Grsnet: gated residual supervision network for pixel-wise building segmentation in remote sensing imagery
INTERNATIONAL JOURNAL OF REMOTE SENSING(2022)
摘要
The increasing development of imaging technology has made aerial image analysis one of the most widely used fields in image processing. Building extraction is the basic step in analysing urban structures, detecting construction violations, updating urban geographical divisions, and forecasting natural disasters. In this study, the aim is to automatically segment buildings in high-resolution satellite images using a new hybrid deep learning model, named Gated Residual Supervision Network (GRSNet). GRSNet extends the UNet framework by including three important components, i.e. attention gates (AG), residual units, and deep supervision part, with the main focus on transferring and reusing features. At first, the AG mechanism utilizes channel and spatial fine features to merge them effectively and deep supervision transfers feature details from the depths of the network. Then, residual units retrieve the information at different levels to train the model quickly. Finally, a fully connected classifier recovers features from the input image. GRSNet is evaluated on Massachusetts buildings public dataset and Inria aerial image labeling benchmark. The obtained results show the superiority of the proposed method compared to other deep learning-based state-of-the-art building segmentation methods with an intersection over union (IOU) of 89.86% and an F1-score of 94.53%.
更多查看译文
关键词
building extraction, deep learning, semantic segmentation, high-resolution imagery, remote sensing
AI 理解论文
溯源树
样例
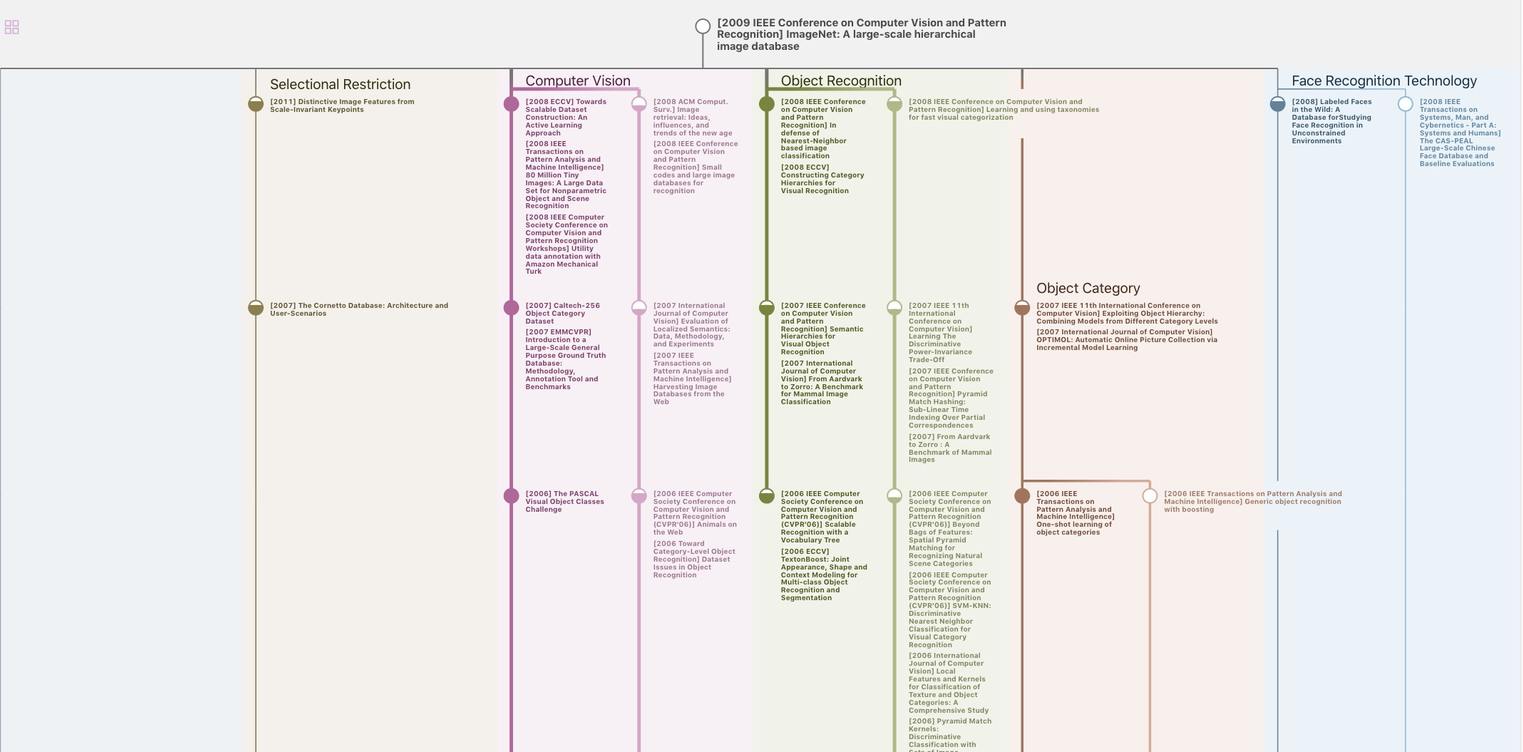
生成溯源树,研究论文发展脉络
Chat Paper
正在生成论文摘要