Load Forecasting Combining Chaos Theory and Long-Short Term Memory in Absence of Key Information
2021 IEEE IAS INDUSTRIAL AND COMMERCIAL POWER SYSTEM ASIA (IEEE I&CPS ASIA 2021)(2021)
摘要
Power load forecasting used to rely on some key data such as weather information and regional information. However, it is usually difficult to obtain accurate data of all influencing factors. For this reason, we adopted phase space reconstruction of chaos theory to process the historical load series to avoid collecting some key data. To forecast ahead of time and solve the divergence of forecasting error caused by forecasting ahead of time, this paper proposes a rolling load forecasting method and apply long-short term memory (LSTM) to solve them. The rolling forecasting method is to predict a forecast length in advance, and the test data of LSTM is obtained by shifting a forecast length of training data. This method can not only predict ahead, but also predict the longer-term data through keeping shifting. The proposed methodology is implemented on the PJM power grid dataset in the United States. Compared with some other methods, the results show that the proposed rolling load forecasting model is of more accurate prediction.
更多查看译文
关键词
chaos theory, phase space reconstruction, LSTM, permutation entropy
AI 理解论文
溯源树
样例
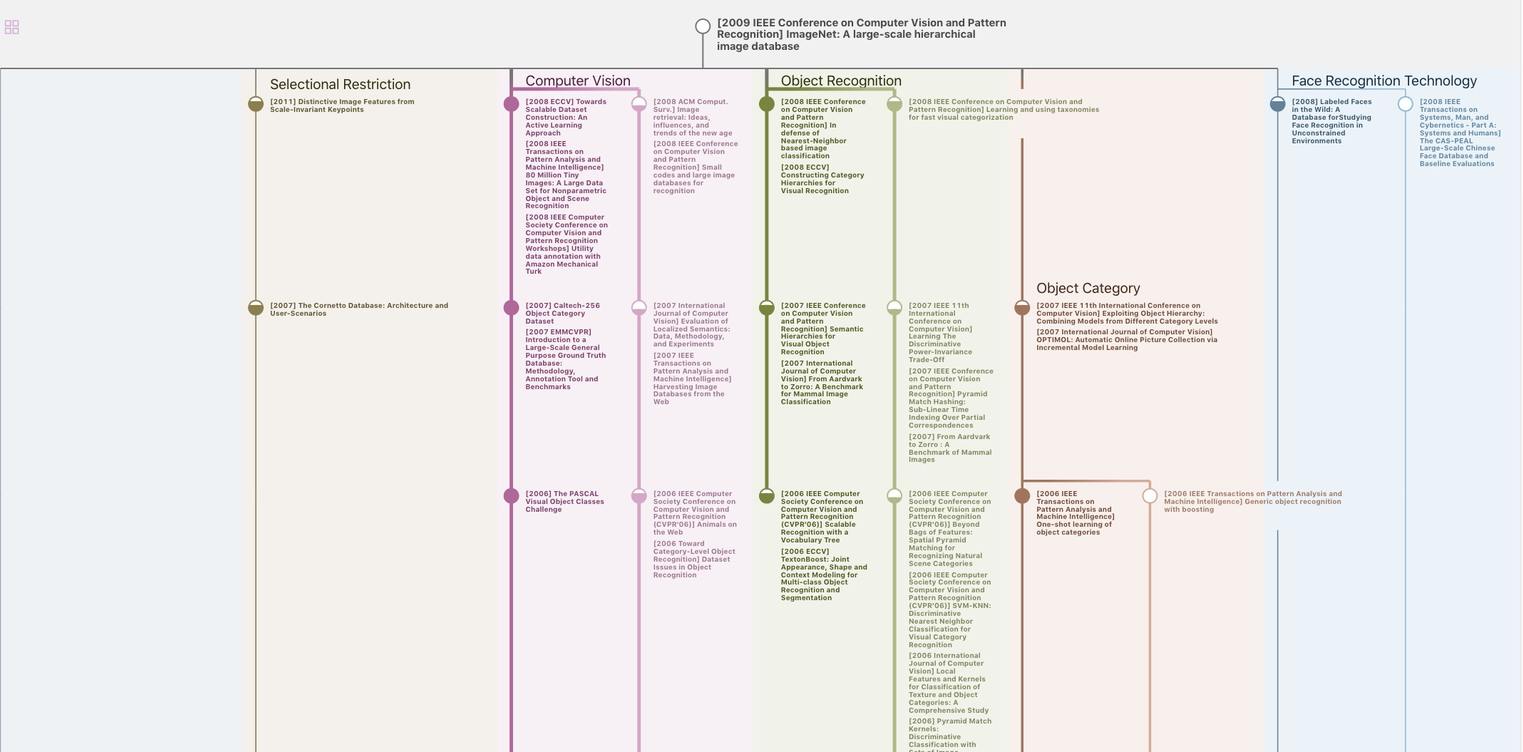
生成溯源树,研究论文发展脉络
Chat Paper
正在生成论文摘要