Transfer Learning of Motor Difficulty Classification in Physical Human-Robot Interaction Using Electromyography
JOURNAL OF COMPUTING AND INFORMATION SCIENCE IN ENGINEERING(2022)
摘要
Efficient human-robot collaboration during physical interaction requires estimating the human state for optimal role allocation and load sharing. Machine learning (ML) methods are gaining popularity for estimating the interaction parameters from physiological signals. However, due to individual differences, the ML models might not generalize well to new subjects. In this study, we present a convolution neural network (CNN) model to predict motor control difficulty using surface electromyography (sEMG) from human upper limb during physical human-robot interaction (pHRI) task and present a transfer learning approach to transfer a learned model to new subjects. Twenty-six individuals participated in a pHRI experiment where a subject guides the robot's end-effector with different levels of motor control difficulty. The motor control difficulty is varied by changing the damping parameter of the robot from low to high and constraining the motion to gross and fine movements. A CNN network with raw sEMG as input is used to classify the motor control difficulty. The CNN's transfer learning approach is compared against Riemann geometry-based Procrustes analysis (RPA). With very few labeled samples from new subjects, we demonstrate that the CNN-based transfer learning approach (avg. 69.77%) outperforms the RPA transfer learning (avg. 59.20%). Moreover, we observe that the subject's skill level in the pre-trained model has no significant effect on the transfer learning performance of the new users.
更多查看译文
关键词
artificial intelligence, human computer interfaces, interactions, machine learning for engineering applications
AI 理解论文
溯源树
样例
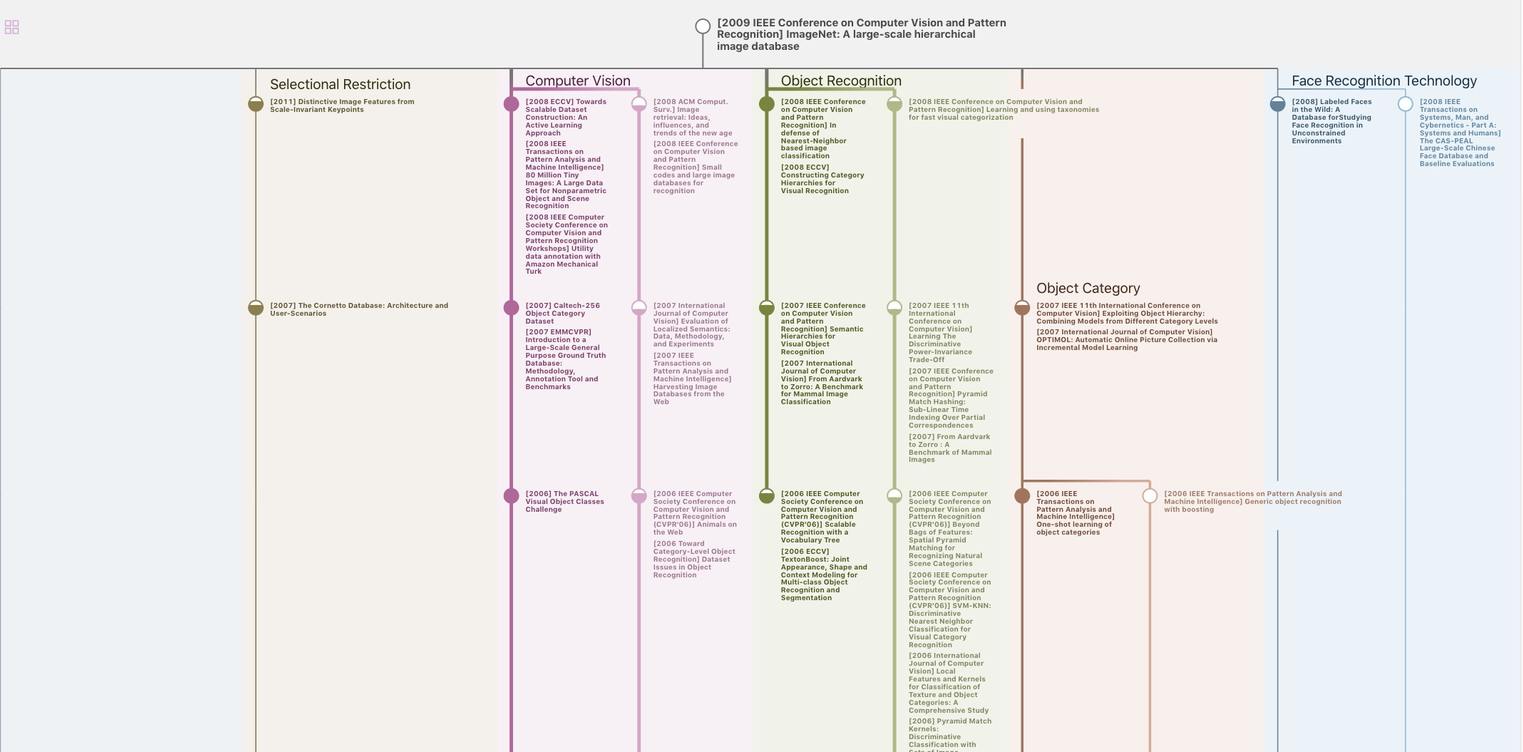
生成溯源树,研究论文发展脉络
Chat Paper
正在生成论文摘要