Evaluating automated benthic fish detection under variable conditions
ICES JOURNAL OF MARINE SCIENCE(2022)
摘要
Advances in imaging systems have facilitated the collection of high-volume imagery datasets in fisheries science. To alleviate the costs of sorting these datasets, automated image processing techniques are used. In this study, we investigate a machine learning-enabled imaging technique for automating individual fish detection from stereo image pairs of orange roughy (Hoplostethus atlanticus). We performed a set of object detection experiments to investigate how well a Single Shot Multi-Box Detector (SSD) model worked under dynamic real-world conditions when trained over a small number of epochs. We tested model generalization between the port and starboard side cameras; at variable fish densities; different benthic substrates; and at different altitudes above the seafloor. We show that (1) changes in perspective between starboard and port images are not enough to break the object detector, (2) the object detector begins to have trouble differentiating individuals at high fish densities (>20 fish per image), (3) substrate type does not affect model performance, and (4) altitude is not a major factor contributing to model error. Ideally, this type of real-world dataset exploration should be performed prior to committing the resources to train the final object detector over several hundred epochs.
更多查看译文
关键词
automation, benthic imagery, density, machine learning, object detection, orange roughy, substrate
AI 理解论文
溯源树
样例
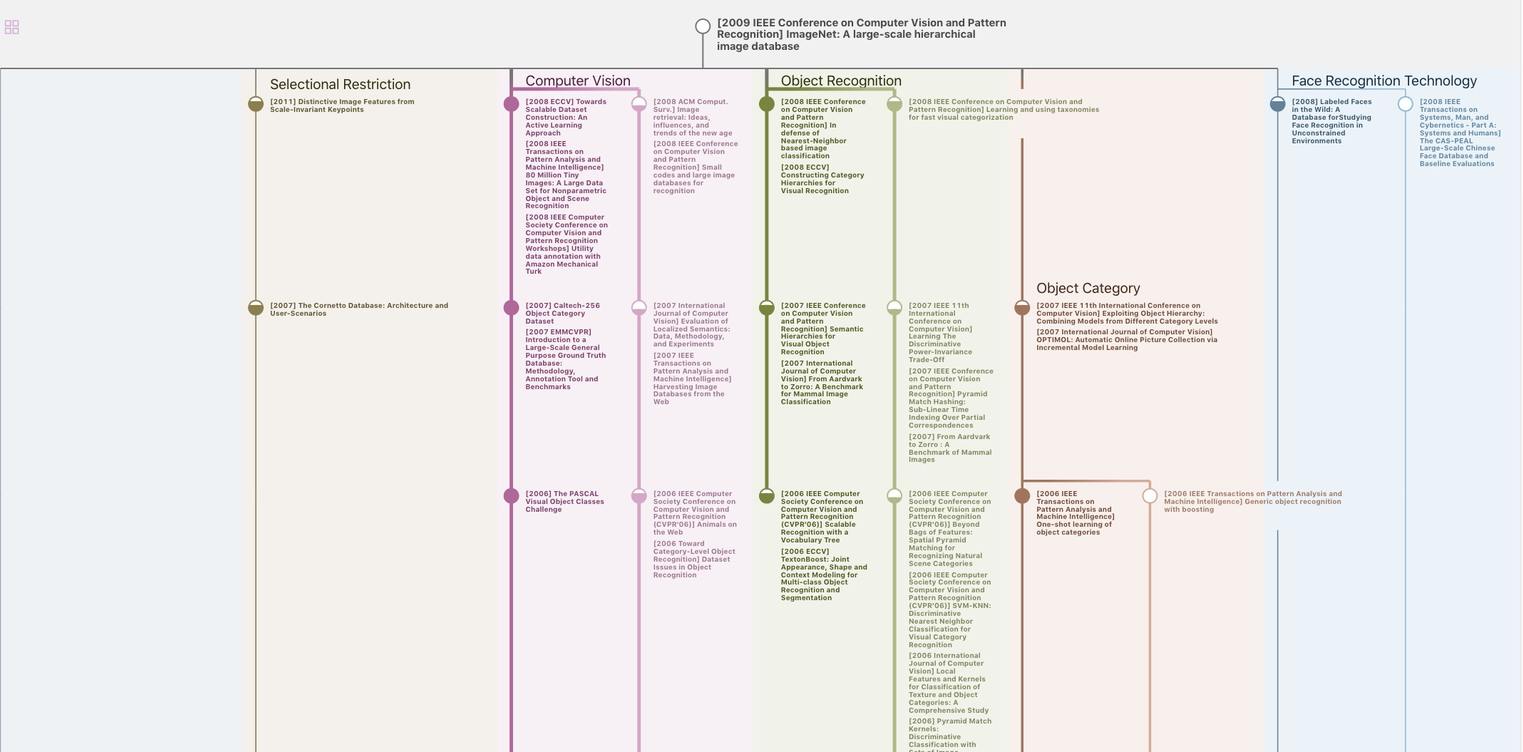
生成溯源树,研究论文发展脉络
Chat Paper
正在生成论文摘要