Automatic Classification of Crack Severity from Cross-Section Image of Timber Using Simple Convolutional Neural Network
APPLIED SCIENCES-BASEL(2022)
摘要
Featured Application Automatic evaluation for timber in construction. Currently, humans conduct this evaluation. It is tedious and time-consuming. CNN is suitable because it can incorporate subjective human evaluations and reduce time and human effort. Cedar and other timbers used for construction generally undergo a high-temperature drying process after being harvested to maintain their quality. However, internal cracks occur during this process. This is an issue because it deteriorates the structural performance, such as buckling strength and joint durability of the timber. Since preventing these internal cracks is difficult, their severity must be examined manually. Currently, the length, thickness, and area of the cracks on a cross-sectional surface of square timber are measured using calipers. However, this process is time-consuming and labor-intensive. Therefore, we employed a convolutional neural network (CNN), widely used in artificial intelligence applications, to automatically evaluate the severity of cracks from cross-sectional images of timber. A novel CNN was constructed and experimentally evaluated in this study. The average classification accuracy was 85.67%.
更多查看译文
关键词
timber, crack, simple convolutional neural network, human fuzziness, machine learning
AI 理解论文
溯源树
样例
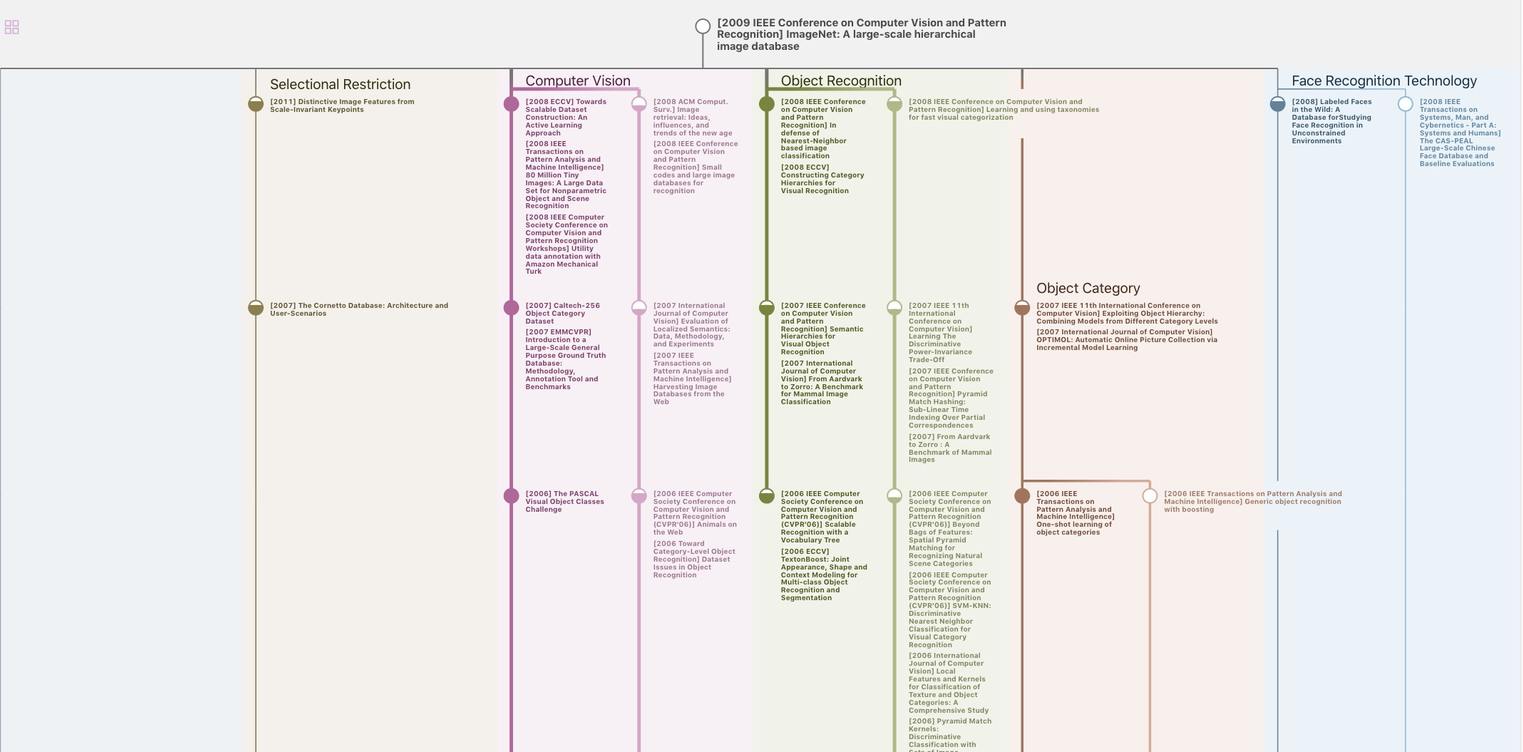
生成溯源树,研究论文发展脉络
Chat Paper
正在生成论文摘要