Data Augmentation-based Prognostics for Predictive Maintenance of Industrial System
CIRP ANNALS-MANUFACTURING TECHNOLOGY(2022)
摘要
Anticipating system failures using predictive strategies based on efficient prognostics has become an important topic in manufacturing where maintenance plays a crucial role. As such, promising prognostics approaches use data-driven machine learning techniques, though the initial data set for learning is often small as failure occurrences are rare. Therefore, this study investigates data augmentation methods for improving prognostics by increasing data set size using samples generated by altering existing ones. First, a method is proposed for quantifying the gain from additional data. Thereafter, augmentation methods are assessed through a benchmark. Finally, contributions are illustrated in a steel industry case-study. (C) 2022 CIRP. Published by Elsevier Ltd. All rights reserved.
更多查看译文
关键词
Maintenance, Machine Learning, Data Augmentation
AI 理解论文
溯源树
样例
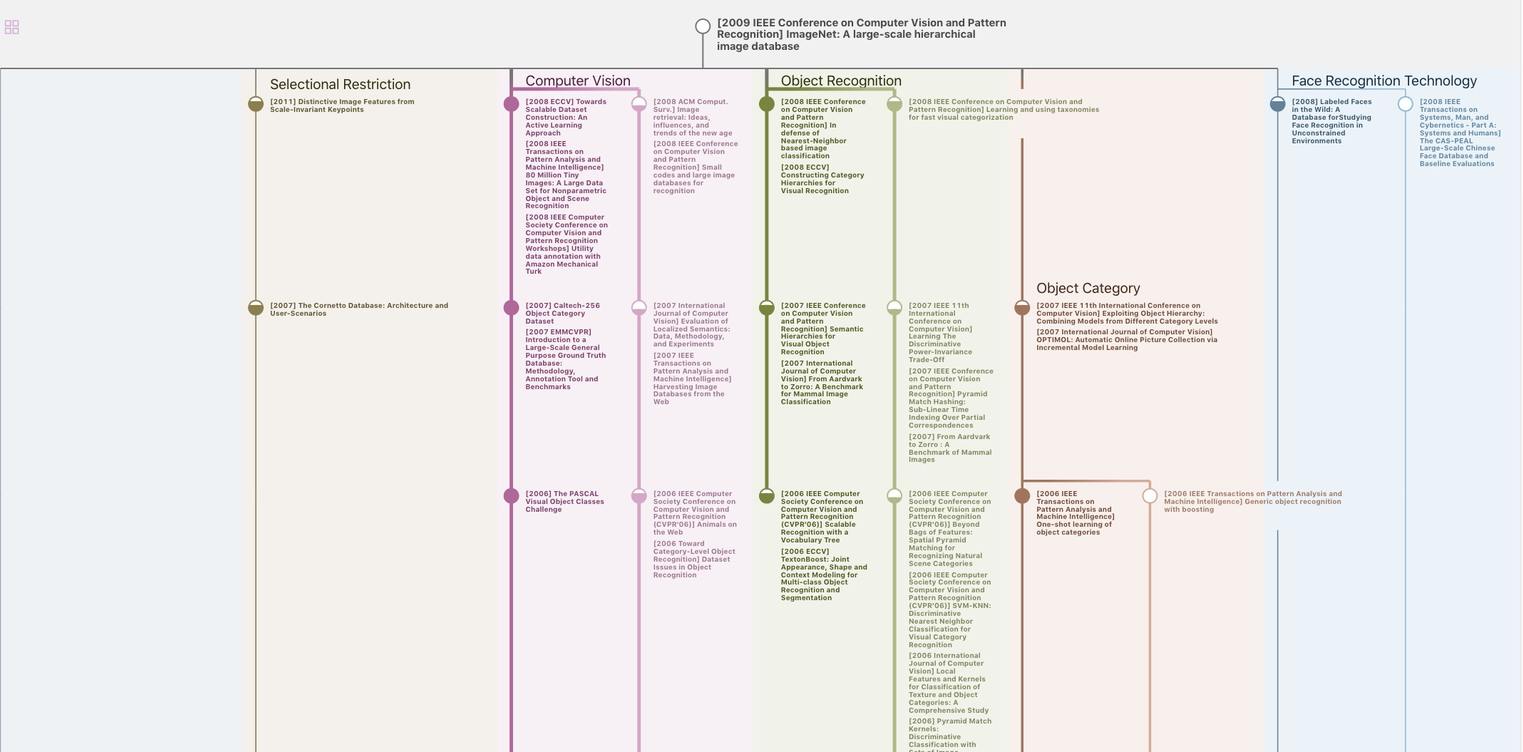
生成溯源树,研究论文发展脉络
Chat Paper
正在生成论文摘要