The multivariate-Tweedie: a self-weighting likelihood for age and length composition data arising from hierarchical sampling designs
ICES JOURNAL OF MARINE SCIENCE(2022)
摘要
Weighting data appropriately in stock assessment models is necessary to diagnose model mis-specification, estimate uncertainty, and when combining data sets. Age- and length-composition data are often fitted using a multinomial distribution and then reweighted iteratively, and the Dirichlet-multinomial ("DM") likelihood provides a model-based alternative that estimates an additional parameter and thereby "self-weights" data. However, the DM likelihood requires specifying an input sample size (n(input)), which is often unavailable and results are sensitive to n(input). We therefore introduce the multivariate-Tweedie (MVTW) as alternative with three benefits: (1) it can identify both overdispersion (downweighting) or underdispersion (upweighting) relative to the n(input); (2) proportional changes in n(input) are exactly offset by parameters; and (3) it arises naturally when expanding data arising from a hierarchical sampling design. We use an age-structured simulation to show that the MVTW (1) can be more precise than the DM in estimating data weights, and (2) can appropriately upweight data when needed. We then use a real-world state-space assessment to show that the MVTW can easily be adapted to other software. We recommend that stock assessments explore the sensitivity to specifying DM, MVTW, and logistic-normal likelihoods, particularly when the DM estimates an effective sample size approaching n(input).
更多查看译文
关键词
data weighting, Dirichlet-multinomial, hierarchical sampling design, stock assessment, Tweedie, WHAM
AI 理解论文
溯源树
样例
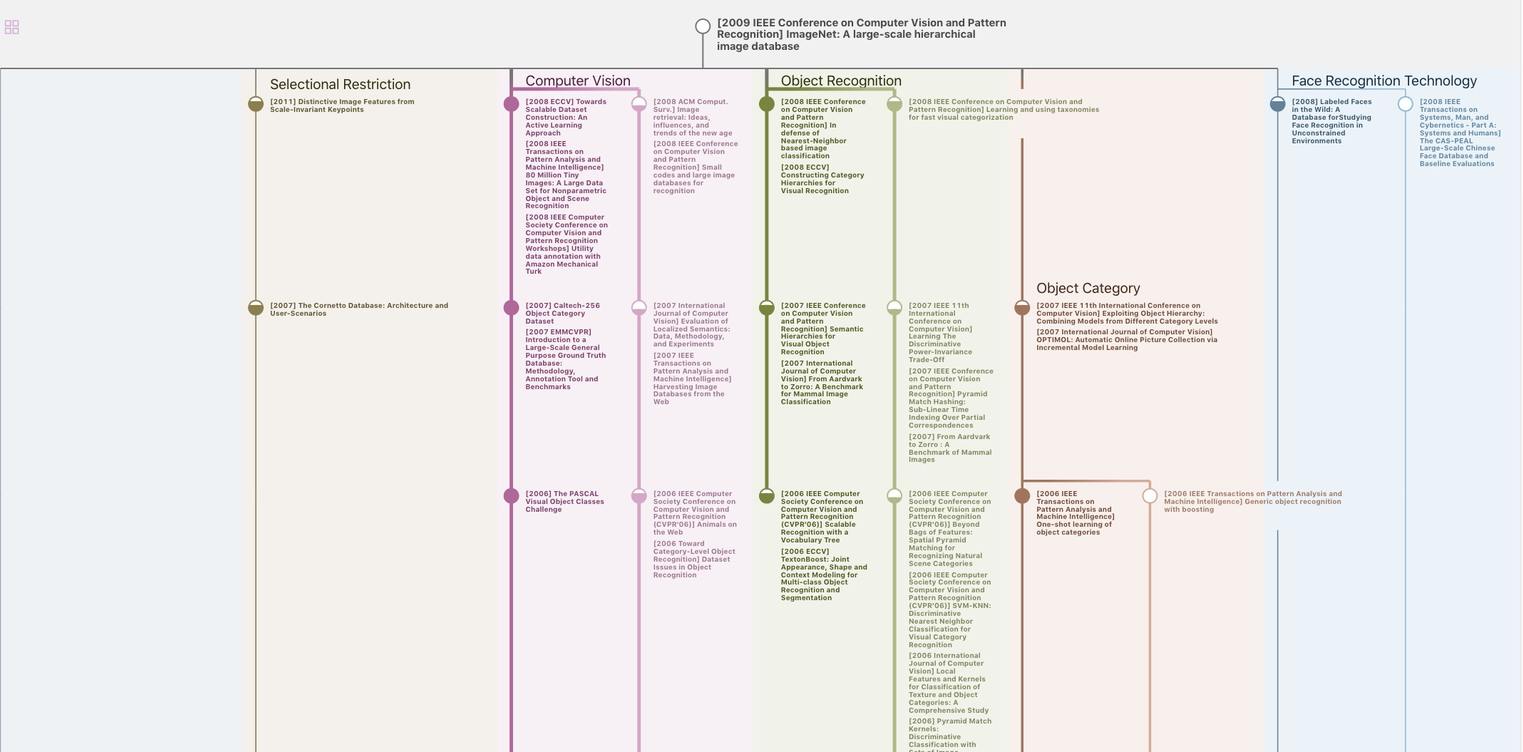
生成溯源树,研究论文发展脉络
Chat Paper
正在生成论文摘要