Optimal Maneuvering for Autonomous Vehicle Self-Localization
ENTROPY(2022)
摘要
We consider the problem of optimal maneuvering, where an autonomous vehicle, an unmanned aerial vehicle (UAV) for example, must maneuver to maximize or minimize an objective function. We consider a vehicle navigating in a Global Navigation Satellite System (GNSS)-denied environment that self-localizes in two dimensions using angle-of-arrival (AOA) measurements from stationary beacons at known locations. The objective of the vehicle is to travel along the path that minimizes its position and heading estimation error. This article presents an informative path planning (IPP) algorithm that (i) uses the determinant of the self-localization estimation error covariance matrix of an unscented Kalman filter as the objective function; (ii) applies an l-step look-ahead (LSLA) algorithm to determine the optimal heading for a constant-speed vehicle. The novel algorithm takes into account the kinematic constraints of the vehicle and the AOA means of measurement. We evaluate the performance of the algorithm in five scenarios involving stationary and mobile beacons and we find the estimation error approaches the lower bound for the estimator. The simulations show the vehicle maneuvers to locations that allow for minimum estimation uncertainty, even when beacon placement is not conducive to accurate estimation.
更多查看译文
关键词
autonomous systems, optimal maneuvering, informative path planning, angle-of-arrival localization, information and sensor fusion, optimization and planning
AI 理解论文
溯源树
样例
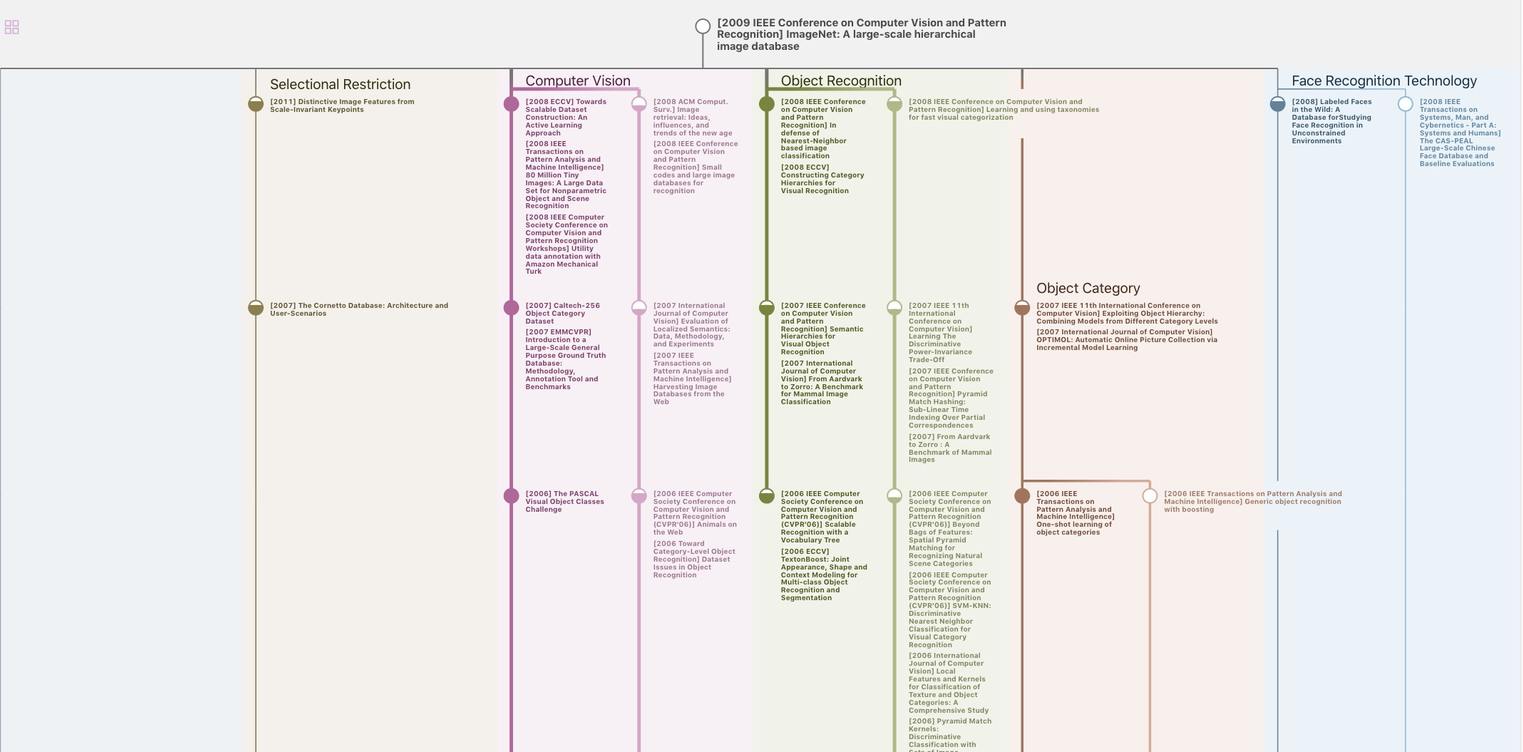
生成溯源树,研究论文发展脉络
Chat Paper
正在生成论文摘要