Refined composite multiscale fuzzy entropy based fault diagnosis of diesel engine
JOURNAL OF LOW FREQUENCY NOISE VIBRATION AND ACTIVE CONTROL(2023)
摘要
Due to complicated transfer paths and strong background noise interference, the fault pattern information deeply hides in common features of the vibration signal at the engine surface. In this study, the refined composite multiscale fuzzy entropy (RCMFE) used to measure the irregularity and self-similarity of time series is proposed to quantify the feature of various fault patterns. Followed by RCMFE, the features dug out are recognized by a parameter-adaptive support vector machine based on the firefly algorithm (FASVM). After putting forward the diagnosing schematics, the RCMFE-FASVM is applied to a fault diagnosis case of a diesel engine on a test rig. A comparative analysis of the four methods to extract features and the four methods to recognize fault patterns are conducted. Results indicate the proposed method has superior recognition performance and can effectively identify the working states of the diesel engine, contrasting the existing methods. Under the small samples and features task of identifying the working states of a diesel engine, the recognition rate of the proposed method with more stability can reach 98.2%, which is larger than other methods. Given the superior performance of the proposed method, the number of input features and training samples should vary from 8 to 20 and from 35 to 50.
更多查看译文
关键词
Diesel engine, fault diagnosis, vibration signal, refined composite multiscale fuzzy entropy, firefly algorithm, parameter-adaptive support vector machine
AI 理解论文
溯源树
样例
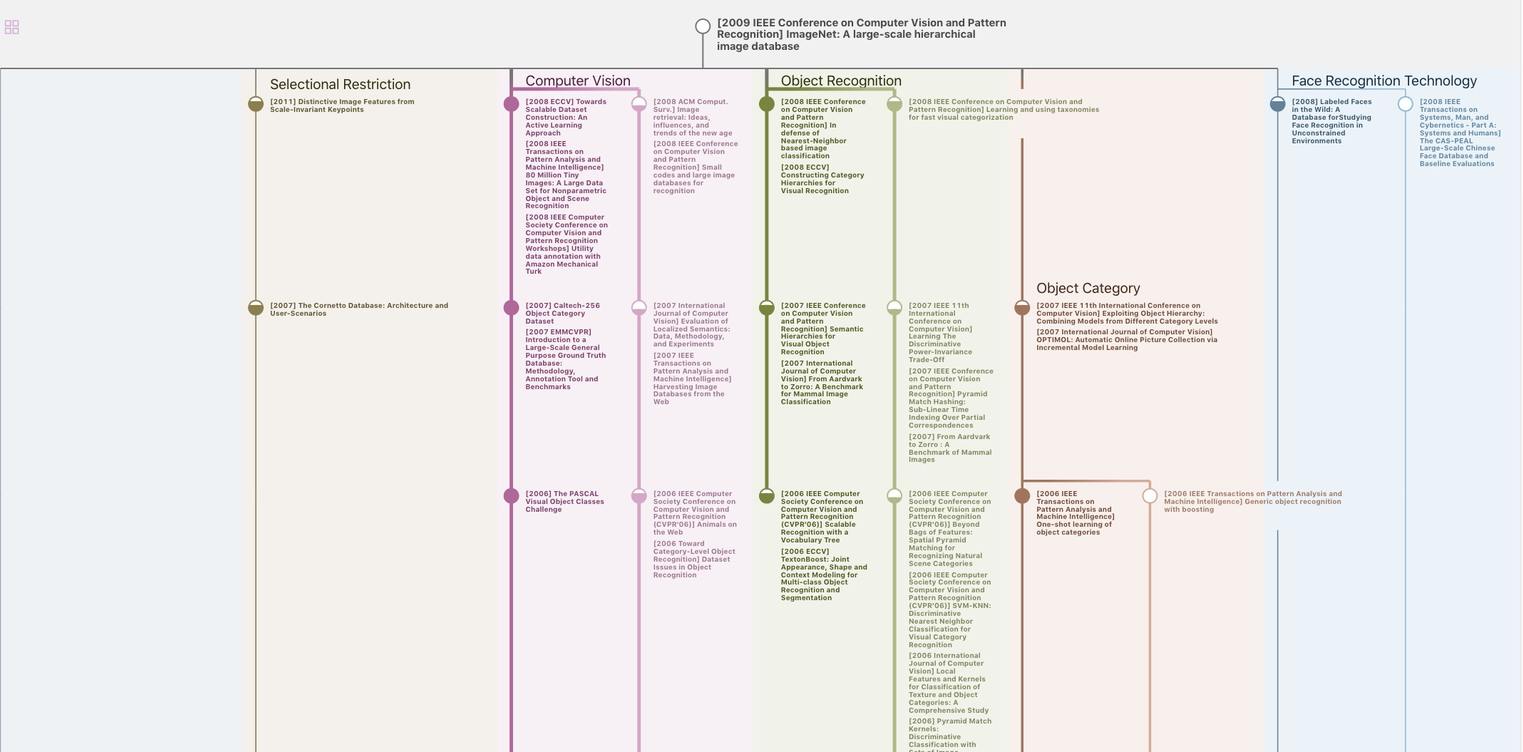
生成溯源树,研究论文发展脉络
Chat Paper
正在生成论文摘要