DEFORESTATION DETECTION WITH WEAK SUPERVISED CONVOLUTIONAL NEURAL NETWORKS IN TROPICAL BIOMES
XXIV ISPRS CONGRESS: IMAGING TODAY, FORESEEING TOMORROW, COMMISSION III(2022)
摘要
Deep learning methods are known to demand large amounts of labeled samples for training. For remote sensing applications such as change detection, coping with that demand is expensive and time-consuming. This work aims at investigating a noisy-label-based weak supervised method in the context of a deforestation mapping application, characterized by a high class imbalance between the classes of interest, i.e., deforestation and no-deforestation. The study sites correspond to different regions in the Amazon and Brazilian Cerrado biomes. To mitigate the lack of ground-truth labeled training samples, we devised an unsupervised pseudo-labeling scheme based on the Change Vector Analysis technique. The experimental results indicate that the proposed approach can improve the accuracy of deforestation detection applications.
更多查看译文
关键词
Change detection, deep learning, domain adaptation, deforestation, weak supervision
AI 理解论文
溯源树
样例
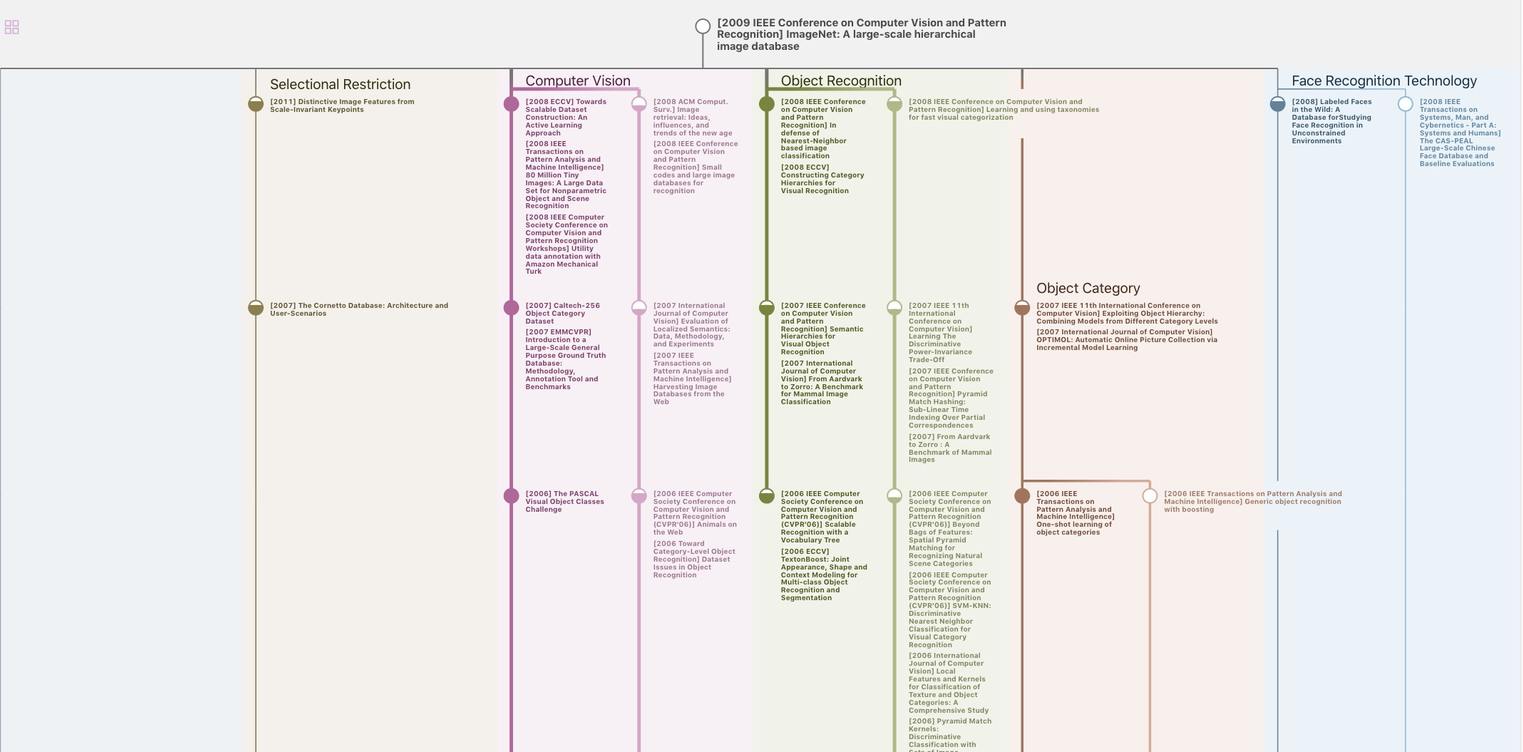
生成溯源树,研究论文发展脉络
Chat Paper
正在生成论文摘要