Statistical, machine learning and deep learning forecasting methods: Comparisons and ways forward
JOURNAL OF THE OPERATIONAL RESEARCH SOCIETY(2023)
摘要
The purpose of this paper is to test empirically the value currently added by Deep Learning (DL) approaches in time series forecasting by comparing the accuracy of some state-of-the-art DL methods with that of popular Machine Learning (ML) and statistical ones. The paper consists of three main parts. The first part summarizes the results of a past study that compared statistical with ML methods using a subset of the M3 data, extending however its results to include DL models, developed using the GluonTS toolkit. The second part widens the study by considering all M3 series and comparing the results obtained with that of other studies that have used the same data for evaluating new forecasting methods. We find that combinations of DL models perform better than most standard models, both statistical and ML, especially for the case of monthly series and long-term forecasts. However, these improvements come at the cost of significantly increased computational time. Finally, the third part describes the advantages and drawbacks of DL methods, discussing the implications of our findings to the practice of forecasting. We conclude the paper by discussing how the field of forecasting has evolved over time and proposing some directions for future research.
更多查看译文
关键词
Forecasting, artificial intelligence, neural networks, performance measurement, time series
AI 理解论文
溯源树
样例
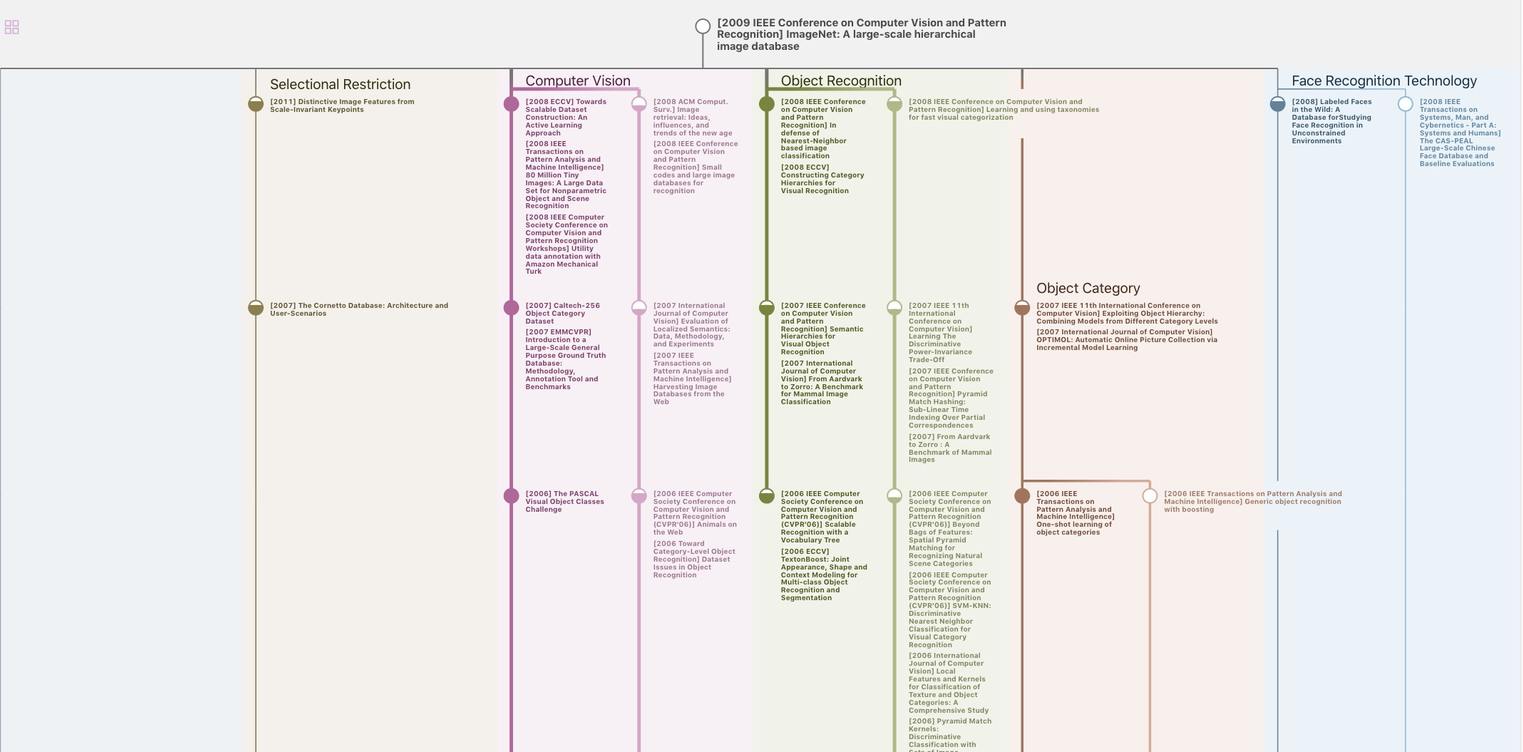
生成溯源树,研究论文发展脉络
Chat Paper
正在生成论文摘要