Comparing Expected Improvement and Kriging Believer for Expensive Bilevel Optimization
2021 IEEE CONGRESS ON EVOLUTIONARY COMPUTATION (CEC 2021)(2021)
摘要
Bilevel optimization refers to a specialized class of problems where one optimization task is nested as a constraint within another. Such problems emerge in a range of real-world scenarios involving hierarchical decision-making, and significant literature exists on classical and evolutionary approaches to solve them. However, computationally expensive bilevel optimization problems remain relatively less explored. Since each evaluation incurs a significant computational cost, one can only perform a limited number of function evaluations during the course of search. Surrogate-assisted strategies provide a promising way forward to deal with such classes of problems. Of particular interest to this study are the steady-state strategies which carefully pre-select a promising solution for true evaluation based on a surrogate model. The main aim of this paper is to compare two widely adopted steady-state infill strategies - Kriging believer (KB) and expected improvement (EI) - through systematic experiments within a nested optimization framework. Our experiments on a set of benchmark problems reveal some interesting and counter-intuitive observations. We discuss some of the underlying reasons and believe that the findings will inform further research on understanding and improving search strategies for expensive bilevel optimization.
更多查看译文
关键词
Expensive bilevel optimization, surrogate modeling, evolutionary algorithm
AI 理解论文
溯源树
样例
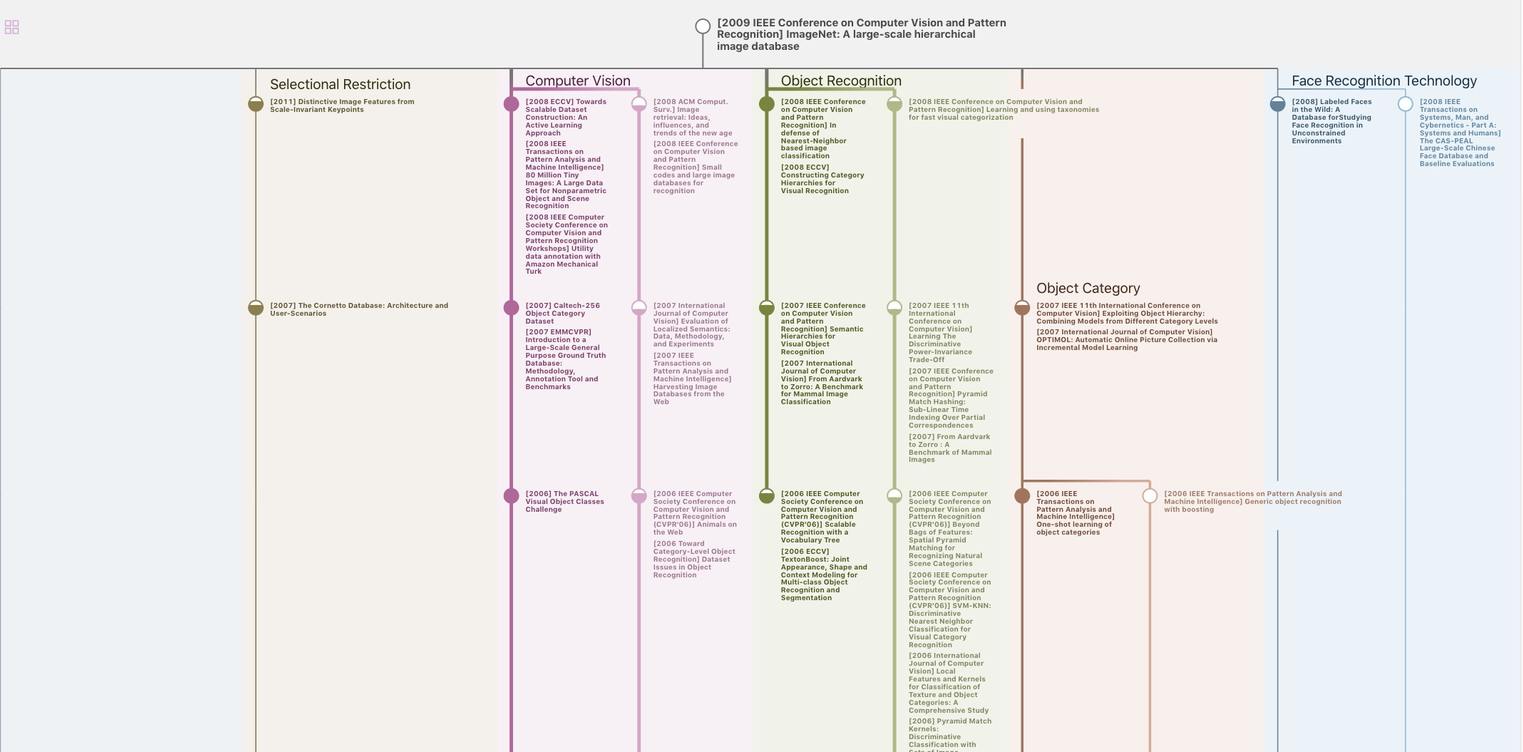
生成溯源树,研究论文发展脉络
Chat Paper
正在生成论文摘要