The Large-Scale Investigation and Analysis of Lophodermium piceae in Subalpine Areas Based on Satellite Multi-Spectral Remote Sensing
DIVERSITY-BASEL(2022)
摘要
Picea asperata, a common tree species in the subalpine areas of Li County, Sichuan Province, China, is susceptible to Lophodermium piceae. Remote sensing has the advantages of large-scale, fast information acquisition, and low cost, which can overcome the shortcomings of ground survey. Hence, we used Landsat 8 satellite multi-spectral images and forest resource distribution data to investigate and analyze this forest disease at a large scale. Firstly, we extracted the spatial distribution information of Picea asperata and chose a temporal sequence indicator to establish a regression model and obtained a significantly negative correlation between the damage degree of plants and the change rate of normalized difference vegetation index (NDVI). Accordingly, the investigation results of the disease have good consistency with the ground survey data in spatial distribution and damage degree. On this basis, a temporal regression analysis was performed by combining the remote sensing investigation results with climate variables, and canonical correspondence analysis (CCA) was utilized in the spatial comprehensive analysis of Lophodermium piceae with terrain, soil and forest stand factors. Conclusively, this study effectively coped with the difficulties in full investigation and analysis of Lophodermium piceae in ecologically fragile subalpine areas of Western Sichuan. It is of important reference value in the early warning and monitoring of this disease, and also provides objective and reliable information support for ecological restoration and management planning in the Wenchuan earthquake-stricken areas.
更多查看译文
关键词
Lophodermium piceae, subalpine area, satellite multi-spectral images, large-scale investigation, spatio-temporal analysis
AI 理解论文
溯源树
样例
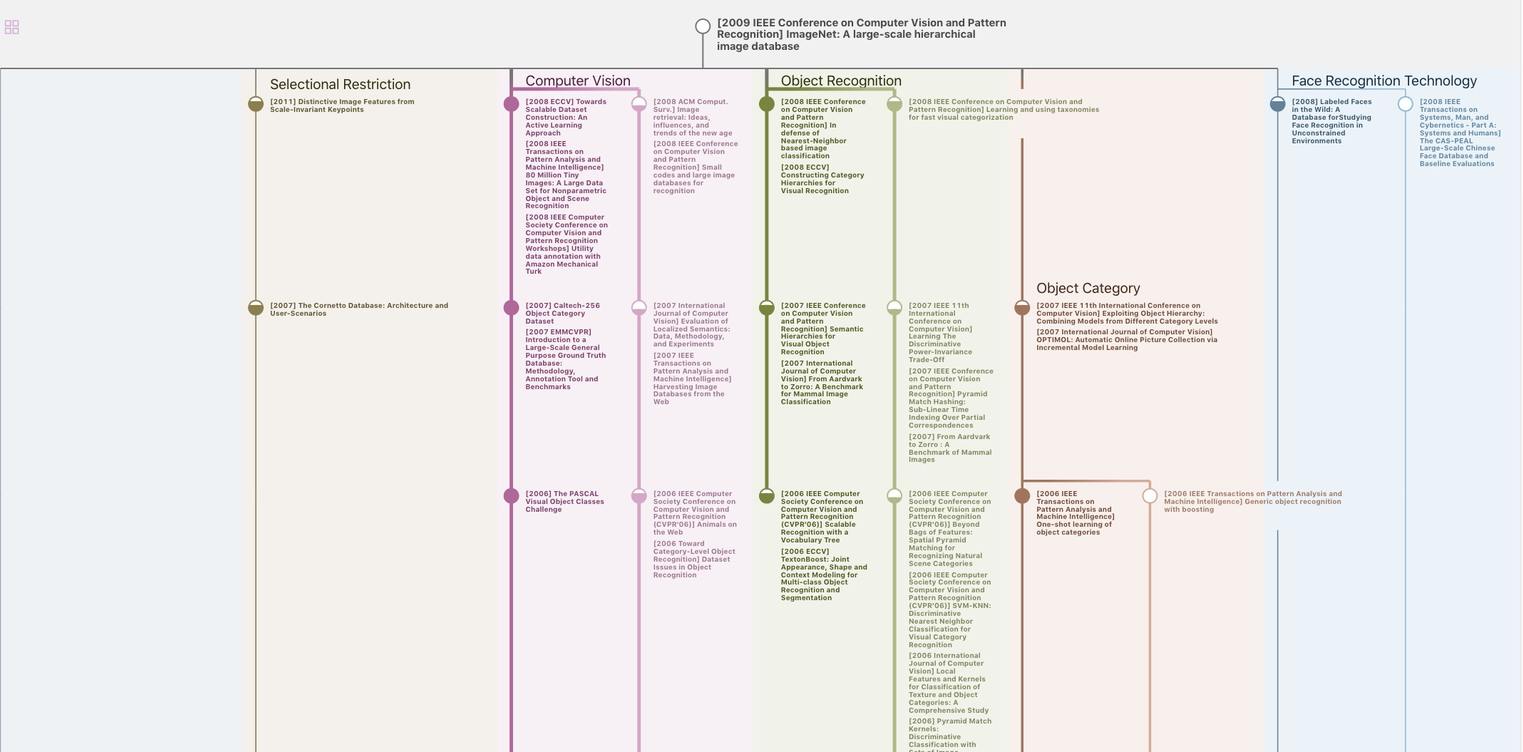
生成溯源树,研究论文发展脉络
Chat Paper
正在生成论文摘要