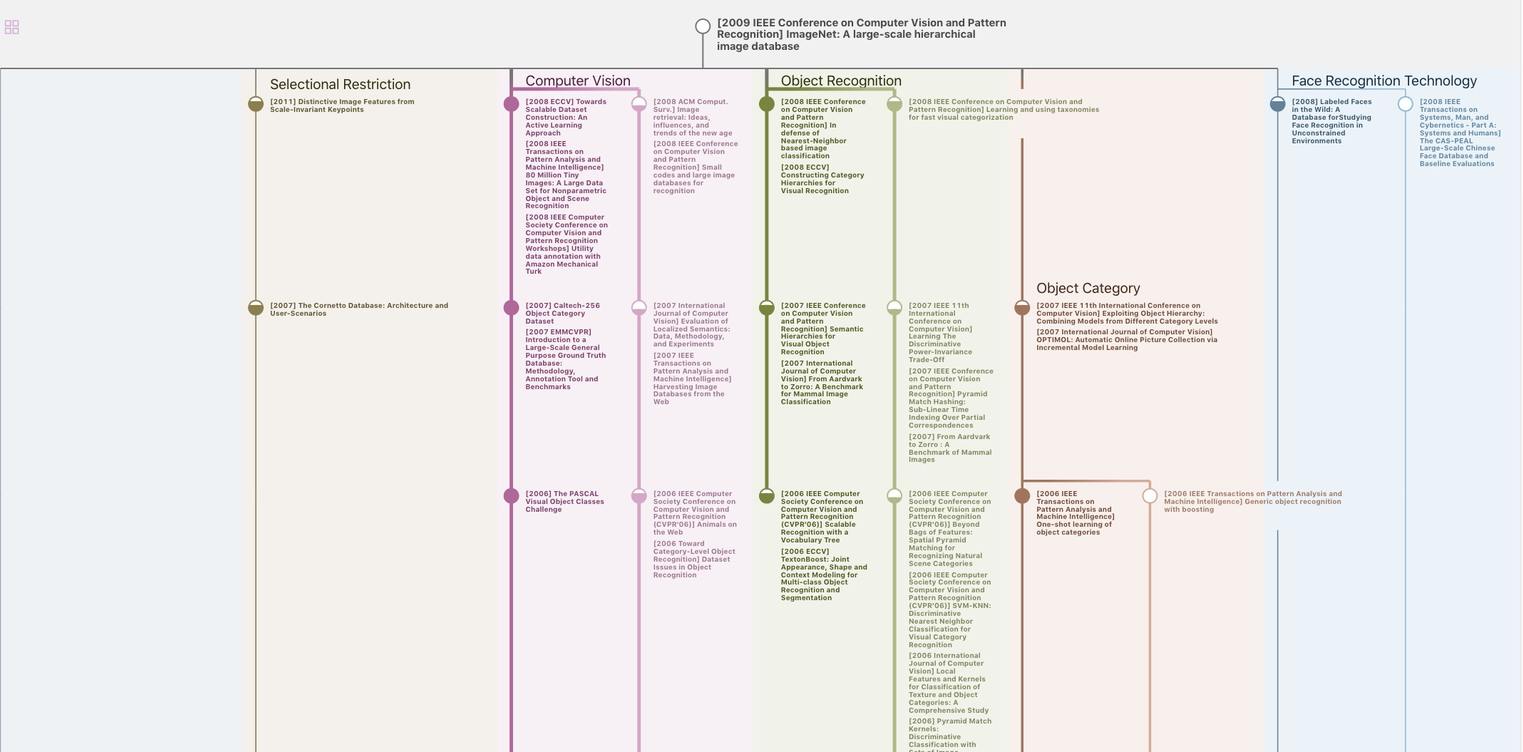
生成溯源树,研究论文发展脉络
Chat Paper
正在生成论文摘要