A new randomized primal-dual algorithm for convex optimization with fast last iterate convergence rates
OPTIMIZATION METHODS & SOFTWARE(2023)
摘要
We develop a novel unified randomized block-coordinate primal-dual algorithm to solve a class of nonsmooth constrained convex optimization problems, which covers different existing variants and model settings from the literature. We prove that our algorithm achieves O(n/k) and O(n(2)/k(2)) convergence rates in two cases: merely convexity and strong convexity, respectively, where k is the iteration counter and n is the number of block-coordinates. These rates are known to be optimal (up to a constant factor) when n = 1. Our convergence rates are obtained through three criteria: primal objective residual and primal feasibility violation, dual objective residual, and primal-dual expected gap. Moreover, our rates for the primal problem are on the last-iterate sequence. Our dual convergence guarantee requires additionally a Lipschitz continuity assumption. We specify our algorithm to handle two important special cases, where our rates are still applied. Finally, we verify our algorithm on two well-studied numerical examples and compare it with two existing methods. Our results show that the proposed method has encouraging performance on different experiments.
更多查看译文
关键词
Randomized block coordinate algorithm, primal-dual method, constrained convex optimization, fast last-iterate convergence rate
AI 理解论文
溯源树
样例
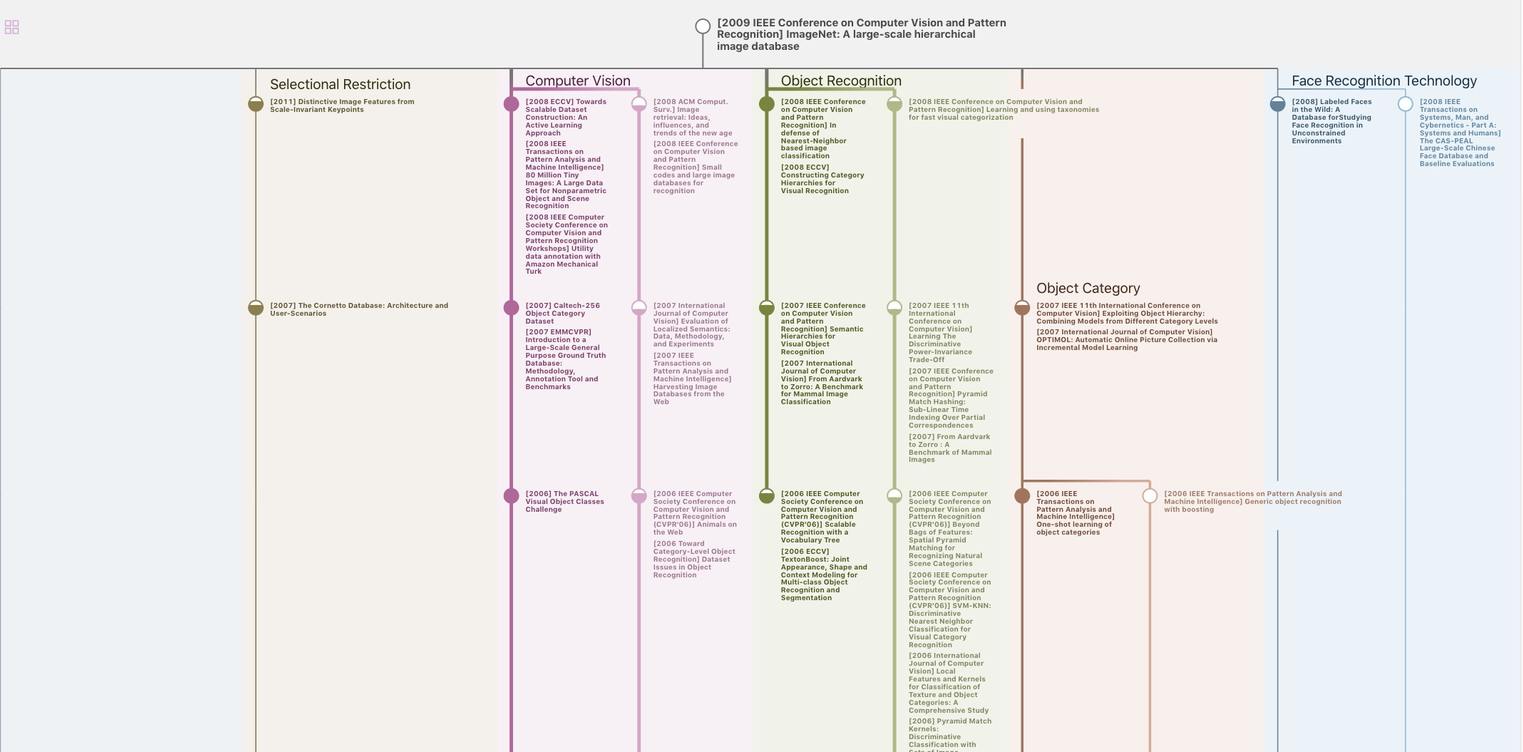
生成溯源树,研究论文发展脉络
Chat Paper
正在生成论文摘要